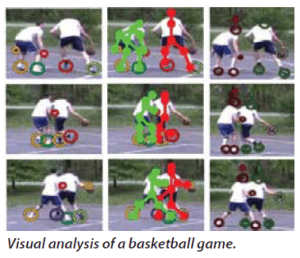
The camera is the primary technology we use to observe our environment and record real-life events. Whether for recreation, information, business, academic or security purposes, people are increasing their use of cameras – and the information the cameras provide – at a rapid pace. Processing this data is inefficient and time-consuming due to its volume and volatility. Therefore, new ways of processing camera-sensed data are needed in order to recognize and analyze events of interest.
To learn more about what types of technologies are being developed today to make cameras more effective and valuable in all of their applications, RT Insights contributor George Paliouras, of NCSR DEMOKRITOS in Greece, sat down with Professor Larry S. Davis, Director of the Center for Automation Research at the University of Maryland. Professor Davis’ lab is at the forefront of this exciting and challenging research area. Here, he shares some of the exciting work being conducted there.
Paliouras: What is video analysis used for?
Davis: There is a wide variety of applications of video analysis. The most common is monitoring areas for events that suggest security and safety violations. However, the technology used in these systems for locating and tracking people or analyzing their movements and activities, is also applied to analyzing sports videos or monitoring animal experiments instead of giving this boring task to people.
Paliouras: Can you provide some examples?
Davis: Most currently deployed systems that are completely automatic are used for perimeter and facility security. So, along the U.S./Mexican border, parts of the border are patrolled with manned cameras while other parts are monitored with cameras on unmanned air vehicles. Most systems are still used for forensics: after-the-fact gathering of evidence after a security or safety violation has occurred.
Paliouras: What types of event can be sensed through video surveillance?
Davis: Most surveillance systems on the market today can reliably detect only fairly simple events. Examples include a person being in a restricted area (e.g., a border zone), someone entering a room in an unauthorized way (e.g., through an exit door) or a lab rat making its way through a maze.
In research settings, however, we deal with more complex events such as analyzing crowd behavior (like the annual Hajj in Jeddah where dense crowding of pilgrims can result in injuries) or sports games where broadcasters are supported by automatic annotation and commentaries. A major challenge in deploying these more advanced methods is their high false alarm rate, which means they sometimes detect events that did not happen. False alarms reduce people’s confidence in systems and can lead to inappropriate, automatic response in some cases.
Paliouras: What are the technical difficulties involved in implementing video surveillance?
Davis: Most surveillance efforts require integrating information from many cameras. That’s because the physical area under surveillance is too large to be monitored by one camera. This creates a number of challenges. Often, companies have to archive the video from all cameras for a period of time. This means that the cameras have to be connected over a high-speed network to a central storage facility or they need to have local recording and storing capabilities. Some cameras are “smart” and can monitor incoming video for specific events of interest such as a security violation and can then send data to the central processing server only when such an event occurs.
The operator interface for large-scale surveillance is also very important. Typical control rooms have people monitoring a bank of screens, with each screen showing the video feed from a single camera. Several studies have shown that this is neither efficient nor effective as people get tired and bored. There are great opportunities for amplifying the effectiveness of human security personnel by intelligent detection or prediction of events and directing the attention of security personnel to them.
Paliouras: What is your approach to detecting interesting events?
Davis: In our lab at the University of Maryland, we are specifically interested in activities involving multiple people. On the one hand, there are very difficult technical problems involved when processing video of people interacting with each other or with objects. When people are close to each other, there may be significant interpersonal occlusion, meaning that one body partially blocks the view of another from the camera’s point of view. To make things more difficult, person-to-person interactions are often complex so that their recognition in videos requires fairly high-level reasoning. This requires some way to encode the common sense reasoning that people employ all the time to understand the activities of others.
Paliouras: How can computer vision systems use common sense knowledge?
Davis: Our own approach at the University of Maryland involves a blend of artificial intelligence and video processing. For example, consider the problem of monitoring a parking lot by trying to keep track of the vehicles going in and out. People can do this effortlessly although it is tedious work. Computer vision algorithms that detect and track people and vehicles get confused.
For example, when a person moves between two parked vehicles and disappears, a computer vision algorithm must determine which vehicle the person entered. Common sense can help: If one of the two vehicles leaves after the person “disappears” between them, then the person probably entered the vehicle that left. We would be especially certain of this if the person was on the driver’s side of the vehicle and the system believed that the vehicle was previously empty. All of this common sense knowledge can be encoded in logic but traditional logic has to be extended to reason with the aid of probability. This is the current focus of our research: Identifying the relevant common sense knowledge, encoding it logically, and working to improve the way computers use it to visually analyze human interactions.
Paliouras: What developments do you see coming in the future?
Davis: Future research on visual surveillance will emphasize the modeling and recognition of events that evolve over much longer periods of time than current surveillance systems can handle. For example, it would be useful to model the formation of crowds to anticipate potentially dangerous situations or create summaries of long videos (such as sports games) for browsing. Such events will be composed of what we currently consider to be complex events and will capture the way in which they evolve over time.