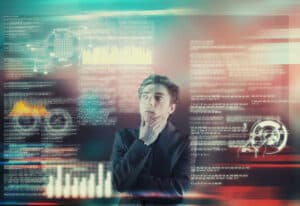
Most businesses are new to artificial intelligence and face daunting challenges when trying to scale their efforts that seek to derive business value from data.
Get artificial intelligence right, and generate $460 billion in additional revenues. That’s the estimated gains today’s companies may see if they do three things: improve data practices, trust in advanced AI, and integrate AI with business operations. However, most companies have not gotten the memo.
That’s the word from Infosys Knowledge Institute, which finds in its latest study that while the potential for AI-driven gains are significant, most companies are still struggling to “convert data science to business value.”
Part of the reason is AI is still relatively new. “Most businesses are new to AI and face daunting challenges to scale: 81% of respondents deployed their first true AI system in only the past four years, and 50%, in the last two,” the Infosys study suggests. The report also found that 63% of AI models function only at basic capability, are driven by humans, and often fall short on data verification, data practices, and data strategies. Plus, only 26% of practitioners are highly satisfied with their data and AI tools.
See also: The 4th Shift in the Evolution of Finding Insights from Data
The study’s authors conclude that high-performing companies – 21% of the sample – think differently about AI and data, and these leaders focus in three areas:
They transform data management to data sharing. “Companies that embrace the data-sharing economy generate greater value from their data. Data increases in value when treated like currency and circulated through hub-and-spoke data management models ($105 billion incremental value). Companies that refresh data with low latency generate more profit, revenue, and subjective measures of value.”
They emphasize data trust over mere data compliance. Companies highly satisfied with their AI have consistently trustworthy, ethical, and responsible data practices. These prerequisites tackle challenges of data verification and bias, build trust, and enable practitioners to use deep learning and other advanced algorithms.”
They extend the AI team beyond data scientists: “Businesses that apply data science to practical requirements create value. For intelligent data, business and IT are much better together.”
Combined, these areas not only scale AI usage but unlock its potential value – transforming AI dreams to insights and operational effectiveness and improving the human experience. Infosys research found the financial services industry recorded the strongest satisfaction with its data and AI uses, followed by retail and hospitality, healthcare, and high tech.
The study’s authors offer the following suggestions:
Get your data right, and share it: “Data management startegues that foster data sharing, both importaing in and sharing out, expand the universe of available data.” Move toward a “hub-and-spoke strategu with a centralizd platform and technology , but also providing teams flexibility to operate on their own. “The hub is a common repository that defines the data and its location. The spokes, radiatig from the hib, contain and sharee data as dictated by buisiness specialists and AI teams.”
Build trust in advanced AI: “Companies have a lot of value in their data that trhey have not un;locked. This is leading to a realization that they cannot approach data and AI as individual use cases or projects. They must extablish an enterprose-wide strategy to discover, democratize and de-risk AI implertmattion at scale.”
Compose an AI team biased to to business value. “The mission of the AI team is to deliver democratized tools to employees, customers, and partners to enable them to develop and use data responsibly, accurately, and ethically across their entire ecosystem.”