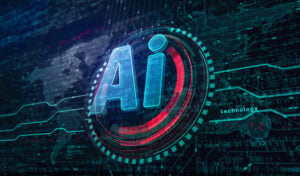
It’s time to move from ambitious AI pilots to sustainable execution. To do that, organizations must build trust in data and AI, develop adaptive data ecosystems, and embed AI into company culture through behavioral change initiatives.
In today’s data-driven world, most companies have high ambitions for their data and analytics projects, as well as AI initiatives. However, as these efforts grow and take on more important roles, a common issue many companies face is the challenges they encounter when scaling such programs.
Many talks at this week’s Gartner Data & Analytics Summit raised this issue. That was particularly the case in the keynote address, “Scale Data and Analytics on Your AI Journeys,” delivered by Gareth Herschel, VP analyst at Gartner, and Carlie Idoine, Vice President Analyst for Data, Analytics & AI at Gartner. The high-level point in their talk was that as companies scale their efforts, they must seek consistent execution across the entire organization. Here are some of the main points they raised:
The Journey to Business Outcomes: Trust as the Foundation
One of the biggest challenges organizations face is demonstrating the value of data, analytics, and AI initiatives. Many struggle with trust…not just in AI but in the very data that fuels it. Data quality, lineage, bias, and complexity are common concerns. The speakers noted that Gartner’s research shows that data availability and quality remain the top obstacles in AI implementation.
To address this, organizations must shift the conversation from asking, “Can we trust AI?” to “How can we make AI more trustworthy?” This requires robust data governance and the implementation of trust models—systems that assess and rate data trustworthiness based on value, lineage, and risk.
A key example highlighted in the keynote came from Alda Mizaku, Chief Data and Analytics Officer at Children’s National Hospital in Washington, D.C. She developed a system that rates data assets, helping doctors and nurses quickly assess trust levels. This led to a significant reduction in medical errors and improved patient care—an illustration of real-world value creation.
Furthermore, governance should not be seen as a roadblock but as an enabler. Thierry Martin of Toyota Motor Europe adopted a “freedom in a box” approach, where governance provides boundaries rather than restrictions. This fosters innovation while ensuring compliance and security.
See also: Top 5 Challenges When Integrating Generative AI
The Journey to Capabilities: Building an Adaptive Data Ecosystem
A key takeaway from the keynote was that AI is not a one-time implementation; it requires an adaptive data ecosystem. Companies must move from rigid data infrastructures to flexible, modular architectures that can accommodate rapid technological advancements.
Organizations also need to shift their focus from one-time data use cases to reusable data products. Only one in five companies consistently reuse their data for AI use cases. A structured approach—documenting and cataloging data products, implementing service-level agreements, and creating internal data marketplaces—can help scale AI initiatives more effectively.
Tom Black, VP of Data and Analytics at Molex, demonstrated this by identifying repeated data access requests. By turning these into standardized data products, Molex accelerated innovation while reducing costs.
Additionally, active metadata—data about data that can drive automated actions—is critical in governing the data ecosystem. China Merchants Bank, for example, developed a system to classify and update trust levels dynamically, ensuring that AI models operate with high-quality, relevant data.
Finally, artificial intelligence is shifting from supporting analytics to executing autonomous decisions. AI agents, designed to be perceptive, adaptive, and decisive, will increasingly handle complex tasks across multiple environments. These agents will use active metadata to navigate AI-ready data ecosystems, making real-time decisions and optimizing business processes.
The Journey to Behavioral Change: Embedding AI into Organizational Culture
While technology plays a crucial role, human behavior is the final frontier in AI adoption. Organizations must recognize that AI transformation isn’t just about tools and systems—it’s about people. Change management is often an afterthought, but it should be a strategic priority.
At Froedtert & Medical College of Wisconsin, the data and analytics team identified four stages of engagement: resistant, curious, assimilating, and independent. By tailoring training and support based on these stages, they increased the adoption and effectiveness of data-driven decision-making.
A common barrier to AI adoption is company culture. Many organizations say their culture isn’t data-driven, but Gartner emphasizes that culture is not an abstract concept—it’s defined by day-to-day behaviors. Shifting culture requires establishing repeatable habits that reinforce the value of data-driven decisions.
Organizations should also rethink roles and skill development. AI will automate many existing tasks, but it will also create new job functions, requiring a focus on continuous learning. Applied ethics, bias detection, problem-solving, and critical thinking will become as important as machine learning and programming skills.
Gartner also highlighted the evolving role of Chief Data and Analytics Officers (CDAOs). As artificial intelligence becomes a competitive differentiator, CDAOs must assert their influence within the C-suite, demonstrating how data-driven strategies drive AI success.
The Path Forward: From Experimentation to Execution
The keynote concluded with a clear call to action: It’s time to move from ambitious AI pilots to sustainable execution. Organizations must build trust in data and AI, develop adaptive data ecosystems, and embed AI into company culture through behavioral change initiatives.
By focusing on trust models, reusable data products, active metadata, and cross-functional collaboration, companies can scale artificial intelligence initiatives effectively. The organizations that thrive will not be those with the most dashboards or the latest AI tools but those that embed trust, governance, and adaptability into every stage of their AI journey.
Ultimately, success in AI is not about who has the best technology; it’s about who can execute consistently, adapt continuously, and bring people along on the journey.
Salvatore Salamone contributed to this article.