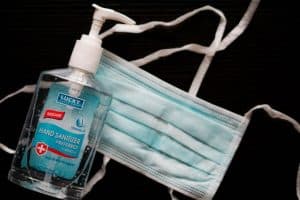
In the post-pandemic “new normal,” AI-driven intelligence will enable enterprise HR personnel to dynamically assess the operational costs, security risks, and productivity trade-offs associated with having employees work out of shared offices.
Pandemics are a business-continuity challenge unlike what most enterprise professionals have had to confront in the past.
When a pandemic strikes, human resources (HR) personnel may be caught off-guard by health authorities’ mandates that large segments of organizations’ personnel be quarantined and facilities closed for an indefinite period. As the COVID-19 pandemic recedes into history, it will leave a new best practice for enterprises to include in their HR handbooks.
See also: AI Bat Sensor to Monitor Social Distancing in Offices
In the post-pandemic “new normal,” best practices for containing contagions will fall into three broad categories, each of them directly enabled by sophisticated artificial intelligence (AI):
- Monitoring contagion extent and impact
- Determining when to impose and lift work-from-home mandates
- Identifying optimal office safety and sanitization practices
Monitoring contagion extent and impact
Monitoring the spread and severity of pandemics and other contagions will require nerve centers, dashboards, and the data and analytics needed to monitor the threats in real-time and to support useful predictions of how they might develop.
Counter-contagion nerve centers will become the core of the new normal, both in the public sector and in many large, geographically distributed enterprises. The purpose of these centers will be to coordinate preventive and remedial responses to these threats. These operations will consolidate information from various sources of health, immigration, customs, telecommunications, and travel information.
Chief among these sources will be two types of in-office sensors: those that sense the pathogens themselves (e.g., COVID-19) and those that sense the symptoms. An example of the latter is infrared thermal imaging cameras. Aggregating this data and applying sophisticated AI—historical, real-time, and predictive, the nerve centers will feed community contagion dashboards. These dashboards will provide every impacted person AI-tailored intelligence on their level of exposure and on how best to adjust their behavior in order to minimize the risks to themselves. They will also help the health and other authorities to better assess where to provide medical, pharmaceutical, and other resources needed to stem the outbreaks, prevent needless death and disability and keep society and the economy from grinding to a halt during the outbreak.
Determining when to impose and lift work-from-home mandates
In the post-pandemic future, the new best practice will be for HR administrators to use statistical models for guidance in orchestrating and optimizing their organizations’ lockdown and work-from-home policies in response to future outbreaks. Over the past several months, there has been a cottage industry in AI-based predictive models to forecast the spread, severity, and end of the COVID-19 crisis. For example:
- Carnegie-Mellon researchers have developed AI systems to track the spread of COVID-19 and retraining an algorithm to predict the seasonal flu.
- Robert Koch Institute researchers have developed a predictive AI model that shows how contagion spread can be contained through various containment tactics, including lockdowns, quarantines, and social distancing.
- Metabiota has developed an AI-based epidemic tracker and near-term forecasting model of disease spread.
One of the most interesting efforts in this regard is discussed in this recent research preprint. The paper provides a useful framework for generating AI-based prediction models to recommend lockdown strategies. The paper–co-authored by researchers at Microsoft, the Indian Institute of Technology, and TCS Research (the R&D division of Tata Consultancy Services)–describes a reinforcement-learning AI framework for helping cities and regions make policy decisions about lockdowns, closures, and physical distancing in response to pandemics like COVID-19.
To some extent, this framework—which relies on AI-based simulations–can be adapted for use by private organizations trying to optimize their own lockdown and work-from-home strategies. It uses AI to automatically optimize policies based on data regarding disease parameters such as infectiousness, gestation period, duration of symptoms, probability of death, population density, and movement propensity.
The framework models an infectious disease’s likely transmission path as a graph network consisting of 100 nodes and 1,000 individuals. Each node stands for a geography (such as an office or a city) that contains a certain number of individuals. The strength of the connections between pairs of nodes—in other words, the likelihood that an infectious disease would be transmitted between them—is directly proportional to the product of the population between nodes and inversely proportional to the square root of the distance between them.
In this modeling framework, an “open” node (which can also be equated with a shared office) allows people to travel to and from other open nodes in the network. A “lockdown” node is one into and out of which all travel has been blocked. People showing symptoms aren’t allowed to travel to other nodes, but asymptomatic and exposed people can do so. symptomatic people are quarantined within nodes, while a small number of people are able to break quarantine and circulate within a node.
On a per-node basis, the researchers establish baseline lockdown policies if the percentage of symptomatic people in that node crossed various predefined thresholds. Within this reinforcement learning framework, an algorithm makes per-node containment decisions— “open” or “lockdown” — by running simulations of the contagion’s spread. Identifying the optimal policy for lockdowns involved quantifying the cost of each outcome of the simulation, with the “reward” (in reinforcement learning parlance) defined as the negative of those costs. In experiments over dozens of simulations, the researchers determined that policies with 5-10 percent lockdowns experienced a lower peak of infections.
Identifying optimal office safety and sanitization practices
As lockdowns ease and group offices are reoccupied, the best practice everywhere will be to prep these facilities using several tactics:
- Sanitization-intensive maintenance: Custodial staff will wipe down and disinfect all surfaces daily, while office administrators now distribute hand sanitizer, masks, gloves, and sanitary wipes to all parts of the office on a regular basis.
- Contactless amenities: Facilities administrators have installed touch-free doors, garbage cans, and restroom fixtures.
- Group-averse facilities: Companies have closed shared meeting rooms, lunchrooms, coffee bars, exercise facilities, shared printers, and other infrastructure where groups would normally congregate.
- Prophylactic furnishings: Desks are now separated by plexiglass dividers, and covered with plastic shields to prevent the spread of infections.
- Lower-density layouts: Office layouts have been modified to spread desks further from each other, to reduce occupancy, and otherwise minimize the risk of people coming into close physical contact.
In addition, AI-infused tactics will enable a more comprehensive and fine-grained cleansing and sanitization of group offices, through such tactics as:
- Site exposure surveys: When considering whether to bring employees back to the office while the pandemic is still going on, organizations base their decisions on-site surveys informed by facility-embedded biosensors, augmented reality visualizations, and AI-abetted location intelligence.
- Comprehensive biosensing: Offices will be studded with biosensors to detecting viral pathogens in the air, floors, walls, ceilings, equipment, and every surface. Some organizations will require that their employees don wearable biosensors and use mobile-based contact-tracing apps to determine their exposure to disease carriers in the workplace. Infrared thermal imaging and other AI-equipped sensors will enable active surveillance and screening for infected and carrier persons in every facility. AI-powered computer vision will monitor and enforce social distancing guidelines.
- Robotic disinfection: We also expect to see AI-driven automation of the disinfection of group working environments. Robotics is key to this emerging practice. For example, one commercial solution–from UVD Robots—rolls through facilities and irradiate all critical surfaces with enough ultraviolet light to kill viruses and bacteria. Built for hospitals but adaptable to other in-building environments, the company’s flagship robot uses AI-driven sensors to scan rooms and create digital maps of their layout. Users annotate these layouts to specify where the robot should perform disinfecting tasks.
Takeaway
Remote work is not necessarily the future of all work. The new best practice in the post-pandemic age will be to ensure that all offices with two or more personnel conform to a basic level of health-conducive disinfection.
In the post-pandemic “new normal,” AI-driven intelligence will enable enterprise HR personnel to dynamically assess the operational costs, security risks, and productivity trade-offs associated with having employees work out of shared offices vs. “locked down” from home. Containment decisions such as these will need to be made dynamically from week to week in alignment with shifting trends and predictions of a contagion’s spread and severity.
Containment management best practices such as those outlined above will likely be a standard feature of enterprise HR tools in the future. Indeed, it’s a sure bet that many enterprises will crystallize these painful lessons into strategic planning frameworks for dealing with such emergencies in the future.
Read other articles by James Kobielus here.