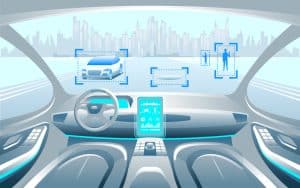
Synthetic data can help train AI/ML applications in edge cases where it is difficult or dangerous to capture data in real life.
It’s no surprise that collecting real-world data for training an artificial intelligence or machine learning (AI/ML) is time-consuming and costly. It’s also full of risk—sometimes literally, but more commonly in terms of how too little data or biased data could send an organization astray. But what if you could just fabricate new data, so called synthetic data, out of thin air?
That sounds improbable, but that’s exactly what Synthesis AI is planning to do with $17 million in series A fundraising from VC firms like 468 Capital, Sorenson Ventures, Strawberry Creek Ventures, Bee Partners, PJC, iRobot Ventures, Boom Capital, and Kubera Venture Capital.
Download Now: Building Real-time Location Applications on Massive DatasetsThat’s a pretty solid proof point. The company is planning on using this windfall of cash to expand its R&D in the area of mixed real and synthetic data.
In a statement, Yashar Behzadi, CEO of Synthesis AI, is quoted: “Synthetic data is at an inflection point of adoption, and our goal is to develop the technology further and drive a paradigm change in how computer vision systems are built. The industry will soon fully design and train computer vision models in virtual worlds, enabling for more advanced and ethical AI.”
See also: How Synthetic Data Combats Security and Privacy Challenges in Regulated Industries
But what is synthetic data?
Synthetic data is created artificially rather than collected from the real world. Many applications are currently focused on visual data, like what you would collect from computer vision systems. Still, there’s no practical reason you couldn’t create synthetic data for other use cases, like testing applications or improving algorithms for detecting fraud. They’re a little bit like highly-structured digital twins of a physical recording.
By delivering massive, realistic datasets at scale, data scientists and analysts can, theoretically, skip over the data-gathering process and jump straight into testing or training.
That’s because most of the cost of creating real-world datasets isn’t just in collecting raw data. To use the example of computer vision and self-driving cars, carmakers and researchers can attach all sorts of cameras, radar, and LiDAR sensors to vehicles for collection, but raw data doesn’t mean anything to AI/ML algorithms. An equally tough challenge is manually labeling the data with contextual information to help the system make better decisions.
To give context to this challenge: Imagine a short drive you take on a somewhat regular basis, all of the stop signs, intersections, parked cars, pedestrians, and so on, and then imagine the herculean task of labeling every one of these potential hazards.
The core advantage of synthetic data is that, in theory, it creates perfectly labeled datasets at the scale required to properly train AI/ML applications, which means that data scientists can suddenly test out their algorithms in lots of new places before they have real-world data or in situations where it’s hard to acquire. To continue the example of self-driving cars, a data scientist could create synthetic data to train the car to drive under adverse conditions, like snow-covered roads, without sending drivers north or into the mountains to collect it manually.
Download Now: Building Real-time Location Applications on Massive DatasetsThere is, however, a chicken-and-egg problem with synthetic data because you can only create it with… more data and more AI/ML algorithms. You start with a “seed” dataset, which then becomes the baseline for your synthetic creations, which means that they’ll only be as good as the data you start with.
The (in)tangible benefits
What data scientist or researcher wouldn’t benefit from a seemingly endless generator of data to test and explore against? The core benefit—being able to avoid manually collecting real-world data—is just one of the ways in which synthetic data could accelerate AI/ML applications.
Because analysts and data scientists can tightly control the seed data, even putting in additional effort to include diversity or work with outside consultants to discover and decode bias, they can hold themselves to a higher standard. For example, Synthesis AI is developing a system for monitoring drivers’ state and carefully including diverse faces in their computer-generated synthetic dataset to ensure that the real-world applications work for everyone.
Privacy is another potential win. If a company clocks millions of miles to collect real-world data for their self-driving car, they’re gathering a whole lot of data that many would consider personal—their faces, in particular. Big companies like Google and Apple have found ways to avoid these types of problems in their mapping software, but their route isn’t viable for smaller AI/ML teams who want to test their algorithms.
“Companies are also struggling with ethical issues related to model bias and consumer privacy in human-centered products. It is clear that a new paradigm is required to build the next generation of computer vision,” CEO and founder Yashar Behzadi said to TechCrunch.
And while synthetic data does depend on a seed to get started, it can be tweaked and modified to help train AI/ML applications on edge cases that are difficult or dangerous to capture in real life. The company behind a self-driving car would want to excel at identifying objects or people who are only partially in view, like a stop sign hidden behind a parked U-Haul truck, or a pedestrian, standing between two cars, who is about to dart out into the road.
With these wins in mind, and despite any of the concerns that some have with the chicken-and-egg problem of encoding bias into synthetic data, Gartner predicts that by 2024, 60% of the data used for developing AI and analytics products will be synthetically generated. They predict much of that new data will focus on fixing forecasting models in situations where the historical data they were based on loses relevance or assumptions based on past experience break down.
But there will always be a need to collect some real-world data, so we’re still a ways off from being completely obsoleted by 3D avatars of our generic, unbiased selves.
Download Now: Building Real-time Location Applications on Massive Datasets