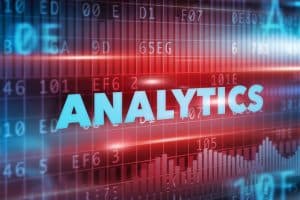
Untethering compute from the cloud allows the broadening of AI’s reach. And it speeds up response time by reducing the lag caused by communicating with distant servers.
Artificial intelligence has a bottleneck problem. It’s based on deep neural networks that can require hundreds of millions to billions of calculations — a processing and energy-intensive undertaking. Then there’s the cost and latency of shuffling data to and from memory to perform these and other analytics computations. Enter Vivienne Sze, associate professor at MIT, known for her role in developing video compression standards still in use today. Now, she is focusing on designing more-efficient deep neural networks to process video, and more-efficient hardware to run AI applications on smartphones, embedded devices, tiny robots, smart homes, and medical devices.
In a recent interview at MIT, she explains why we need low-power AI now. “AI applications are moving to smartphones, tiny robots, and internet-connected appliances and other devices with limited power and processing capabilities. The challenge is that AI has high computing requirements. Applying analytics to sensor and camera data from a self-driving car can consume about 2,500 watts, but the computing budget of a smartphone is just about a single watt.”
Localizing AI on small devices such as smartphones “means that the data processing no longer has to take place in the cloud, on racks of warehouse servers,” Sze says. “Untethering compute from the cloud allows us to broaden AI’s reach. It speeds up response time by reducing the lag caused by communicating with distant servers. This is crucial for interactive applications like autonomous navigation and augmented reality, which need to respond instantaneously to changing conditions. Processing data on the device can also protect medical and other sensitive records. Data can be processed right where they’re collected.”
From a hardware perspective, Sze seeks to “reuse data locally rather than send them off-chip. Storing reused data on-chip makes the process extremely energy-efficient.” On the software side, Sze is designing “pruning” into algorithmic code to remove energy-intensive “weights” from a deep network, along with other adaptations. A potential application she is looking at is eye-movement tracking to help diagnose neurodegenerative disorders that can be done with an ordinary smartphone from patients’ homes, versus the expensive in-office equipment typically required up until now.