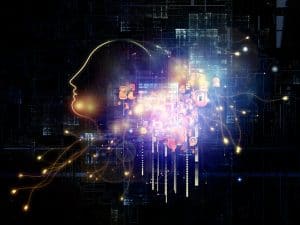
To attain true AI understanding, researchers should shift their attention to developing a basic, underlying AGI technology that replicates the contextual understanding of humans.
Industry giants like Google, Microsoft, and Facebook, research laboratories such as Elon Musk’s OpenAI, and even platforms like SingularityNET are all betting that Artificial General Intelligence (AGI) – the ability of an intelligent agent to understand or learn any intellectual task that a human can – represents the future of AI technology.
Somewhat surprisingly, though, none of these companies is focused on developing a basic, underlying AGI technology that replicates the contextual understanding of humans. That likely explains why the research being done by these companies depends entirely on an intelligence model that possesses varying degrees of specificity and relies on today’s AI algorithms.
Unfortunately, that dependence means that, at best, AI can only give the appearance of intelligence. No matter how impressive their capabilities are, they still follow predetermined scripts that contain numerous variables. As a result, even massive, highly sophisticated programs such as GPT3 or Watson only appear to exhibit understanding. In actuality, they have no understanding that words and images represent physical things that exist and interact in a physical universe. The concept of time or the idea that causes have effects is completely foreign to them.
See also: Exploring Artificial Intelligence Variants and Their Uses
That’s not to take anything away from what today’s AI is able to do. Google, for example, is able to search volumes of information at an incredible speed to provide the results the user wants (at least most of the time). Personal assistants such as Siri can make restaurant reservations, find and read emails, and give directions in real-time. The list goes on and on and is constantly being expanded and improved.
But no matter how sophisticated these programs are, they are still looking for input and making specific output responses that depend entirely on the data sets at their core. If you don’t believe me, ask a customer service bot a question that is “off-script,” and the bot will likely generate a response that makes no sense or no response at all.
Bottom line, Google, Siri, or any of the other current examples of AI lack true, common-sense understanding, which ultimately will prevent them from ever advancing to Artificial General Intelligence. The reasons for this can be traced back to the principal assumption underlying most AI development over the past 50 years, namely that simple intelligence problems would fall into place if the difficult problems could be solved. Best expressed as Moravec’s Paradox, this assumption holds that it is relatively easy to make computers exhibit adult-level performance on intelligence tests but difficult to give them the skills of a one-year-old when it comes to perception and mobility.
False, too, was the assumption made by AI researchers that if enough narrow AI applications were built, they eventually would grow together into a general intelligence. Unlike the way a child might effortlessly integrate vision, speech, and the other senses, narrow AI applications are unable to store information in a generalized way that allows that information to be shared and subsequently used by other AI applications.
Finally, researchers mistakenly assumed that if a big enough machine learning system with enough computer power could be built, it would spontaneously exhibit general intelligence. This also proved to be false. Just as expert systems which attempted to capture the knowledge of a specific field were unable to create enough cases and example data to overcome an underlying lack of understanding, AI systems are unable to deal with “off-script” requests, regardless of how large and variable their data sets are.
Artificial General Intelligence fundamentals
To attain true AI understanding, researchers should shift their attention to developing a basic, underlying AGI technology that replicates the contextual understanding of humans. Consider, for example, the situational awareness and contextual understanding displayed by a 3-year-old child playing with blocks. The 3-year-old understands that blocks exist in a three-dimensional world, have physical properties like weight, shape, and color, and will fall down if stacked too high. The child also understands the concepts of causality and the passage of time since the blocks can’t be knocked down until they first have been stacked.
The 3-year-old can also become a 4-year-old, and then a 5-year-old, and eventually a 10-year-old, and so on. In short, the capabilities of the 3-year-old innately include the ability to grow into a fully functioning, generally intelligent adult. Such growth is impossible for today’s AI. No matter how sophisticated it is, today’s AI remains completely unaware of its existence in its environment. It has no understanding that an action it takes now will impact its actions in the future.
While it is unrealistic to think that an AI system that has never experienced anything outside of its own training data would be able to understand real-world concepts, adding mobile sensory pods to the AI holds the potential for an artificial entity to learn from a real-world environment and demonstrate a fundamental understanding of physical objects, in reality, cause and effect and the passage of time. Just like that 3-year-old, the artificial entity equipped with sensory pods is able to learn first-hand about stacking blocks, moving objects, performing sequences of actions over time, and learning from the consequences of those actions.
With vision, hearing, touch, manipulators, etc., the artificial entity can learn to understand in ways that are simply impossible for a purely text-based or a purely image-based system. As previously noted, such systems simply can’t understand and learn no matter how large, and variable their data sets are. Once the entity has gained this ability to understand and learn, it may even be possible to remove the sensory pods.
While we are unable to quantify how much data it might take to represent true understanding at this point, we can speculate that a reasonable percentage of the brain must pertain to understanding. Humans, after all, interpret everything in the context of everything else already experienced and learned. As adults, we interpret everything within the context of the understanding we learned in the first few years of life. Given that, it seems likely that true Artificial General Intelligence will only be able to fully emerge once the AI community recognizes this fact and takes the necessary steps to establish a fundamental basis for understanding.