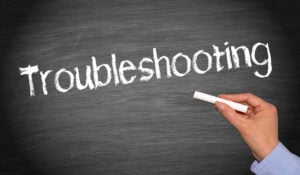
As industrial operations grow more complex, traditional troubleshooting methods are no longer sufficient. Organizations must embrace AI-driven, data-rich solutions to enhance their troubleshooting workflows.
Industrial operations have become increasingly complex and reliant on interdependent processes that all work together harmoniously. Any problem in one or more processes, pieces of equipment, or operational steps can lead to performance degradation or outages, both of which have significant consequences on the system-wide operations and the bottom line.
As such, industrial organizations face mounting pressure to resolve operational issues quickly. Troubleshooting teams must act fast to minimize losses and ensure operational continuity. However, traditional troubleshooting methods—such as predictive maintenance models, manual log reviews, and experience-based problem-solving—are becoming increasingly inadequate.
To address these issues, many industrial organizations will need to leverage the vast amount of data they have about their operations and processes and use advanced analytics and AI to gain greater insights that lead to speedier troubleshooting and problem resolution.
Unfortunately, what many organizations find is that they need help applying the analytics and AI to the data in a suitable manner to get the information their troubleshooting teams need to do their jobs.
The Scope of the Problem
Today’s industrial environments generate an overwhelming volume of data from IoT sensors, industrial control systems, and a variety of other sources. Subject matter experts must sift through massive amounts of real-time and historical data, technical manuals, vendor specifications, and operational records to pinpoint the root cause of a problem. This complexity can lead to delays, increased costs, and unnecessary downtime.
This process needs to be streamlined. Troubleshooting teams need access to the right data at the right time, all in one place. With such access combined with advanced data platforms and AI-powered tools, industrial organizations can enhance troubleshooting workflows, leading to faster problem resolution and better business outcomes.
One might ask, why is this an issue, and why now? Organizations have been using IoT and other data for decades to support predictive maintenance and other efforts that reduce downtime and speed up the resolution of problems. What’s new is a combination of the large volume of data now available plus the need to make troubleshooting decisions faster.
To diagnose a problem effectively, engineers and operators need more than just real-time data—they need context. Historical trends, maintenance logs, past incident reports, and even external resources such as equipment manuals and vendor specifications must be incorporated into the analysis. However, accessing and synthesizing all this information from disparate sources is often time-consuming and inefficient.
Without a streamlined approach, troubleshooting remains reactive, with engineers wasting valuable time searching for relevant data rather than solving the issue at hand.
What’s Needed? A Single Collaborative Workspace
For troubleshooting workflows to be truly effective, industrial teams need a unified digital workspace that consolidates all relevant data sources into a single, easy-to-navigate interface. This workspace should include:
- Real-time sensor data to understand current conditions.
- Historical time-series data to identify patterns and anomalies.
- Asset history and maintenance logs for context on past failures and fixes.
- Technical documentation such as engineering documents, 3D CAD models, vendor manuals, process diagrams, and specifications.
- Collaboration tools to enable cross-team discussions and knowledge sharing.
A key component of this workspace is generative AI (GenAI), which can automate data retrieval and analysis, significantly reducing the time it takes to find relevant information. Rather than manually searching through databases, engineers can ask AI-powered assistants natural language questions and receive immediate, context-rich answers.
For example, if a turbine is experiencing unexpected vibration, a GenAI system can instantly pull in:
- The last 12 months of vibration data to identify anomalies.
- Past maintenance records for that specific turbine.
- Similar failure cases from across the organization.
- Vendor recommendations for troubleshooting vibration issues.
By integrating all of these elements, troubleshooting teams can accelerate problem resolution and make better-informed decisions, minimizing downtime and maximizing operational efficiency.
Working with a Technology Partner
Many industrial organizations find that they lack the time, resources, and, in some cases, internal expertise to build this kind of integrated troubleshooting environment on their own.
The obvious solution is to team with a technology partner that brings both a solution and real-world expertise in using such a solution to support the demanding troubleshooting needs of industrial operations.
Enter Cognite. Cognite offers advanced data and AI-driven solutions designed to empower troubleshooting teams with fast, contextualized insights. Cognite helps in a number of ways, including:
- Unifies all relevant data in one place – Cognite’s solutions connect real-time and historical data, asset documentation, and operational insights, eliminating silos.
- AI-powered search and recommendations – Cognite’s Enhanced Troubleshooting solution allows engineers to interact with their data using natural language queries, significantly reducing the time spent searching for information.
- Automated root cause analysis – AI-driven insights help teams identify the most likely cause of failures based on past occurrences and similar cases.
- Improved collaboration – With a shared workspace, troubleshooting teams can work together efficiently, leveraging AI-generated insights and historical data for faster resolution.
The Business Impact of AI-Powered Troubleshooting
By adopting Cognite’s solutions, industrial companies can realize significant benefits. Teams can achieve faster problem resolution, codify their analysis, and be better prepared for the next quick response. Specifically, AI-assisted troubleshooting can cut the time spent diagnosing issues, reducing costly downtime. And engineers can spend more time fixing problems rather than searching for data, thus increasing operational efficiency.
The contextual insights delivered using Cognite enable teams to act based on a full picture of a problem. That leads to improved decision making when troubleshooting an issue.
Additionally, the predictive insights garnered working with Cognite’s technology can reduce maintenance costs by preventing unnecessary part replacements and optimizing maintenance schedules.
A Final Word
As industrial operations grow more complex, traditional troubleshooting methods are no longer sufficient. Organizations must embrace AI-driven, data-rich solutions to enhance their troubleshooting workflows. By unifying real-time and historical data, leveraging AI for faster root cause analysis, and providing a collaborative workspace, companies can resolve issues faster, reduce downtime, and drive better business outcomes.
With Cognite’s Enhanced Troubleshooting capabilities, industrial teams can stop wasting time searching for data and start solving problems—quickly, efficiently, and intelligently.