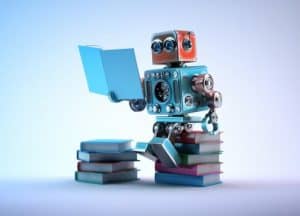
If artificial intelligence machines can take us through their experiences, they can begin to teach us new ways to solve problems.
We’ve spent so much time building machines that think the way we do. Now that we’ve partially accomplished that, it could be time to learn from our machines in ways we didn’t think possible. At the heart of this concept is to leverage the fact that many artificial intelligence applications learn over time as more data becomes available and outcomes are evaluated. If the AI systems could then share this gained knowledge with humans, computers could soon be responsible for our greatest innovative leaps.
Essentially, AI would explain how and why it made a decision or took an action, and humans would learn from this knowledge base. It is the equivalent of a new employee being mentored by a seasoned professional.
See also: Decision-Making Algorithm Aids Group Choices
Artificial intelligence black boxes do us no favors
Traditionally, such a process does not happen. AI is treated as a black box, revealing little about the way machines come to decisions. We get amazing insights from millions and billions of data points, but we cannot figure out how machines reach those conclusions.
That’s a problem when we need to understand new disease recommendations or figure out how machines choose certain candidates over others. Researchers are beginning to run after explainable AI, not just for liability or privacy purposes, but for the learning opportunity it presents for humanity.
Machines can teach us from experience
Experience is a wonderful teacher, but humans cannot hope to experience all the data needed to reach certain conclusions. This is where reinforcement learning can fill the gaps.
Machines use reinforcement learning to explore the world and come to conclusions based on those experiences. If machines can take us through their experiences, they can begin to teach us new ways to solve problems, new components of existing problems, and a whole host of other things.
Currently, machines simply spit out conclusions. We cannot retrace the steps. We cannot peer into the process. In the early days, this wasn’t an issue; we were so enamored that machines were thinking that it didn’t matter how.
The pursuit of explainable AI
The pursuit of explainable AI means access to a lot of things. We would no longer have to scrap an entire program because it came to sketchy conclusions. We’d no longer be liable for a machine’s terrible decisions based on mysterious problems with data.
Even more than that, we may finally make a huge jump in innovation. When machines can explain their fantastic solutions based on data patterns beyond our control, we may find ourselves on the cusp of a huge leap.