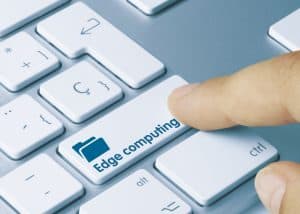
From the simple to the incredibly complex, edge intelligence is an ally for the success of your IoT project.
Digital transformation is sweeping through every industry, prompting organizations to install audio, video, and vibration sensors across their operations. However, given that 30% of IoT projects fail in the proof-of-concept stage, it’s entirely reasonable to be cautious when it comes to investing money in large-scale IoT deployments.
One of the primary causes of IoT failure is organizations are seldom prepared for the tsunami of data all those devices generate. The quantity of data puts a strain on latency and the central compute infrastructure or cloud, not to mention the workforce. The labor that goes into separating the wheat from the chaff – routine maintenance data from actionable insights like imminent machine failure – is daunting for most organizations.
See also: The Edge is Now the Center of the Action
Enter edge computing. Edge computing is poised to become the status quo, involving processing data locally to the point of data creation. Gartner predictsthat by 2025, 75% of enterprise-generated data will be created and processed outside the cloud; today, it’s around 10%. Organizations started implementing edge computing to remedy the latency and bandwidth costs associated with transmitting large amounts of data from centralized data centers to the cloud.
There’s a lot of variety though in edge computing solutions. Many solutions lack a way to make sense of the data collected. OT personnel receive this data and often don’t know what to do with it or how to analyze the data to increase operational efficiencies. Further processing is often required, typically from the cloud. However, intelligent edge computing platforms add fundamental advancements that address these challenges, making robust analytics available without a cloud connection.
Edge Intelligence: For a Smarter, More Agile IoT
Edge intelligence builds on the typical data ingestion capabilities common among edge computing platforms with layers of advanced functions like machine learning (ML) and artificial intelligence (AI.) ML lifts the workload off the cloud and data centers by providing analytics and actionable insights right at the edge. Intelligent solutions derive actionable insights from the streaming data and respond to it through real-time alerts to operators and other enterprise systems. Closed-loop control capabilities between the cloud and the edge are also supported to automate corrective actions on assets or processes immediately.
ML with intelligent edge capabilities reduces compute load by learning in action. It continuously processes high frequencies of data streams and information packets, forming a complete solution right at the edge where you need it most. Here is a three-point approach to data processing at the edge:
- Ingestion and Enrichment: The first stage of edge processing is data ingestion and enrichment. This layer cleans data and prepares it for processing through decoding, filtering, interpolation, and more. It combines and aligns high volumes, varieties, and velocities of streaming video, digital sensor, and asset data. It is the cornerstone that ensures data quality for all additional processing and computing.
- Complex Event Processing (CEP): Intelligence is provided through the CEP by providing pattern detection and real-time analytics to the cleaned, streaming data. Here, actionable insights are derived through rules-based algorithms, and actions can be taken.
- Machine Learning Engine: Executing machine learning models at the edge can be a challenge as edge devices are lower in compute power and memory. A proper CEP provides data pre and post-processing required for the models, so model size, layers, and memory needed for execution is often reduced by 10X or more after being prepared for the edge.
When it comes to edge intelligence, machine learning can solve very complex problems and even provide predictions into health and performance of operations. In certain environments, it’s not an exaggeration to say that advanced analytics and ML at the site-level can save lives.
Edge Intelligence Off-Line
There are many instances when an organization might not want to trust sending a device’s data to the cloud or the internet, and edge intelligence makes it possible to keep the processing power and ML, while losing the need for connectivity to the cloud. Your organization can reap the rewards of advanced, deep data processing without the security risks and costs for bandwidth, processing, and storage.
What benefits can your organization expect to see after making the transition to edge intelligence?
- Massive reduction of data. When analytics move to the edge, there is a massive decrease in the amount of data pushed across the network. This reduces data storage and data-handling costs, while also freeing up IT and OT personnel to work on more complex issues.
- Better real-time insights. By keeping the computing close to the data source, edgified machine learning can detect emerging patterns and enable immediate responses before a glitch becomes a full-scale crisis.
- Predictive maintenance for all. Because an edge-based system can handle all incoming machine data, it can predict maintenance needs across all equipment in the operation, not only substantially slashing the time it takes to plan maintenance activities, but also preventing accidents caused by delayed upkeep.
- Improved yield. Users can increase productivity and reduce downtime by rapidly detecting and addressing suboptimal performance, providing better performance with a 360-degree view of operations.
By transforming the IoT market, edge intelligence makes real-time analysis easier, hence increasing operational efficiency while decreasing the costs incurred in handling and storing data.
An intelligent edge solution can perform many functions that take the manual load off operators and data consumers. Ideally, you need a solution that is not only smart about aggregating and sorting data but also has the capability to detect when an asset or process is malfunctioning.
As you’ve seen, intelligent edge computing, in addition to machine learning capabilities, provides vital, actionable insights in real-time, helping organizations conquer a few fundamental challenges and open the door to advanced analytics. On the fundamental side, they make sure that all your devices are communicating in the IoT network protocol of your choice (ModBus, OPCUA, etc.), while also processing advanced data at the edge, without lagging from latency issues. And, they can improve your decision-making because you’ll always have the most up-to-date data.
From the simple to the incredibly complex, edge intelligence is an ally for the success of your IoT project.