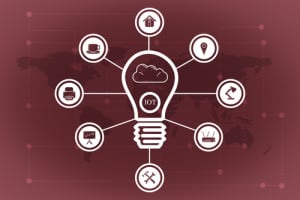
Teradata eyes predictive maintenance, asset performance and energy use as applications of cloud-based IoT analytics.
Teradata, known for its data warehousing capabilities and marketing applications, is ramping up its offerings in IoT analytics.
“We are seeing a spike in interest in the Internet of Things,” explained Dan Graham, director of technical marketing for Teradata Corp. The company, he stressed, does not make IoT sensors, and while it remains a database company, its main business these days is using Big Data analytics to help other companies create more profit from a venture.
On April 18, Teradata announced the creation of a global IoT analytics unit within Teradata Labs. The “special-ops” team, as Teradata calls it, will be composed of data scientists, data engineers and software designers that will develop cloud-based analytics solutions for the IoT.
Analytics will include machine learning, including for system administration and DevOps tasks. Use cases will include predictive maintenance, energy use and pay-per-use models, as well as applications in asset monitoring: (idle time, geo-positioning, and energy/fuel use).
Teradata also rolled out innovations to its hybrid cloud environment for its data warehouse line. Innovations include Intelliflex, an in-memory, massive parallel processing architecture designed to scale with data growth and eliminate downtime; the availability of Teradata Database ( a hybrid row/column database) on Amazon Web Services; expansion of its managed cloud product to Europe; and a set of services to ease hybrid cloud adoption.
Teradata said the new hybrid cloud products are designed to give businesses flexibility and speed in deploying analytics.
Listener and Aster Analytics
The announcement of a global IoT analytics unit and extended cloud offerings builds on two new software capabilities Teradata announced last October: Teradata Listener and Aster Analytics on Hadoop.
Listener is self-service software for ingesting and distributing fast-moving data streams, and can feed data to applications in Hadoop or SQL databases. Aster Analytics, meanwhile, contains 100 business-ready analytics techniques for seven industry verticals, and is designed to query Graph, R, and MapReduce with just one SQL query. The system, which has a visual interface, is designed so that non-data scientists can perform advanced analytics.
At left, data feeds from various sources (industrial IoT, web clickstreams) are ingested by Listener then written to target DBs and analytics platforms. Source: Teradata
Teradata IoT: Stream Correlation and Machine Learning
The new software enables predictive analytics for IoT applications with multiple steams of data, Graham said. For example, with construction equipment, perhaps 20 machines out of 50 will give some indication through sensors they are about to fail, but the machine itself never gives out an alert. Or in semiconductor manufacturing, sensors might say everything is working fine but on the production line, wafers are failing.
To prevent such problems, it’s crucial to develop a model through machine learning, as “there’s no way you can get a programmer to code for 40 different scenarios” of equipment failure, Graham said. “And that’s where massively parallel machines like Aster come in — the more data you see, the more accurate the model gets. If you don’t have at least a few terabytes, you’re not going to get a decent model.”
Through machine learning, unseen data correlations can be seen, whether in the industrial IoT, in e-commerce, or in finance. For example, suppose a business is looking at between five and 20 different streams of data, each from a different machine sensor. Or an e-commerce business might be monitoring clickstreams, a news feed, and weather data.
“You have to look for a pattern that says when A, B, and C happens, within this period of time, then D happens,” Graham said. That’s your correlation.”
In the world of customer experience, Graham cited an example of a banking customer closing an account. Without necessary data, a bank might think the customer’s first point of contact was the account closure. But the customer’s real journey entailed visiting a branch to reverse an overdraft charge, later making a phone call to the call center, and visiting the bank’s website.
At present, Teradata Listener, which can be run in the cloud or on-premise, is comprised of RESTFul APIs and Apache open-source technologies — Kafka, ElasticSearch, and Mesos — but the company is “starting to add a lot of connectors to it,” Graham said, such as to enable monitoring of server logs or IoT devices on the edge.
“That will allow us to stream the data from the edge nodes back to the data warehouse or the data lake,” he said.