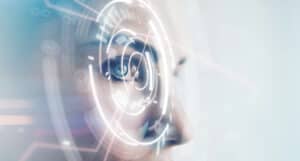
The Visual State Space Duality (VSSD) model, introduced by researchers at City University of Hong Kong and Tianjin University, offers a groundbreaking approach to vision transformers, significantly improving efficiency and performance in computer vision tasks.
As companies implement computer vision in more aspects of daily operations, achieving high efficiency and performance in processing image data is crucial. Traditional vision transformers have advanced capabilities but come with high computational costs. However, one research team has introduced the Visual State Space Duality (VSSD) model, a breakthrough in vision transformers designed to improve efficiency and performance in computer vision tasks. This model addresses the high computational demands of traditional vision transformers, making it a robust solution for processing long sequences of image data.
Streamlining Vision Models
Traditional vision transformers, while powerful, are resource-intensive, especially for long sequences. State Space Models (SSMs) emerged as an efficient alternative, offering linear computational complexity. However, the inherent causal nature of SSMs limited their application in vision tasks, where image data processing is naturally non-causal. The VSSD model overcomes this by discarding the magnitude of interactions between the hidden state and tokens, focusing instead on their relative weights. This change allows VSSD to process data in a non-causal format, significantly improving both efficiency and performance.
The VSSD model captures motion information from different perspectives and integrates it with historical data stored in short-term memory. This method enhances the perception of dynamic objects, ensuring consistent and accurate predictions. The model employs a voting mechanism to refine these predictions using long-term memory, maintaining accuracy across various frames.
See also: The Crucial Role of Machine Vision and AI in Modern Manufacturing
Performance and Applications
Extensive experiments demonstrate that VSSD surpasses existing state-of-the-art SSM-based models in image classification, detection, and segmentation tasks. The model shows improved efficiency, making it suitable for applications in autonomous driving and mobile robotics, where processing speed and accuracy are critical.
VSSD’s innovative approach to handling non-causal vision data sets it apart from previous models. By transforming the causal properties of traditional SSMs, VSSD retains the global receptive field and linear complexity benefits and enhances training and inference speeds. The model’s superior performance on various benchmarks indicates its potential to revolutionize computer vision, offering a more efficient and accurate solution for complex visual tasks.
The introduction of VSSD marks a significant advancement in computer vision. By addressing the limitations of traditional vision transformers and SSMs, VSSD provides a robust, efficient, and accurate solution for processing long sequences of image data. This model is poised to significantly impact various industries, particularly those relying on advanced visual processing capabilities.