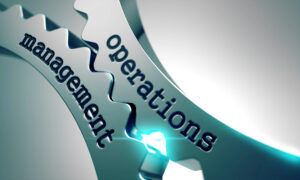
For those industrial organizations in the early stages of a digital transformation, condition-based maintenance represents a significant step forward. It can help enhance operational efficiency, reduce costs, and improve safety.
In the demanding world of industrial operations, downtime is the enemy of productivity and profitability. Whether in manufacturing, oil and gas, chemical processing, or other industries, the need to maintain equipment reliability while managing costs is paramount. Many have looked to digital transformation to help. The main idea is that data from a plethora of sensors and IoT devices can be leveraged to improve uptime.
For some, the jump from preventive maintenance to predictive maintenance that relies on real-time analysis and predictive algorithms is too great a leap. There are data management issues, skills gaps in using predictive technology, and more. As such, some industrial organizations are taking an intermediate step, moving to a condition-based maintenance (CBM) strategy.
What Is Condition-Based Maintenance?
Condition-based maintenance is a proactive approach that monitors the actual condition of equipment to determine when maintenance is needed. Unlike traditional methods, which rely on fixed schedules or predictive models, it focuses on real-time monitoring and analysis of parameters such as temperature, vibration, pressure, or oil quality. When an anomaly is detected, maintenance is scheduled, ensuring timely intervention before a failure occurs.
Condition-based maintenance’s core principle is simple: repair or replace components only when there are signs of deterioration. This minimizes unnecessary maintenance while ensuring that equipment operates efficiently, safely, and reliably.
Adopting a CBM strategy offers numerous advantages, including:
Reduced Downtime: By addressing issues as they arise, CBM prevents unexpected equipment failures that can halt production and lead to significant financial losses.
Cost Savings: CBM minimizes unnecessary maintenance activities, reducing labor and material costs. It also extends the lifespan of equipment by preventing damage caused by prolonged undetected issues.
Enhanced Safety: Detecting and addressing equipment issues early reduces the risk of accidents and ensures compliance with safety regulations.
Improved Asset Utilization: With CBM, equipment operates at optimal efficiency, maximizing throughput and reducing energy consumption.
Data-Driven Insights: CBM generates valuable data that can be used to identify patterns, optimize maintenance schedules, and improve overall operational efficiency.
How CBM Differs from Preventive and Predictive Maintenance
Condition-based maintenance is often compared to preventive and predictive maintenance, but each has distinct characteristics:
- Preventive Maintenance: This approach schedules maintenance at regular intervals based on time or usage—for example, changing machine components every six months. While it reduces the risk of failure, it can lead to unnecessary maintenance if the equipment doesn’t need servicing.
- Predictive Maintenance: Predictive maintenance uses historical and real-time data to predict when a failure might occur. It relies heavily on advanced analytics and machine learning to forecast maintenance needs. While highly effective, it requires substantial investment in data infrastructure and expertise.
- Condition-Based Maintenance: CBM bridges the gap between these two approaches. It doesn’t rely on fixed schedules or predictive algorithms but instead monitors equipment in real time. Maintenance is performed only when specific thresholds or anomalies indicate a problem, offering a more flexible and responsive solution.
Technologies Enabling Condition-Based Maintenance
Implementing condition-based maintenance requires a combination of technologies to collect, process, and act on real-time data. Key components include:
- Sensors and IoT Devices: Sensors are the backbone of CBM, measuring critical parameters like temperature, vibration, pressure, and fluid quality. IoT devices connect these sensors to centralized systems, enabling continuous data collection and transmission.
- Data Acquisition Systems: These systems aggregate data from sensors and ensure it is available for analysis in real time. They form the link between physical equipment and digital systems.
- Edge Computing: In industries with low-latency requirements, edge computing processes data locally, enabling faster decision-making and reducing the reliance on cloud-based solutions.
- Data Analytics and Machine Learning: Analytics platforms analyze sensor data to identify patterns, anomalies, and trends. While CBM doesn’t always require predictive algorithms, machine learning can enhance its effectiveness by improving anomaly detection and identifying subtle warning signs.
- Connectivity and Communication: Wireless technologies such as Wi-Fi, Bluetooth, or cellular networks ensure seamless data transmission, even in remote or challenging environments.
The Final Word on Condition-Based Maintenance
For those industrial organizations in the early stages of a digital transformation, condition-based maintenance represents a significant step. It can help enhance operational efficiency, reduce costs, and improve safety. By leveraging real-time data and advanced technologies, CBM empowers organizations to take a proactive, targeted approach to equipment maintenance.
As industrial operations become increasingly digital, the integration of CBM with predictive analytics, AI, and other innovations will unlock even greater opportunities for efficiency and resilience. For organizations willing to invest in this transformative approach, the rewards are clear: reduced downtime, optimized performance, and a competitive edge in today’s fast-paced industrial landscape.