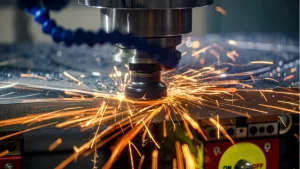
Researchers have utilized AI as an alternative to physics-based modeling simulations to design and synthesize advanced materials.
The formulation of advanced materials which meet the technological and environmental challenges of today’s world is becoming increasingly difficult to achieve, due to the complexity involved in the development, processing, and application of the material.
Traditionally, advanced materials are developed through physical-based modeling simulations. Researchers from the Max-Planck-Institut für Eisenforschung (MPIE) in Düsseldorf, Germany have embedded artificial intelligence into these simulations, with the aim of utilizing AI’s extensive computational capabilities to aide in the discovery and development of advanced materials.
SEE ALSO: Supercharge Your Database with Language AI
“Our means of designing new materials rely today exclusively on physics-based simulations and experiments,” said professor Dierk Raabe, director at MPIE and co-author of the publication. “This approach can experience certain limits when it comes to the quantitative prediction of high-dimensional phase equilibria and particularly to the resulting non-equilibrium microstructures and properties. Moreover, many microstructure and property-related models use simplified approximations and rely on a large number of variables. However, the question remains if and how these degrees of freedom are still capable of covering the material’s complexity.”
In comparison to physics-based modeling simulations, descriptor-based modeling with artificial intelligence can reduce the cost of predicting material sustainability and applicability, while also enabling the researchers to test more materials at a faster rate through automated development.
“AI is capable of automatically extracting thermodynamic and microstructural features from large data sets obtained from electronic, atomistic and continuum simulations with high predictive power,” said professor Jörg Neugebauer, director at MPIE and co-author of the publication.
As AI works best with very large datasets, researchers found it difficult in testing for the AI modeling simulation to reach its maximum potential. One way around this, according to the MPIE professors, would be to initially start with a small subset of labeled data, which could then be processed by the AI and fed into a high-quality dataset. The machine learning model runs the same active learning cycle again and again, until it is able to recognize to a high degree of accuracy materials, composition, synthesis, and processing.
High-quality data remains the most important factor in the functionality and accuracy of machine learning models, and for specialist development such as advanced materials, industry titans and AI-leaders may need to create an open-source database for the advancement of the entire industry. This could be similar to the protein structure database which DeepMind, a subsidiary of Alphabet, created and published in 2022, with over 200 million protein structures catalogued.
That database has already been utilized by several academic and research labs, as a way to speed up the development of drugs. The University of Toronto, alongside Insilico Medicine, reduced the amount of time to design and synthesize a drug for primary liver cancer from several months to 30 days, by using the AlphaFold database and machine learning algorithms.
Healthcare and medical research is seeing more investment from AI-leaders than advanced materials and chemicals however, so it is less likely a leading-edge AI developer such as DeepMind, OpenAI or Google would invest time into that industry. It would also have less moral obligation to open source the dataset, in comparison to medical research.