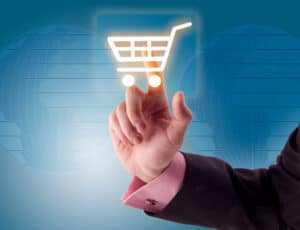
The complexity of retail is begging for more investment in AI/ML to determine how much it can improve the way companies interact with customers and each other.
It’s a confounding question. How do you push the boundaries of reach and scale that the online world can offer while also offering the tailored, personalized experience every individual wants? How, in a sea of millions with nearly infinite combinations of channels, do you create a relationship with a single customer that resonates enough to cut through the noise and foster lifetime loyalty? Artificial intelligence and machine learning (AI/ML) may have the answer.
Retail is the ultimate testing ground for what artificial intelligence can do. Sure, plenty of companies are leveraging solutions they call AI but aren’t. Automation strategies make the top of that list. But retail provides a reason and a test case for machines that think like humans and collaborate with them. This is what’s happening.
Online retail is a behemoth. On the customer side, you have a bewildering combination of channels that customers use to find a retail company, interact with it, and then spread the word. Customers want to feel like a company knows their individual preferences and can remember when and why they’ve interacted before, and all this with no wait time (preferably in their language of choice.) We want what we want, and we’ll change companies to get it.
On the other side, the back office of online retail companies includes an equally as complex and bewildering global supply chain with its own set of rules and potential pitfalls. It requires a highly deft hand to predict what products consumers will want and when and then pivot to other solutions if the back end doesn’t deliver. The edge here, of course, is data.
But it isn’t just gathering data. Companies have been doing that for years and still getting inventory wrong. Even two years ago, well into the digital transformation era, consumers still couldn’t get toilet paper onto shelves. Earlier this year, one weak link made feeding babies in the United States a national crisis. Retailers have plenty of data but haven’t been able to turn it into actionable insights consistently.
See also: How Online Retailers Use AR to Enhance Profits
Companies are adopting AI in response to the challenge
Artificial intelligence solutions are the missing piece in this data puzzle. Even five years ago, very few online retailers integrated true artificial intelligence into their daily operations, customer-facing or otherwise. That number is undoubtedly higher now, but still, very few companies see the full potential of their AI deployments.
Some of the challenge has been a lack of access to the right tools and solutions in an untested field. Back in 2018, McKinsey predicted that retail spending on AI solutions would reach $8 billion by 2024. That number seems conservative now. Companies are realizing the power that AI/ML solutions can unlock in their pursuit of previously abandoned initiatives such as Customer360 and full operations transparency.
Another McKinsey survey estimates that AI integrations will bring an annual value of $400 billion to $800 billion globally. Companies that recognize this value stand to gain a better bottom line, an increased market share, and even better customer perceptions.
For example, traditional companies such as Walmart have launched projects like the Intelligent Retail Lab to put AI to the test in real-world situations. Test stores allow customers to interact with intelligent solutions such as intelligent shelving and interactive displays. Their retail incubator project provides a truly remarkable customer experience while also helping Walmart collect challenging data from a physical location. By extending AI into the physical store, Walmart can personalize and predict even better than simply gathering online data and keeping it in storage.
Top challenges companies like Walmart face with their expansion and online life—based on an eBook released by AI/ML company Daitaku—include:
- Improving search and product discovery to reduce customer frustration and increase on-site conversions.
- Create dynamic pricing strategies that optimize revenue while preventing stagnant inventory and considering other unforeseen disruptions.
- Optimizing stock, improving inventory predictions, and detecting anomalies related to inventory loss.
- Better leveraging data to understand insights in real-time and acting proactively to create better customer experiences and smooth disruptions on the back end.
AI-first companies are emerging to fill gaps
Walmart is a massive retailer with a lot of capital to invest in new tech and retail locations to explore their own in-house solutions. What can small to medium-sized companies do without that type of reach? IDC recently highlighted four AI-first companies emerging in retail to fill those gaps.
- AiFi: Companies that want to collect customer data at physical locations to integrate with online sites can leverage AiFi’s computer vision and multi-level AI. These solutions use human biometric tracking—anonymized movement data—to explore human activity and pattern recognition to identify how customers interact with products on shelves.
- Birdzi: Companies implementing AI into marketing campaigns usually reduce unnecessary marketing spending and get better ROI. Birdzi offers a platform for personalization that can scale to millions of customers.
- Eversight: Pricing is a difficult component of new retail. Setting the price too low means losing revenue but too high means losing revenue as well. Eversight offers a dynamic price optimization tool that provides a complete picture of base price value through continual testing. The tool becomes more accurate by learning over time through AI/ML algorithms optimized for this challenge.
- Lily AI: Companies struggle to provide customers with relevant and valuable inputs when interacting with products online. Lily AI converts visual product images into data. This allows companies to improve search functions and recommendations, gather more accurate data about what customers are doing and improve revenue by transforming product discovery. Plus, it automates the process to help relieve the burden on limited teams.
Retail can be a proving ground for AI/ML
The complexity of retail is begging for more investment in AI to determine how much it can improve the way companies interact with customers and each other. This use case goes far beyond the limited deployments many companies have leveraged in the past and into full operational integration. No matter what happens, it’s going to change how we see the industry.