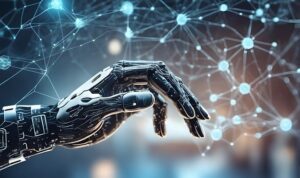
Instead of focusing on AI model comparisons or rushing to deploy AI across all business users, leaders should build structured frameworks that guide AI adoption gradually and strategically.
Undoubtedly, generative AI (GenAI) is here to stay. Every day, there are numerous examples of its usefulness in application after application. However, like all new technologies, the hard part is going from its limited use by individuals and departments to one where GenAI becomes an integral part of an entire organization’s operations. That was the focus of a talk by Kurt Schlegel, a longtime Gartner analyst specializing in analytics, at this week’s Gartner Data & Analytics Summit in Orlando, Florida.
Specifically, Schlegel’s talk, “Top 5 Analytics and AI Challenges and How to Handle Them,” focused on the issues organizations face when trying to integrate GenAI into their analytics and business intelligence applications and workflows. He noted the transformative nature of AI in analytics also brings significant challenges that organizations must navigate.
These challenges span from technological fears to governance concerns, financial implications, and structural changes in how analytics teams operate. His insights provide a roadmap for organizations looking to move from AI experimentation to full-scale production. Here are some of the key points he raised.
1. From Fear to Trust: Addressing AI Skepticism and Risk Management
One of the most significant barriers to AI adoption is fear…fear of errors, misinformation, security risks, and unintended consequences. Generative AI’s ease of use can be deceptive, leading business leaders to assume it can automatically generate accurate and meaningful analytics. However, underlying complexities such as query accuracy, table joins, and data governance make AI implementation far more intricate.
To address this, Schlegel advocates for transparency, explainability, and responsible implementation:
- Organizations must clearly communicate AI’s limitations and ensure users understand that AI-generated insights are probabilistic, not absolute.
- AI models should be accompanied by error metrics like Mean Absolute Percentage Error (MAPE) to prevent blind trust in AI-generated data.
- Trust must be built through rigorous development, testing, and production (Dev-Test-Prod) cycles, ensuring that AI models are validated before being used for critical business decisions.
Additionally, Schlegel highlighted how the collaboration between domain experts and data scientists is crucial in creating AI models that are both powerful and interpretable. Without this partnership, AI-driven insights risk being dismissed or misused.
See also: Overcoming the Top Barriers to GenAI Adoption In the Enterprise
2. From Cost to Benefit: Balancing AI’s Financial Investment with ROI
The financial implications of generative AI cannot be ignored. Schlegel pointed out that AI computation is expensive, and organizations must be cautious about escalating costs related to token usage, cloud processing, and data storage. A seemingly minor increase in AI query volume can quickly lead to massive operational expenses.
To mitigate financial risks, organizations should:
- Start AI adoption with specialists (e.g., data engineers and data scientists) rather than opening access to all business users, which could lead to uncontrolled costs.
- Use AI to enhance efficiency, reducing bottlenecks caused by limited data science resources.
- Monitor AI expenses using FinOps strategies, ensuring costs are aligned with business value.
- Develop gradual deployment strategies (proof-of-concept → pilot → production) to prevent wasteful investments in AI projects that don’t yield meaningful benefits.
Schlegel likened AI’s cost evolution to the early days of mobile phones, where calls were expensive but became more affordable as adoption scaled. Similarly, generative AI’s costs may decline over time, but organizations must manage spending strategically until economies of scale kick in.
3. From Chaos to Order: Establishing AI Governance and Analytical Sandboxes
Generative AI, if mismanaged, can lead to data inconsistencies, security vulnerabilities, and decision-making chaos. Organizations must balance freedom for exploration with governance for reliability.
Schlegel compared AI governance to a ski resort model:
- Beginners (new users) should operate in controlled environments with structured guidelines.
- Intermediate users should have flexibility with oversight.
- Advanced users should be given autonomy but still follow governance best practices.
To establish order, organizations should:
- Create analytical sandboxes where AI models can be tested without impacting critical business operations.
- Implement strict governance models to ensure AI-driven insights are based on trustworthy and consistent data.
- Use role-based access controls to prevent unauthorized AI usage.
Schlegel cited General Mills as an example of a company that successfully balanced freedom and control by implementing a structured governance framework, ensuring AI adoption was both innovative and reliable.
4. From Technology to Solution: Shifting Focus from AI Tools to Business Impact
While AI technology is complex, analytics leaders should focus less on AI technical intricacies (e.g., which LLM is superior) and more on real business solutions.
Schlegel emphasized that AI should not be treated as a technology-first initiative but rather as a problem-solving tool that improves:
- Resource allocation (e.g., optimizing budgets, inventory, workforce planning).
- Conversion rates and customer engagement through data-driven personalization.
- Forecasting accuracy allows companies to make better strategic decisions.
Instead of fixating on model architecture or debating OpenAI vs. Google Gemini vs. Anthropic Claude, leaders should prioritize AI’s practical applications, ensuring it solves tangible business problems.
5. From Owning to Influencing: Redefining the Role of AI Leadership
Unlike traditional analytics, which can be centrally managed, a single team cannot own generative AI. AI is inherently diffused across different departments, requiring a new leadership approach based on influence rather than control.
Schlegel introduced the “franchise” model for AI governance:
- Centralized teams provide global consistency, best practices, and governance.
- Decentralized teams (or “franchises”) maintain local autonomy, tailoring AI applications to specific business needs.
This model resembles hub-and-spoke architectures, where a core team sets the AI strategy, but individual business units drive execution.
Schlegel’s recommendation for AI leaders:
- Act as AI enablers, not gatekeepers—ensuring AI is widely accessible but responsibly used.
- Educate and empower business leaders to leverage AI responsibly within their domains.
- Encourage an iterative approach, where AI solutions evolve based on real-world feedback rather than top-down mandates.
Conclusion: Balancing Optimism with Pragmatism
Schlegel closed his talk by reinforcing the need for a balanced mindset when integrating generative AI. While AI has the potential to revolutionize analytics, organizations must proceed cautiously, ensuring that AI adoption aligns with business value, governance, cost-efficiency, and ethical considerations.
Instead of focusing on AI model comparisons or rushing to deploy AI across all business users, leaders should build structured frameworks that guide AI adoption gradually and strategically. By addressing these five challenges head-on, organizations can transform generative AI from an experimental tool into a trusted driver of business success.
Salvatore Salamone contributed to this article.