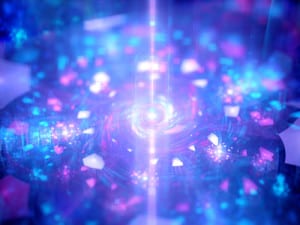
The necessary compute power to run AI models is increasing by a factor of two every three months.
A new method for training AI algorithms may reduce the computational demands, providing underfunded academic researchers with an economical way to train models.
A team from the University of Southern California (USC), in partnership with Intel Labs, presented the method at the 2020 International Conference on Machine Learning.
SEE ALSO: Digital Twin Market to See Tenfold Growth in Next 5 Years
Instead of running all three main computation jobs (simulating environment, deciding next move based on rules, using results to update the model) at once, the method, called Sample Factory, divides them into separate parts, allocating resources as necessary.
Through this, the team has recorded speeds far greater, while using less computational resources.
“From my experience, a lot of researchers don’t have access to cutting-edge, fancy hardware,” said lead author and graduate student at USC, Aleksei Petrenko, to Spectrum IEEE. “We realized that just by rethinking in terms of maximizing the hardware utilization you can actually approach the performance you will usually squeeze out of a big cluster even on a single workstation.”
The team ran the method against several open-sourced algorithms, from DeepMind and Google brain, in the first-person shooter Doom. It was able to process about 140,000 frames per second with a single machine, around 15 percent better than the industry’s best.
The necessary compute power to run AI models is increasing by a factor of two every three months, according to OpenAI. This has led to more academic and enterprise research to find ways to reduce the cost and energy use, while maintaining adequate performance.
But, even with these advancements, it may still be necessary for academia to be able to tap into the largest supercomputers and data centers. A national research cloud has been proposed, as a potential way to alleviate the costs and level the playing field.