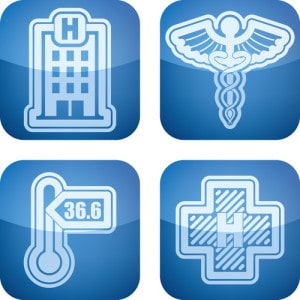
Amara Health Analytics is a provider of predictive clinical analytics software driven by a combination of machine learning, natural language processing, semantic analysis and real-time, physiological signal processing. This is how Amara Health solved their problem with struggling with the wide array of structured and unstructured data it had to manage.
Industry:
Healthcare
Location:
San Diego, Calif.
Business Opportunity or Challenge Encountered
To gain the ability to manage the wide array of structured and unstructured data Amara Health has to manage was a major step for the company in enabling early detection and reducing the death toll from sepsis, an inflammation of the body that occurs in response to infection.
Amara Health focuses on providing real-time predictive analytics to support clinicians in the early detection of critical disease states, particularly sepsis, which takes up to 20 million lives globally. Early detection enables rapid response, deriving better patient outcomes and lower healthcare costs.
Steve Nathan, CEO of Amana Health Analytics, and Christopher Rosin, Ph.D., a lead investigator at Amana, undertook the challenge of battling sepsis with real-time analytics data. “We stepped back and realized that, for a complex disease state like sepsis, there’s a lot of subjectivity and ambiguity for a clinician in analyzing those clinical variables,” Nathan explained in an interview in Datanami.
Watching the vital signs coming off bedside machines isn’t enough, according to Nathan. “You’ve got to look at all of the available the data because you might find a signal in an unexpected place or a combination of places,” he continued.
In addition to dynamic data, information received by Amara’s software varies in format including structured (such as medical codes and other numeric values), unstructured clinician narrative (such as doctor’s notes, operative reports and discharge summaries) and real-time, physiologic signals from patient monitoring devices. The team at Amara Health had to manage large volumes of dynamic structured and unstructured data.
Due to the dynamic nature of data being ingested, it was imperative that the system be agile and able to ingest new data sources unknown at the time of designing the system. “Input data comes from a wide variety of centers and is wildly heterogeneous. Issues range from proprietary health IT systems and incompatible usage of available standards like HL7, to wide variations in clinical language used by physicians and nurses in their notes, to varying patterns of diagnostic testing by different providers,” Nathan told ODBMS Industry Watch.
“Much of our system is dedicated to producing a standardized timeline for each patient in which every variable has a consistent interpretation, no matter where it originally came from,” he continued. “We then use these patient timelines as input for machine learning. And there are some unique challenges there in developing predictive models that are appropriate for use in real-time decision support.”
Amara required a database with a flexible data model, capable of change in real-time. In addition, although much of the information received was in Health Level 7 (HL7) format, a key requirement was being able to handle custom formats hospitals may have. Another critical component in Amara’s search for the ideal database technology infrastructure was enterprise-grade, search over text and the ability to query information.
Amara also needed to show that its alerts were having a significant impact on the care of patients. It is imperative to show over a period of time that the patients that get alerts (and, therefore, receive treatment sooner) have better outcomes.
How This Business Opportunity or Challenge was Met
Traditional databases could not cut it for the large volumes and varieties of real-time data that forms the basis of Amana Health’s solution. The system needed to ingest real-time healthcare data coming from new and varying sources in a variety for formats. Amara Health chose DataStax Enterprise to support Apache Cassandra (considered by many to be optimal for real-time data) plus enterprise capabilities needed in production such as search, query, round-the-clock support and enterprise security.
The Amara solution is a hybrid, on-premises and software-as-a-service (SaaS) solution. In the local model, Amara delivers a local install, with replication. Leveraging Cassandra’s automatic replication and always-on benefits, Amara designed its software to be similar to Cassandra’s architecture in that it includes multiple nodes at which each node fails over to the next node, so rolling upgrades can be performed without downtime. Amara’s SaaS model has geographically distributed data centers, ensuring 100 percent uptime to accommodate varying data sources.
Amara now ingests all of its incoming data into a variety of column families including a patient timeline, time-series in nature. All incoming data is organized into the patient timeline in chronological order, giving users a holistic picture of the patient’s history from the moment they walked into the hospital, until they leave and afterward.
Amara also generates a report column family for analysis. The solution is a combination of natural language processing, machine learning and rules-based analytics so the team has built up a large set of fairly complex rules that deduce the state of the patients based upon the data it’s getting and then identifying risks to patients’ health. Amara’s software is looking for an accumulation of evidence that indicates degradation in the patient’s state, so the system has complex rules.
Clinicians receive text message alerts from Amara’s system on their smartphone or tablet that direct their attention to the at-risk patient. The messages include key clinical variables to provide context for the alerts. Amara’s software connects with multiple hospital data sources and analyzes data streams that are very real-time in nature.
Early detection and treatment are essential to improve sepsis outcomes and costs—every hour matters. But successful early detection is complex and costly, requiring a high level of expert clinician attention. Clinical Vigilance for Sepsis software automatically monitors all patients all of the time, issuing alerts that bring immediate attention to at-risk patients.
Measurable/Quantifiable and “Soft” Benefits from This Initiative
The configuration provides Amara the flexibility to create and modify their underlying data model in the live system. If Amara gets a new type of data, the Amara team can introduce that new information into the live system without having to re-install it or modify the code. This enables users to get immediate insight on new information for analysis and reporting.
Amana calculates that this means a savings of up to $2 million for a typical 300-bed hospital. In addition, the system helps alleviate “alert fatigue,” which may result from too many false positives. In his ODBMS interview, Nathan pointed out that “many companies have attempted to do a sepsis alerting system but almost all of them have suffered from high false positives. What we do both with natural language processing and machine learning algorithms is look at the entire context of the patient before the alert is sent out. Our specificity—the accuracy of the system across all adult patients—is about 99 percent.”
Sources: DataStax, Amana Health Analytics, Datanami, ODBMS Industry Watch
So many articles, so little time. Luckily, our content is edited for easy web reading! Read more:
Research from Gartner: Real-Time Analytics with the Internet of Things
From the Center to the Edge: The IoT Decentralizes Computing
Becoming an ‘Always On’ Smart Business
Urgency of Present and Past in IoT Analytics
Liked this article? Share it with your colleagues using the links below!