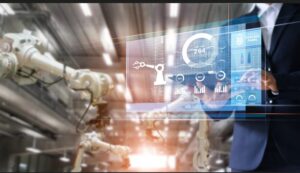
The future of digital twins in intelligent manufacturing is promising and highly dependent on the ability to fuse and analyze data from multiple sources.
Manufacturers face increasing pressure to improve efficiency, reduce costs, and adopt sustainable practices. On top of this, continued disruptions to the supply chain and an increasing focus on personalization make manufacturing an enormous balancing act. Traditional methods of addressing these challenges often fall short because of limitations in real-time insights and predictive capabilities.
But digital twins— virtual counterparts to physical assets and processes—are helping ease roadblocks to modern manufacturing. They offer unprecedented visibility and control over their operations, not simply as they are or might be in a few months, but as they could be over infinite scenarios. Manufacturers can simulate various scenarios, predict potential issues, and make data-driven decisions, giving manufacturing a dynamic capability and enabling immediate adjustments and long-term strategic planning. Here’s how it’s creating a better, more sustainable practice.
Overview of Digital Twin Technology
Digital twin technology aims to reduce product development cycles and costs through continuous feedback between the virtual and real worlds, data fusion analysis, and iterative decision-making optimization. The technology addresses several key challenges in traditional manufacturing, such as poor real-time monitoring, difficulty in diagnosing equipment failures, and significant errors during machining.
What Technology Supports Digital Twins
Recent research has focused on the integration of digital twins into intelligent manufacturing. This includes developing a comprehensive understanding of the basic concepts and technical pathways required to create an interactive system between the virtual and real worlds. Essential technologies for digital twins include:
- Multimodel Fusion: This combines various models to create a cohesive and accurate representation of the physical asset. By integrating different data sources and models, digital twins can provide a more comprehensive view of the asset’s behavior, improving predictive accuracy and decision-making.
- Data Sensing: Collecting real-time data from sensors embedded in physical objects is crucial for maintaining an up-to-date digital twin. This data provides the input for the digital twin to reflect the current state of the physical asset, enabling real-time monitoring and adjustments.
- Algorithm-Based Prediction: Using advanced algorithms to predict future behaviors and potential issues allows digital twins to anticipate problems before they occur. This predictive capability supports proactive maintenance and optimization, reducing downtime and improving efficiency.
- Intelligent Decision-Making: Enabling automated and optimized decision-making processes ensures that digital twins can respond quickly and effectively to changing conditions. This reduces the need for human intervention and enhances the overall responsiveness and agility of manufacturing operations.
Some organizations like NIST are undertaking more rigorous studies to help organizations better understand, and later implement, what is shaping up to be transformative technology.
Applications in Intelligent Manufacturing
Digital twins are applied throughout the product life cycle, including design, manufacturing, and service stages. According to the paper above, digital twins have shown significant promise in the manufacturing phase. For instance, a model based on heat conduction theory and visualization was used to construct a time-varying error model for the motion axis. The experiments accurately predicted the time-varying error in the hole spacing of a workpiece, with a minimal prediction error of just 0.2 micrometers compared to the actual error. By compensating for these errors in real-time, the variability in the hole spacing error was reduced by an impressive 69.19%. A small change with big results.
Advancements in Digital Twin Technology
Digital twin technology is evolving rapidly, driven by advances in computing power, analytics approaches, and artificial intelligence. McKinsey highlights that these advancements have led to the emergence of digital twins as digital replicas of current or future products capable of simulating all the characteristics of their physical counterparts. Interacting with or modifying a product in a virtual space can be quicker, easier, and safer than doing so in the real world.
Impact on Product Development
Product development leaders expect digital twins to accelerate product development processes and improve outcomes, all while reducing costs. McKinsey reports that the global market for digital-twin technologies is forecast to grow at about 60 percent annually over the next five years, reaching $73.5 billion by 2027. In advanced industries, survey data indicate that almost 75 percent of companies have already adopted digital-twin technologies that have achieved at least medium levels of complexity.
See also: Factory Resets: Digital Twins Add New Dimension to Industrial Settings
Roadmap for Implementing Digital Twins
Implementing digital twins in manufacturing involves several key steps:
- Process Planning: Define the scope and objectives of the digital twin initiative, identifying the specific assets and processes to be digitized.
- Process Validation: Ensure the accuracy of data sources and validate the digital twin’s performance through pilot projects and simulations.
- Production Planning: Integrate the digital twin into production workflows, aligning it with existing systems and processes.
- Production Execution: Deploy the digital twin in live production environments, continuously monitoring and optimizing its performance.
McKinsey further outlines three archetypes companies can choose when integrating digital-twin technology into the organization:
- Separate Business Unit: Creating a distinct portfolio of digital assets sold alongside corresponding physical assets.
- Integrated into Existing Business Unit: Developing and supporting digital-twin applications associated with the products of that business unit, gaining experience with limited synergies.
- Center of Excellence: Operating as a separate center of excellence that supports the development of applications within existing business units, ensuring consistent processes and technology components.
See also: How Industrial Connectivity Enables Manufacturing Automation and Insights
Future Trends and Key Technologies
The future of digital twins in intelligent manufacturing is promising, with ongoing research focusing on several key areas:
- Enhanced Data Integration: Improving the ability to fuse and analyze data from multiple sources for more accurate and comprehensive digital twins.
- Real-Time Optimization: Advancing real-time optimization techniques to further enhance the efficiency and responsiveness of manufacturing processes.
- Broader Application Areas: Expanding the use of digital twins beyond traditional manufacturing to areas such as predictive maintenance, supply chain optimization, and more.
- Sustainability: Leveraging digital twins to drive more sustainable manufacturing practices by minimizing waste and reducing energy consumption.
This ongoing research and development in digital twin technology provide a solid foundation for further advancements in intelligent manufacturing. By continuously enhancing the interaction between the virtual and real worlds, digital twins will play a crucial role in shaping the future of manufacturing, leading to more efficient, cost-effective, and sustainable operations.