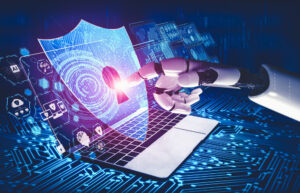
RAG-powered fraud detection systems offer unparalleled adaptability, accuracy, and transparency, equipping businesses with the tools needed to safeguard their platforms. By leveraging historical fraud intelligence and generative AI, RAG ensures that e-commerce platforms stay one step ahead of fraudsters, protecting both businesses and consumers in an increasingly digital economy.
E-commerce fraud is evolving at an unprecedented pace, with fraudsters leveraging sophisticated techniques that easily bypass traditional security measures. Conventional fraud detection systems, relying on static rule-based models and even some machine learning approaches, struggle to keep up with these dynamic threats. This has led to a pressing need for more adaptive and intelligent solutions. Retrieval-Augmented Generation (RAG) offers a promising alternative, integrating historical fraud patterns, transactional metadata, and real-time contextual understanding to enhance fraud detection accuracy and reduce false positives.
The Role of AI in Fraud Detection
Artificial intelligence has already transformed fraud detection by identifying patterns, anomalies, and suspicious behaviors across vast datasets. Traditional machine learning models, however, often operate within fixed feature sets, making them vulnerable to new fraud tactics. Deep learning models improve accuracy but lack interpretability, making them difficult for financial analysts and security teams to trust. RAG bridges this gap by providing real-time contextual retrieval and generative reasoning, enhancing both accuracy and explainability.
See also: Key Lessons for Building Effective RAG Systems
Why RAG for Fraud Detection?
RAG combines the strengths of retrieval-based AI and generative models to create a system that is both adaptable and explainable. Unlike traditional fraud detection models, which rely solely on predefined features, RAG dynamically retrieves past fraud cases, metadata, and external intelligence sources, enriching decision-making in real time.
Key Benefits of RAG in Fraud Detection
- Context-Aware Decisions: The model retrieves past similar fraud incidents and uses that context to evaluate ongoing transactions.
- Improved Accuracy: By integrating structured and unstructured data, RAG significantly reduces both false positives and false negatives.
- Explainability: Traditional black-box AI models lack transparency, but RAG provides a clear audit trail of why a decision was made.
- Scalability: RAG can process vast amounts of transactional data in real time, making it ideal for large-scale e-commerce platforms.
Architecture of a RAG-Powered Fraud Detection System
A RAG-based fraud detection system consists of the following key components:
- Data Ingestion Layer: Collects transactional metadata, past fraud cases, user behavior patterns, and external risk intelligence.
- Retrieval Engine: Queries historical fraud cases, merchant records, and anomalies to provide relevant context to the model.
- LLM-Based Decision Layer: A fine-tuned LLM processes retrieved insights, generating fraud likelihood scores and risk explanations.
- Feedback Mechanism: Continually refines the fraud detection system using human feedback and reinforcement learning.
Real-world Applications and Case Studies
Several financial institutions and e-commerce platforms are exploring RAG-based fraud detection to address pressing security challenges:
- Credit Card Fraud Prevention: By retrieving past fraud patterns and transaction similarities, banks can identify fraudulent purchases in real time.
- Account Takeover Detection: Detects unusual login behaviors based on previous suspicious activities, alerting security teams before fraud occurs.
- Fake Reviews & Promotion Abuse: E-commerce platforms use RAG to detect patterns in review manipulation and discount abuse, reducing financial losses.
Challenges and Ethical Considerations
While RAG presents a groundbreaking approach, challenges remain:
- Data Privacy Risks: Fraud detection requires handling sensitive financial data, making secure data governance critical.
- Bias in AI Models: Training on skewed datasets can lead to unintended biases, potentially flagging legitimate users as fraudsters.
- Computational Costs: RAG models require high computational power, necessitating efficient deployment strategies for large-scale use.
The Future of RAG in Fraud Prevention
Retrieval-Augmented Generation is poised to become a cornerstone technology in fraud prevention, continuously evolving alongside emerging threats. Future developments may include real-time adaptive learning, integration with blockchain for immutable transaction tracking, and multi-modal fraud detection models combining voice, text, and biometric data.
Conclusion
Fraud detection in e-commerce is at a turning point. Traditional methods are no longer sufficient against rapidly evolving cyber threats. RAG-powered fraud detection systems offer unparalleled adaptability, accuracy, and transparency, equipping businesses with the tools needed to safeguard their platforms. By leveraging historical fraud intelligence and generative AI, RAG ensures that e-commerce platforms stay one step ahead of fraudsters, protecting both businesses and consumers in an increasingly digital economy.