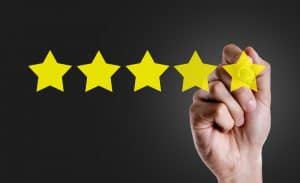
Here is a quick overview of the major real-time analytics developments and innovations that got our readers’ attention over the last year.
As the year comes to an end, it is the perfect time to look back at the trends, technologies, and issues that shaped the real-time analytics marketplace. Our most-read articles at RTInsights covered a wide range of topics including open source tools for deep learning, bee colony behavior algorithms that improve speech recognition, infrastructure challenges to support continuous intelligence and IoT, explainable artificial intelligence (AI), innovative use cases for IoT, the benefits of digital transformation, and more. With that said, here are our top 10 articles for 2019.
1) Top Open Source Tools for Deep Learning
Popular open-source tech can help future-proof your teams. Set up your analytical teams for success by arming them with open source libraries running on an open-source platform. This will future-proof against lock-in by any single AI tool or cloud vendor. But doing so means keeping up with up fast-moving developments, so your enterprise can quickly adopt promising technology as it emerges.
Read the article here.
2) Researchers Surpass Speech Recognition Methods with Help from Honeybees
Speech recognition software has come a long way since the launch of Siri, but most commercial services still suffer from accuracy issues, especially with non-English speaking users. While the artificial intelligence at work behind the scenes should be able to parse different languages with ease, there are large variations in languages across the world. Improved speech recognition is achieved using an artificial neural network that uses opposition-based learning and bee colony behavior algorithms.
Read the article here.
3) Infrastructure Architecture Requirements for Continuous Intelligence
Continuous intelligence (CI) takes real-time analytics to new levels. It uses predictive analytics, artificial intelligence (AI), and machine learning (ML) on streaming data to get insights into events as they are happening. To reap the full benefits of CI, and take actions in milliseconds to minutes, businesses need to update their infrastructure architectures to accommodate data in motion.
Read the article here.
4) Will 5G Have a Role in Providing CI Apps with IoT Data?
The heart of any continuous intelligence (CI) effort is having access to real-time data to make decisions based on events as they are happening. The plethora of smart sensors and Internet of Things (IoT) devices organizations use (or plan to use) in their CI efforts means large amounts of structured and unstructured data will be available for analysis. The challenge is how to get access to that data in a timely manner. Some see 5G (fifth-generation cellular) services playing an important role.
Read the article here.
5) 8 Digital Transformations Coming in 2020
2020 is the year many analysts and industry experts have marked as the start point of new technologies coming online. Developments are expected in areas ranging from mobile operators rolling out 5G in earnest next year to many car manufacturers launching their first autonomous vehicles. Here are a few of the digital transformation trends expected to have the greatest impact in 2020.
Read the article here.
6) Explaining “Black Box” Neural Networks
Countless organizations hesitate to deploy machine learning algorithms, given their popular characterization as a “black box.” While their mathematical equations are easily executed by AI, deriving a human-understandable explanation for each output is often difficult. The result is that, especially in regulated industries, machine learning models that could provide significant business value are often not deployed into production. To overcome this challenge, FICO is developing a patent-pending machine learning technique called Interpretable Latent Features. Instead of explaining AI as an afterthought, as in many organizations, its new technique brings an explainable model architecture to the forefront. The approach is derived from FICO’s three decades of experience in using neural network models to solve business problems.
7) How 5G Could Improve Food Traceability
5G networks have the potential to enable dynamic, end-to-end food traceability – thanks to an ability to connect to many low-power devices, like embedded Internet of Things sensors at the same time. As a result, the food supply chain will be able to modernize its practices and processes further, becoming super-interconnected and better equipped to leverage technologies like blockchain and AI. That will increase the accountability of food businesses in the event of foodborne illness outbreaks, help organizations better mitigate food safety risk, and streamline costs for companies and, in turn, consumers. But with all this promise, how do we get there?
Read the article here.
8) Beyond Devices: The Second Wave of IoT Emerges
Many companies have mastered the ins and outs of the Internet of Things, designing and building networks that respond to and exchange data with arrays of sensors, devices, and remote systems. However, until now, much of this activity has been transactional and mechanical in nature. The emerging next phase of IoT needs to be more intelligent and human-centric — conforming to and enhancing the flows of human activity, whether from room to room in a building, or enabling collaboration across a network.
Read the article here.
9) Solving Real-Time Needs as Enterprise Leaders Race Ahead with ML and AI
Making data quickly actionable creates difficult challenges for the old data management order. Given the raft of challenges, more and more companies are experimenting with using a “single backbone” data automation foundation for building the Internet of Everything (IoE) applications. This requires an open, flexible platform for building real-time, scalable, data-automation, and AI solutions that can handle scalable data ingestion, normalization, and enrichment along with real-time business logic, data storage, and decision analytics.
Read the article here.
10) AI’s Empathy Problem
The frontline of many of today’s enterprise AI systems is customer service — chatbots and online response systems that attempt to deliver friendly, informed services on demand. Some systems come close, but AI may not be ready to deliver the kind of empathy that a live human agent can provide – such as going the extra mile to help a customer. The problem: human emotions and reactions are complex, and likely too much for AI to handle today, beyond shallow engagements or analysis. This is the next frontier for AI.
Read the article here.