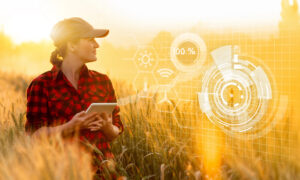
Neural networks help growers to turn satellite images into clear, actionable maps for data-informed decisions on sustainable food production and environmental care.
In the heart of every farmer’s field lies a delicate balance between nature and innovation. As humanity faces the dual challenges of feeding a growing population and preserving the planet, agriculture is undergoing a profound transformation. At the forefront of this change are neural networks — advanced algorithms inspired by the human brain that are reshaping how we understand, predict, and optimize farming practices.
By harnessing the power of neural networks, agriculture is moving from intuition-driven decisions to data-informed precision. These tools offer a way to unravel the complexity of natural ecosystems, turning raw data into actionable insights. From predicting weather patterns to diagnosing crop diseases, neural networks are opening doors to solutions that were once out of reach, promising to make farming more efficient, sustainable, and resilient.
Deep Learning with Artificial Neural Networks
Deep learning with artificial neural networks is revolutionizing how we interact with and interpret data. Inspired by the structure of the human brain, these computational models are designed to process vast amounts of information, identify patterns, and make decisions with astonishing accuracy. From live satellite maps that monitor Earth’s changes in real time to tools that optimize everyday operations, neural networks are shaping a future where data-driven insights redefine what’s possible. Their ability to “learn” from data through training makes them a cornerstone of modern technology, enabling applications that once belonged to the realm of science fiction.
Training a neural network is akin to teaching a student. We provide it with data (the curriculum) and a goal (the task it must master). Through an iterative process involving layers of interconnected nodes, the network adjusts its internal parameters, improving its predictions or classifications over time. Feedback mechanisms, like backpropagation and optimization algorithms, act as teachers, fine-tuning the network’s understanding until it achieves the desired level of accuracy.
The magic of training lies in the data. Clean, diverse, and well-labeled datasets ensure the network learns the right lessons, while powerful computing systems make the training process feasible for complex tasks. The result? A neural network capable of making sophisticated decisions in real-world scenarios.
Artificial neural networks can solve all types of problems where the presence of a pattern, trend, or correlation within large volumes of data needs to be unraveled. Further, they can also address different types of problems, from simple classification to higher-order predictive modeling. For instance:
- Classification Tasks: Neural networks can sort images into categories, such as identifying whether a photo contains a cat or a dog. They’re also used in medical imaging to distinguish between healthy and diseased tissue or in spam filters to separate legitimate emails from junk.
- Prediction Tasks: Neural networks are exceptional at forecasting trends, such as predicting stock market movements, estimating demand for products, or forecasting weather. In agriculture, they predict crop yields based on soil conditions and weather data.
By seamlessly handling these tasks, neural networks are empowering industries to become more efficient, adaptive, and forward-looking. Whether it’s guiding autonomous vehicles, diagnosing medical conditions, or enabling smarter supply chains, deep learning continues to unlock the potential of artificial intelligence, transforming data into meaningful insights that shape our world.
See also: Sustainability and Reliability: The Future of Agriculture Technology Lies in Microgrids
Building Process of a Neural Network
Building a neural network is much like creating a masterpiece of art in which every step in the process has to go just right. The data acquisition starts the process, using raw information as its building blocks. High-quality datasets are everything, whether pictures, words, or even numbers; for visual purposes, that could mean assembling up-to-date satellite images to get all the angles of the Earth. The more accurate and representative the data, the better the network learns.
Next comes the stage of data preprocessing, where cleaning, organization, and preparation of data are done to make sure the neural network focuses on the relevant features. Following this, the architecture is designed: a decision is made on the number of layers and nodes to balance complexity with efficiency. The network is then trained by passing the data through the system, refining its accuracy through algorithms with each iteration. Finally, validation and testing ensure that the model performs well in real-world scenarios, completing the journey from concept to a powerful AI tool.
Each stage plays a critical role, transforming raw inputs into actionable insights, whether predicting trends, classifying objects, or interpreting the real-time satellite view of our planet.
Classification of Crops
As neural networks have become invaluable tools for modern agriculture, one of their most particular values lies in classifying crops with remarkable precision. By analyzing recent satellite images, these models can identify specific crop types by recognizing their distinct spectral patterns. This level of classification provides farmers and agricultural organizations with critical insights into crop distribution, health, and growth patterns. It also enables better decision-making for planting schedules, pest control, and irrigation management. With neural networks continuously learning from newer datasets, the accuracy and reliability of crop classification keep improving, driving more sustainable and efficient agricultural practices.
Field Delineation in Practice
Besides crop predictions, neural networks also outshine in field delineation — a vital aspect of precision farming. Using data from a live satellite view, these models can detect and outline field boundaries with impressive accuracy, even in landscapes with overlapping or irregular features. This capability allows for improved land management, helping farmers allocate resources effectively and monitor field-specific activities. Furthermore, accurate field delineation helps in yield estimation and monitoring of soil conditions and prevents the overuse of fertilizers and pesticides. Neural networks help growers to turn satellite images into clear, actionable maps for data-informed decisions on sustainable food production and environmental care.