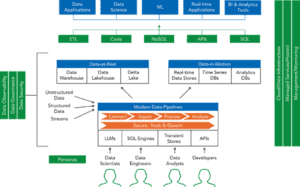
The Data-in-Motion Ecosystem Map shows the various moving parts of your data architecture and how they are all connected with each other.
Over the last decade, I have been an industry observer, a hands-on practitioner, an evangelist, and a CIO/CTO whisperer in the data-in-motion space – a space that I have seen grow, evolve and explode in terms of the number of tools, solutions, and platforms. With over 330 million TBs of data being generated every day in the world, at least 30% of that is real-time streaming data, and it is only increasing exponentially every day. So, the road ahead indicates that we will only have more complexity in this space. This article is the first in a series of many to demystify that complexity and offer some clarity for all the buyers and users in the data-in-motion landscape.
For the uninitiated, “data-in-motion” refers to all real-time data that is continuously streamed into the enterprise from various data sources such as log streams, social streams, telemetry data, IoT devices, sensor data, and so on. Unlike traditional data-at-rest found in various data stores within the enterprise, data-in-motion carries the unique attribute of “data freshness” that is extremely valuable to any enterprise. Data tends to decay over time in terms of its value for making key business decisions. The sooner the data is looked at in the right business context and correlated with the right metrics, the more valuable it becomes to the organization.
So, the key is to not only capture such data in real-time but also to be able to process and analyze it in near real-time in order to gain valuable insights and analytics. This type of real-time intelligence is critical to a business in enhancing customer experiences, boosting operational efficiencies, and managing risks of all types. IDC predicts that “By 2025, real-time intelligence will be leveraged by 90% of G1000 to improve outcomes such as customer experience by using event-streaming technologies.”
See also: Beyond Kafka: Capturing the Data-in-motion Industry Pulse
Many tools, solutions, and platforms have been developed over the last few years to address the growing challenges of volume, velocity, and variety when it comes to data-in-motion. And this trend has only compounded quite a bit over time, and today, there are dozens of tools that address every aspect of the data-in-motion lifecycle. For example, just for data ingestion alone, there are purpose-built tools, open-source options, third-party APIs, traditional means, home-grown microservices, and comprehensive streaming platforms. To the average IT decision-maker, it can become an excruciating task to understand which tool or platform to choose for what purpose, given the score of options they have in the market today.
The Data-in-Motion Ecosystem Map
I want to introduce you to the Data-in-Motion Ecosystem Map – a guide that will not only show the various moving parts of your data architecture but also paint the big picture of how they are all connected with each other. Once you understand this, you can place specific vendor tools and platforms over several of these boxes and know where your gaps are. This should make the buying process easier and help optimize the various tools and platforms currently in use within your organization.
Click to view Data-in-Motion Ecosystem Map
Just to explain the lay of the land here, it all starts with different types of data – unstructured, structured, or streams, coming in from various sources. The way the data gets ingested is through a modern data pipeline. This may be a single tool or a suite of tools that allow native and organic ways to connect with such data sources as well as ingest them immediately and efficiently. Before such data gets pushed into any type of data store, modern data pipelines have the ability to process and analyze real-time data while they are in flight. This enables various personas that require access to such immediate insights to get them through various mechanisms such as SQL, APIs, and even more modern GenAI-type methods.
See also: Do You Need to Process Data “In Motion” to Operate in Real Time?
From here, the data can be pushed into multiple directions depending on what is expected from the data. It can go into long-term data-at-rest stores such as data lakes or data warehouses. Or, it can go into more immediate processing stores such as time-series databases or analytics data stores. This data is further made available to other end-user systems, such as data applications, machine learning models, or BI tools, through a variety of industry-standard methods or protocols for data transfer.
Outside of all this, there are other elements of data that need to be considered in this model, such as infrastructure, data governance, security, data privacy, observability, management, monitoring tools, and more. All of these are critical elements in determining your tool or platform of choice for your data-in-motion needs.
With the intent of keeping it simple in this first article, I have probably over-simplified some of the most advanced concepts of data collection, stream processing, and real-time streaming analytics. But, you can expect a lot more detailed information from us going forward. Joining hands with RTInsights, Stratola will be putting together a series of webinars, articles, and guides to provide you with more clarity and information on the data-in-motion ecosystem. Stay tuned as we start revealing more in the coming weeks.