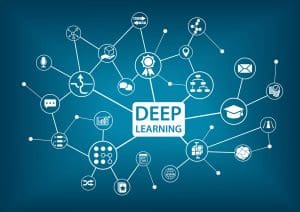
AI, ML, and deep learning have distinct purposes and capabilities and mostly work as holistic units to deliver results and accomplish tasks.
Conceptualized in the year 1955, artificial intelligence (AI) is one of the hottest topics in 2021. Over the last couple of years, we have been witnessing the surge of AI and its allied technologies like machine learning (ML) and deep learning (DL). Today, every single product or service that rolls out in the market has AI as its unique selling proposition. On the one hand, there are people talking about the brilliance of AI, and on the other end of the spectrum, AI is also being seen as a doomsday agent by theorists and enthusiasts.
Despite so much written and said about the technology, there still seems to be some misconceptions regarding AI. People confuse the terms AI, ML, and DL and often refer to the concepts interchangeably. So, what exactly are these technologies?
Are they interlinked, or are they completely different with no overlapping elements?
What Is AI?
Artificial intelligence is the technology that empowers machines to mimic human behaviors. It is all about teaching machines how to learn and think autonomously and use results to react and respond accordingly.
As we mentioned, the foundations of AI were laid as early as the 1950s. Some of the fundamental principles were established in that era on how machines could be programmed to think artificially.
Cut to today. We have full-fledged development of AI-enabled products, machines, and services reaching the masses. From autonomous cars to AI simulation programs to conduct drug trials for vaccines, we have AI assisting every single industry and market segment today.
To give you a better idea, AI is of three types –
- Narrow AI, where an AI system performs only the specific tasks it is programmed to do. Siri and Alexa are good examples of Narrow AI.
- General AI, where an AI module can perform tasks and accomplish goals like a human would do. Currently, there are no entities present we could quote as an example for General AI as they are still being studied and experimented with.
- Super AI, where AI systems can surpass the intelligence of humans and can execute tasks better than us. Such systems are evolved forms of General AI.
Machine learning
As kids, when we put our hands on a hot ladle of soup, we learned what hot and heat meant. Since then, we are conscious when we deal with hot elements. Well, this is exactly what machine learning enables machines to do. ML is all about training machines to detect patterns, learn and adapt based on outcomes and results.
To achieve this level of efficiency, machine learning involves algorithms. Unlike other computer programming codes, algorithms in ML are not linear. Meaning they don’t simply execute commands blindly. ML is designed to think and train autonomously to spot anomalies and patterns and learn automatically. Through consistent training, ML can evolve into systems that can convert arbitrary data into specific results.
Similar to AI, machine learning has three classifications:
- Supervised learning, where an ML module is trained with the help of datasets with preloaded results. You could loosely translate this to showing and teaching. Supervised learning is further classified into two – classification and regression. While the former classifies an element, the latter predicts an outcome.
- Unsupervised learning – this is the opposite of supervised learning, where modules learn by themselves. No datasets are present to give examples or show how things are done. Systems autonomously establish patterns, correct themselves, learn and adapt.
- Reinforcement Learning – when an ML system is rewarded for correct outcomes and penalized for wrong results, it’s called reinforcement learning. Through the process, the systems autonomously device algorithms that feature high rewards and low penalties.
Deep learning
Let’s understand neural networks to understand deep learning. Like the human brain, AI systems have artificial neural networks that function on neurons connecting them. Every single neuron is an input embedded with parameters and distinct weightage. It executes a specific task. This information-carrying neuron is also an input for another neuron, thus making the entire system one complicated web of neurons and connected networks.
Now, deep learning is all about working with such complex networks and the depth of them. How deep? Well, any network that has more than one layer hidden is considered a deep network.
There are two types of neural networks actively used today –
- Convolutional neural networks – deployed for concepts like video analysis, NLP (Natural Language Processing), image recognition, and more
- And Long Short-term Memory – deployed for speech recognition, the prediction of time series, text and image classification, and more.
Are AI, ML, and deep learning interconnected?
Yes, they are interconnected and different at the same time. They are interconnected because artificial intelligence is the holistic term or the umbrella concept under which machine learning falls. Deep learning is the subset of machine learning. Visualize this as Russian Matryoshka dolls, one within each other.
If autonomous cars are synonymous with AI, the algorithms are the machine learning modules, and the neural networks that transmit information and data are part of deep learning functionalities.
Wrapping Up
AI, ML, and DL have distinct purposes and capabilities and mostly work as a holistic unit to deliver results and accomplish tasks.
As we continue to learn and study more about AI and its subsets, let’s wait and witness the rise of General AI and Super AI to find out if newer concepts ultimately emerge.