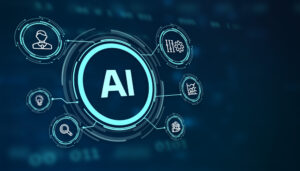
AI agents offer a way to move beyond batch-processing systems, which are often used to get structured data into data pipelines.
They’re calling agentic AI – the use of intelligent, autonomous agents – the next wave of artificial intelligence following the generative AI boom. However, there is a blind spot in the AI landscape that may hold back progress with agents – a lack of attention on structured operational data that needs to be fed real-time to AI environments. Many are still locked away in batch-processing systems, requiring complex or over-engineered infrastructures to leverage.
That’s the word from Ben Lorica, former chief data scientist at O’Reilly Media, In a recent post at Gradient Flow. “While the buzz surrounds unstructured data formats like sales calls, PDFs, and videos, we mustn’t forget that structured operational data remains essential to enterprise intelligence,” he explained. “This might seem like a step backward from the cutting-edge world of multimodal AI, but I believe it represents one of the most pressing challenges facing teams building agents and other AI applications.”
Lorica called this disconnect “more than a technical hiccup; it’s a strategic blind spot.” that results in AI applications “hamstrung by an operational data gap, frustratingly disconnected from business-critical data stored in siloed systems like CRMs and transactional databases.”
The effort required to “integrate AI with real-time data sources often produces brittle solutions that demand significant engineering resources,” he continued.
Real-time access to structured data is essential to enabling AI chatbots to “instantly access live order statuses and customer histories, slashing customer wait times,” or real-time analytics tools to “integrate live data across sales and supply chains.”
Structured data is also essential to enabling autonomous AI agents to “make informed decisions based on up-to-the-minute operational context.”
See also: CIO Insights: The Strategic Importance of RAG and Open Source in GenAI
RAG and AI Agents
While many sites with legacy data environments are looking to Retrieval-Augmented Generation (RAG)-based environments to integrate with large language models, they falter “with the complex relationships inherent in operational databases,” he cautioned. “While RAG excels at processing documents and general knowledge, it often reduces rich, structured data to flat embeddings, losing the relationships that make relational databases powerful.”
There is emerging technology to better reach underlying essential data to deliver to AI agents, though this is a market very much in the early formation stages. Lorica pointed to startup platforms such as Snow Leopard AI (not to be confused with Apple’s Mac Snow Leopard operating system), which he described as a platform that “introduces an intelligent layer that communicates directly with existing systems using their native protocols.”
Lorica also discussed tools such as BoundaryML (BAML), an open-source language that helps extract structured data from LLMs, and Anthropic’s Model Context Protocol (MCP), an open standard for connecting AI assistants to external data sources and systems where data lives, including content repositories, business tools, and development environments.
In addition, tools such as Mosaic AI Agent Framework, available through Databricks, which enables developers to create AI agent tools to query structured data sources such as SQL tables. and C3 Generative AI’s Intelligent Query Agents.
AI agents have recently burst upon the scene – and they will require large amounts of data still existing in structured formats.
Traditional ETL pipelines and batch processes ensure that any data they receive is stale, unsuitable for dynamic AI applications.
Addressing this chasm is imperative and would unlock many applications: For businesses building AI applications, bridging this gap isn’t just about technical elegance—it’s about enabling the kind of responsive, context-aware AI systems that customers increasingly expect.
Data Integration Toolbox: Trading Elegance for Expedience
The tools available to AI teams aiming to bridge the operational data gap range from sophisticated to makeshift.
Traditional methods offer limited improvement. ETL pipelines and data warehouses remain primary means to connect operational systems with AI applications. Yet their batch-processing nature and inherent latency are increasingly inadequate in an era that demands real-time responsiveness. Function calling and AI agents present a more modern approach, providing purpose-built integrations for specific use cases. However, these solutions can be costly: they deliver impressive results for narrow applications but require significant engineering resources and lead to maintenance challenges that grow with each new integration.
The limitations of current solutions highlight a broader issue: balancing scalability and fidelity. Custom pipelines and ad hoc connectors can offer precise, real-time access to operational data but are difficult to scale across multiple data sources. Fine-tuned LLMs provide another option but come with their own trade-offs: high computational costs, the need for fine-tuning data, and the difficulty of keeping models in sync with rapidly changing operational data. In my experience, many teams end up combining these approaches, resulting in complex architectures that function but feel more like temporary fixes than permanent solutions.
Snow Leopard: Bridging the AI-Operational Data Divide
Snow Leopard offers a practical solution to a problem that has hindered many AI implementations: accessing live operational data without overhauling existing infrastructures. Rather than requiring enterprises to redesign their data architectures, Snow Leopard introduces an intelligent layer that communicates directly with existing systems using their native protocols. This approach enables AI applications to access up-to-date data without the latency and complexity introduced by traditional ETL processes.
What sets Snow Leopard apart is its commitment to connecting AI systems with data where it already resides. By employing intelligent query routing and native integrations with systems ranging from SQL databases to REST APIs, it eliminates the need for custom connectors for each data source. This not only simplifies the integration process but also fundamentally changes how AI applications interact with live data, making real-time insights more accessible. Importantly, Snow Leopard is designed to work alongside existing ETL processes when batch processing or data warehousing remains appropriate, providing a complementary solution rather than a complete overhaul.
The platform also addresses critical concerns around governance and security. Operating within customer VPCs and incorporating built-in controls, Snow Leopard meets enterprise requirements for data privacy and compliance—issues that can derail AI initiatives if not properly managed. As an early-stage product, note that Snow Leopard has limited production deployments, with most implementations still in the proof-of-concept phase. Additionally, its focus on structured data means it offers limited support for unstructured data types like images or audio.
Despite these constraints, early adoption by fintech and SaaS companies suggests that Snow Leopard is addressing a significant need. By maintaining data fidelity and eliminating transformation steps, it tackles a major pain point in AI development. Perhaps most compelling is its scalability: the platform promises to support multiple use cases across various data sources without extensive customization. For teams burdened by the maintenance of custom data pipelines, this could represent a meaningful shift towards more efficient and responsive AI applications.
The Road Ahead: Connecting AI with Enterprise Reality
In a recent conversation with Snow Leopard’s CEO, Deepti Srivastava, she outlined the company’s plans to deepen the platform’s capabilities. High on the agenda is expanding their connector library to include integrations with a broader array of data sources, driven by customer demand. They plan to introduce advanced query features like cross-source joins and aggregations, enabling more complex data interactions without sacrificing performance. Recognizing the critical importance of governance, Snow Leopard is building robust frameworks for policy enforcement and compliance, ensuring that data flows securely and within regulatory bounds. And in a nod to the growing significance of unstructured data, they will eventually add capabilities to handle formats like text, images, and audio.
For teams building AI applications, these emerging tools represent essential infrastructure for creating AI that can understand and respond to business realities in real time. The future of AI agents lies not just in their reasoning capabilities but in their ability to seamlessly integrate with the systems where business happens. Looking ahead to 2025, as tools for accessing operational data like Snow Leopard mature, we can expect to see a dramatic evolution in agent AI capabilities. Teams building RAG applications will increasingly shift toward developing AI systems that can access and leverage the right operational data in real time, leading to more sophisticated and business-aware AI applications that can truly deliver on the promise of autonomous decision-making.