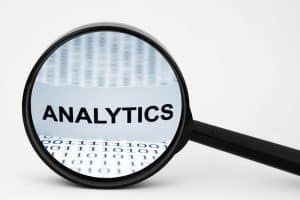
By asking your IT department to implement data analytics, you are asking them to take the focus off of what they are trained to do and dabble into new areas of technology without having the expertise to do so.
Organizations are beginning to understand the importance of using data analytics to accelerate their digital business initiatives to remain competitive, according to a Gartner Top 10 Data and Analytics Trends for 2021 report. Data analytics is shifting from being a secondary focus to a core function. However, business leaders often underestimate the complexities of data and end up missing opportunities. Another problem is that very often, they rely on their IT departments for data analytics, which can compromise the real-time value of data, making it unreliable for genuine business insights.
Your IT department is primarily concerned with maintaining security for your systems and keeping them operational. IT owns the business function of minimizing internal and external security risks and vulnerabilities and maintaining core business systems and operations. IT has service goals and focuses on help desk management, including responding to tickets, issue and resolution performance, and tracking. IT is concerned with uptime and minimizing downtime on both internal and customer-facing systems. This is critical and where you want to keep your IT department focused.
Yet, in most companies, business analysts rely upon the IT department to pull data sets for analysis. Once the data set is received, the analyst can analyze it to answer business questions, look for trends, find growth and cost-cutting opportunities, and create segmented customer lists for targeted marketing.
Yet, the IT department views query requests as less important ad hoc asks compared to keeping your systems live. As it should. The result is that by the time the business analyst gets query results, data is often stale. This means that windows of opportunity are being missed due to delayed analysis.
Further, data analytics is not the skill set of most IT department team members. Data analytics involves expertise in data management, data modeling, and machine learning. Most IT departments do not include data engineers and data scientists. Accordingly, by asking your IT department to implement data analytics, you are asking them to take the focus off of what they are trained to do and dabble into new areas of technology without having the expertise to do so.
At the same time, most IT departments are accustomed to problem-solving, and very few will tell you that they cannot add data analytics implementation to their list of projects. You end up with impatient executives wanting answers to pressing business questions, frustrated business analysts waiting for query results to be able to provide answers to the executives, and a stressed IT department not having time to focus on pulling queries.
What’s needed for data analytics?
What is needed is universal, on-demand data access in a centralized analytics platform built for non-technical business users. What is needed is self-service data availability as a ‘single source of truth’ for data combined from multiple data sources and systems. What is needed is an accurate stream of data ready for analytics in real-time so that analytic results are timely and based upon current information.
This data should be consistent no matter which department grabs a data set. This means that data access needs to originate from a centralized place to pull queries, instead of basing analytics on different ad hoc queries involving different systems that may likely create inconsistent results due to data inconsistencies across business applications. Without proper mitigation, these challenges breed data distrust.
The same is true for dashboards and reporting. If the underlying data feeding the dashboard, report, or analytics is not accurate, the results cannot be accurate. If the underlying data is not trusted, the results will not be trusted.
If a business question is posed, the results should not vary based upon which data source is queried. Data should be the same set of facts across an organization. Accordingly, data from multiple systems in the line of business applications must be (1) integrated, (2) cleansed for data quality issues, errors, and duplicate records, and (3) matched and merged to create holistic data models and views achieving a ‘golden record’ that is ready for analytics.
Modern solutions now include cloud-native data analytics solutions. These solutions have API integrations to data lakes with built-in data management for faster integration, use change data capture technology to better handle data in motion, and efficiently create a real-time analytics-ready stream of accurate information. The analytics must be powered by deep learning models, AI, or machine learning capable of mining transactional data to realize patterns, trends, opportunities, and other insights based upon transactional behaviors.
Modern solutions must include no code or low code query capabilities so that non-technical business users may perform the queries, instead of IT, without having to write SQL or other code. At the same time, modern solutions must include built-in data governance and the ability for technical users to access query code to review and edit, if desired, to gain trust in the solution, the data, and the analytic results.
Finally, modern solutions should include visualizations to better understand analytics results and should be capable of integration with preferred and custom dashboards for further visualization options and reporting. Historically, achieving this type of data integration while keeping the data cleansed as new data is created in business operations daily requires lengthy data source mapping projects for integration and large price tags for data warehouses and data management.
Data solution critical requirements:
- Built for non-technical business users
- Self-service data availability
- Cloud-native
- Fast API-based data integration
- Auto-mapping of new data sources
- Change data capture efficiency for data ingestion
- Built-in data cleansing to tackle data quality issues
- Automated real-time generation of a golden record
- of data ready for analytics
- Data lake with built-in data management
- Built-in data governance
- No code query functionality
- Machine learning-powered analytics
- Ability to include transactional data in analytics
- Visualization options for analytics results.
Data solution improvements:
- NLP query functionality
- AI, ML, and deep learning-powered analytics
- Industry-specific data model design
- Relational data models
- Relational golden records
- Audit trail of changes made to data
- Built-in Data Catalog/Data Dictionary
- Right to Be Forgotten compliance enablement
Let your IT department do what it is best at doing – maintaining security and ensuring systems are operational, minimizing risk, and maximizing uptime while decreasing downtime. A modern data analytics solution is the most efficient way to achieve critical insights, identify trends, growth, and cost-cutting opportunities, and perform well-targeted marketing to accelerate business.