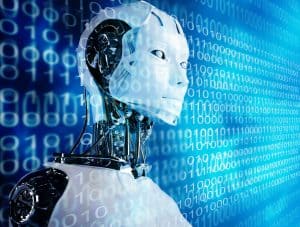
Getting robots to learn is quite similar to how we teach humans, and UC Berkeley may have found a way to speed up the process with deep learning.
Getting robots to complete tasks involves a lot of human-like training, essentially showing the task to the robot and having it repeat and repeat. But there are steps in-between that can speed up the process, researchers at the University of California Berkeley have published another one.
Named AVID, the framework is based on two deep-learning models also developed by UC Berkeley, CycleGAN and SOLAR. CycleGAN reduces images of a human performing a task to the pixel level, which can then be restructured to show the robot completing the same task.
SEE ALSO: IBM Robot Returns To International Space Station
“AVID works by having the robot observe a human perform some task then imagine what it’d look like for itself to perform the same thing,” said UC Berkeley robotics research assistant, Laura Smith. “To learn how to actually achieve this imagined success, we let the robot learn by trial and error.”
Instead of having the human operator reset the task each time the robot fails, the robot automatically resets it. This reduces the amount of intervention needed when setting a task, allowing some form of autonomy. “We design our training framework to be amenable to learning long-term behavior with minimal effort,” said Smith.
In tests, AVID was able to outperform pixel-space ablation, TCN, imitation ablation, and robot demonstrations with observations. Moreover, it managed to complete these tasks with far less direct intervention from humans, which could be useful for developers looking to program thousands of robots to perform tasks.
One of the limitations of the current form of AVID is that robots need to be taught every task, and the knowledge learned cannot be passed on to new tasks. In the future, Smith and her colleagues hope to make CycleGAN training a one-time exercise for a wide range of tasks and skills.