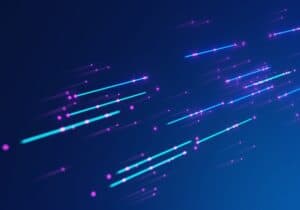
Robotic data automation paves the way to the next generation of data integration architecture, which is data fabric and data mesh.
Every company needs to become a data-driven enterprise, powered by AI and analytics. However, achieving this requires a robust intelligent data supply chain, in which data is piped from its sources in raw form to actionable information. The tools and technologies that enable this are available, but enterprises need to reorganize and rebuild themselves around data supply chains.
That’s the word from the recent Robotic Data Automation Fabric and AIOps event, hosted by CloudFabrix, which explored the requirements for a highly functioning data supply chain – or pipeline – to support emerging AI and analytics initiatives. (I had the opportunity to participate in the event as a keynote speaker.) “We’re using user data to start making better decisions, such as around where to allocate resources, where to allocate time and money and investments, how to optimize business,” said Sean McDermott, CEO of Windward Consulting Group and another keynoter.
See also: Major Integration Challenges of Today and How to Overcome Them
The key to achieving a high-functioning data-driven enterprise is through the joining of two emerging practices: AIOps is the automated and intelligent support of data management, and robotic data automation (RDA) is the automation of the data supply chain, which supports AIOps. While RDA has the potential to underpin data flowing into AI intended for all forms and functions, its initial use cases involve IT optimization, with a focus on well-functioning AIOps setting up the next challenge on the path to fully automated IT.
“AIOps is as transformational to IT operations,” said McDermott. “We want something that’s more intelligent when we deal with systems. We don’t want to be manually inputting data all the time. One customer I spoke to was manually updating seven different CRM systems. It doesn’t make any sense to do that. We’re putting machine learning and AI in front of IT operations. The conversations we’ve been having for the last 20 years include ‘how do I manage alerts? How do I do root-cause analysis? How do I do correlation? How do I manage all this data?’ The difference now is that we have massive amounts of data, and computing is so much more complex than it was before. The value proposition of AIOps is it makes digital transformation possible. Covid accelerated a lot of things, and we were moving into the direction of even more mobility and more digitalization of businesses.“
AIOps and RDA have become critical as companies adopt SaaS, IoT, and other technologies, “the landscape of infrastructure has become complicated,” said Meenakshi Srinivasan, partner of the Global DevSecOps Practice at IBM Consulting, and also a speaker at the conference. “The challenge is to increase manageability.”
Ultimately, AIOps and RDA pave the way to the next generation of data integration architecture, which is data fabric and data mesh. “A data fabric architecture sews together historical and current data across data silos, hopefully giving you a uniform and unified business view of the data,” said McDermott. Data fabric “breaks the data down into reusable components. Data fabric is commonly associated with digital transformation efforts since there’s an assumption that the data fabric architecture will actually reduce costs. Organizations often have issues in governing their data sources and trying to generate value from AI.”
Data mesh, McDermott continued, “is a decentralized architecture, where data architecture is considered with a high degree of democratization, and also scalability in mind as well. It’s really intended to address the common failure modes of traditional centralized data lakes or data platform architecture.”
The transition to data-driven enterprise “is not going to happen overnight just because you have put a few of the tools in place,” said Srinivasan. “Once we get the foundation and automation layer right and start collecting the data. Defining the right sets of data, as well as the quality of the data – plays a major role in AIOps. If you don’t have the right set of data, this journey is going to take longer.”