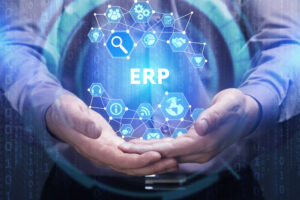
Key advancements in AI-powered master data management within ERP systems enhances data accuracy, streamline business operations, and improves decision-making across diverse industries.
Enterprise Resource Planning (ERP) software is always considered the backbone of business operations across various industries and serves as a critical tool for managing daily activities, like planning, execution, and reporting on business processes and financial health. A McKinsey report states that three-fourths of ERP projects fail to stay within the budget, and two-thirds have a negative return on investment, so organizations need to be cautious about how to make out the most from their ERPs.
ERP systems consolidate datasets from various departments of the organization—finance, procurement, logistics, and more— and compile them to provide a unified view and act as a single source of truth for the company.
However, to provide accurate insights and meaningful forecasts, ERP systems need to rely on accurate and reliable data, wherein data lineage plays an essential role. But given the scale and presence of the companies, data is often collected from multiple sources, locations, and even in different languages, due to which the risk of data duplication, inconsistency, and a lack of standardization becomes a recurring challenge. This highlights the importance of maintaining high-quality data to ensure that the ERP system functions effectively and provides actionable insights.
Master Data in ERP System
Regardless of a company’s size—whether large or small—or the type of business it operates, from B2B to D2C, each organization handles various types of master data, such as vendor master, material master, customer master, location master, employee master, service master, and more.
For instance, businesses operating within B2C or D2C models, like in food and beverage or apparel industries, may need to maintain extensive manufacturing databases and manage large customer datasets coming from multiple locations and systems. These datasets are often handled by personnel across all levels of the organization, from top executives to entry-level employees. This widespread involvement introduces significant challenges in terms of ensuring the accuracy and consistency of data, where the need for training and knowledge dissemination among staff can impose additional costs on the organization.
When these datasets are managed manually, the task of maintaining their accuracy and consistency becomes increasingly burdensome. The process often turns into a monotonous and time-intensive endeavor, heightening the likelihood of receiving inaccurate, unstandardized, or duplicated records in the system. This situation arises primarily due to insufficient training and lack of expertise, further exacerbating the problem of data quality.
While the ideal scenario would be to store and secure all datasets within a single, unified system, many organizations rely on various ERP solutions provided by different vendors to handle specific master data types, such as customer, employee, financial, service, product, and manufacturing data. This fragmentation of systems creates a significant challenge for businesses, as they are unable to obtain a comprehensive, consolidated view of their current business position.
Consequently, this lack of integration hinders the ability to accurately forecast trends and identify potential opportunities, leading to suboptimal decision-making, operational inefficiencies, and, ultimately, lost revenue potential.
AI in Master Data Accuracy
With the ongoing advancement of artificial intelligence (AI), industries worldwide are increasingly leveraging their capabilities to drive improved outcomes and enhance database management. Similarly, companies specializing in master data management solutions are innovating their products and software to address the numerous challenges associated with managing master data.
While human expertise remains a critical component of master data management—particularly in identifying inconsistencies—AI enhances the process by introducing significant improvements in speed, scalability, and precision.
To achieve optimal results, many organizations are adopting models that integrate both human knowledge and AI capabilities. AI excels at processing large volumes of complex data, automating routine tasks, and ensuring accuracy, while human expertise is necessary to interpret intricate data patterns and make informed, strategic decisions.
This complementary relationship between AI and human intelligence proves to be an effective approach to mastering the complexities of data management. Beyond the receipt and maintenance of master data, another crucial aspect is data governance, which is an ongoing backend process that operates throughout the data management lifecycle.
Master Data Governance is essential for ensuring that data remains clean, accurate, up-to-date, and consistent across all systems and departments. It provides the framework necessary for reliable decision-making and operational efficiency, as it guarantees the integrity of data across the organization.
AI’s contribution to enhancing master data accuracy extends well beyond simple data cleaning and organization. It plays a vital role in maintaining the long-term quality of data by eliminating duplicates and standardizing it according to industry taxonomies. This process ensures that data not only meets internal requirements but also aligns with globally accepted industry standards.
One of the primary challenges in master data management is addressing data anomalies arising due to traditional data monitoring techniques. AI helps eradicate data observability and organization and overcome data quality challenges by integrating advanced algorithms into master data management systems that consistently monitor and identify errors or discrepancies. Once anomalies are detected, AI generates real-time alerts, enabling quick resolution and ensuring that data remains consistent across all systems.
AI, therefore, acts as a continuous safeguard for data quality, allowing businesses to operate with confidence, knowing that their systems rely on accurate, up-to-date, and standardized information. By enabling continuous monitoring and enhancement of master data, AI not only addresses existing challenges but also positions organizations to anticipate future needs, thereby providing a solid foundation for sustainable growth and success.
AI in Master Data Management for ERP
Master data is the foundation of any ERP system, as it encompasses critical information such as product details, customer data, vendor records, inventory data, and financial records. Reports published by McKinsey state that 30% of the total enterprise time of employees is spent on non-value-added tasks as a result of poor data quality and availability. To alleviate the burden of routine, time-intensive tasks on their employees and to ensure the reliability and quality of their data, many organizations are increasingly integrating AI tools with their ERP software.
For organizations with offices and customers spread across the globe, AI’s integration into master data management streamlines processes for stakeholders by overcoming geographical and language barriers. It facilitates seamless data flow and simplifies translation, ensuring that accurate, consistent information is accessible to all users, regardless of location.
This integration not only improves the accuracy of their datasets but also streamlines business operations, leading to enhanced operational efficiency. For organizations in industries such as manufacturing, construction, oil and gas, and energy and utilities—many of which deploy digital twin technology—maintaining standardized and enriched data is paramount.
This up-to-date master data is essential for the digital twins to make accurate predictions regarding the maintenance needs of machinery and equipment. By relying on precise master data, digital twins can help organizations avoid costly downtime and last-minute repairs. AI plays a critical role in this process by integrating sensor data from equipment with the asset master data in the ERP system.
By analyzing historical performance patterns, AI can predict potential equipment failures and automatically generate maintenance work orders, ensuring machines are serviced proactively before they break down. Beyond these applications, AI tools are also instrumental in enhancing spend management and supply chain operations. With access to reliable, up-to-date datasets, AI can monitor spending patterns, automatically identifying discrepancies and flagging opportunities for potential cost savings.
This capability not only contributes to more accurate budgeting but also improves inventory management and demand forecasting. By detecting trends and anticipating requirements, AI enables businesses to optimize supply chain processes and reduce operational inefficiencies. Several key functions that AI performs to ensure data accuracy within ERP systems include:
Data Validation and Cleansing
By detecting and identifying duplicate data entries and inconsistencies in the data, AI ensures that the data is cleaned and validated; this improves data accuracy, reduces redundancy, and prevents errors in business operations such as order processing or inventory management. With automation, the tools automatically remove data, standardize the data formats, and correct the errors in real time.
For example: AI can recognize when the same product is entered under multiple names, like “Steel Bolt” and “Metal Bolt,” and automatically merge these entries into one standardized record, improving consistency.
Data Enrichment
A global retailer relies on accurate product data to manage inventory, pricing, and supplier relationships. AI can continuously update and enrich master data by integrating external information sources such as global product catalogs, industry standards UNSPSC(United Nations Standard Products and Services Code), which is widely used across all industries, PIDX (Petroleum Industry Data Exchange) majorly used in the oil and gas, ICIS Chemical Business particular in the chemical industry, IEC (International Electrotechnical Commission) Standards used for standardization in the energy sector, and GS1 Global Product Classification (GPC) majorly used in the food and beverages industry and third-party databases.
For example, AI algorithms automatically enrich material data in an ERP system with missing attributes, such as manufacturer details, material specifications, or regulatory compliance status, by fetching the details from external databases like regulatory databases or supplier websites.
AI for Data Integration and Consistency
Master data is often spread across various systems and departments; thus, ensuring data consistency across these systems could be quite challenging. AI facilitates data integration by merging data from disparate sources, ensuring that all systems reflect the same, consistent, and up-to-date information.
AI models can compare and reconcile data from different departments, such as sales, finance, and supply chain, ensuring that all teams are working with accurate and aligned data. This improves collaboration, reduces discrepancies, and ensures that business operations are based on a single source of truth.
For example, AI-powered tools align customer addresses recorded in different formats in various departments, ensuring consistency and accuracy, like data being entered as”123 Main St” and “123 Main Street”; the data sample will be normalized and improve customer retention.
AI for Real-Time Data Monitoring
AI enhances real-time data monitoring, an essential aspect of modern master data management. With traditional systems, monitoring and maintaining data accuracy over time requires constant manual intervention. AI, however, can automatically track and analyze master data in real time, identifying errors, discrepancies, or outdated information as soon as they occur.
This allows businesses to take proactive measures and make immediate corrections to data, ensuring that their ERP system remains up-to-date and accurate without delays. AI’s ability to continuously monitor data across multiple touchpoints ensures ongoing data quality, preventing issues before they escalate.
For example: When new product data is entered into the system, AI can automatically check for regulatory compliance by cross-referencing the data against the latest standards, flagging any discrepancies or missing certifications.
AI for Data Governance and Compliance
As per a report published by Gartner, 60% of organizations will fail to realize the anticipated value of their AI use cases due to incohesive data governance frameworks. Data governance and compliance are increasingly important, especially in regulated industries like healthcare, finance, and manufacturing.
AI-driven MDM systems support data governance by ensuring that master data adheres to internal policies and external regulatory requirements. AI can automate the enforcement of data quality standards, flagging any inconsistencies or non-compliant data entries. It can also track data lineage, ensuring transparency in how data is collected, processed, and used. Some of the ways AI is implemented for data governance are:
- Semantic Search for Data Retrieval: AI-powered semantic search helps improve how data is accessed within ERP systems. Traditional search engines often rely on exact keyword matching, but semantic search understands the intent behind the query, making it more accurate and efficient. This means that users can find relevant data even if they use different terminology or formats that do not match their exact same query; for example, a misspelled query would also give you back the correct results, making the software easier and user-friendly.
- Access Controls and Data Security: The governance control framework defined by the entity helps control the access of data, which helps organizations safeguard sensitive data from unauthorized access. AI systems can adapt access rules based on patterns of behavior, ensuring that only the right people have access to the appropriate data. This enhances data security and ensures compliance with regulations.
- Data Quality Framework: AI can automate and enforce data quality frameworks, ensuring that master data adheres to predefined standards. These frameworks consist of guidelines to assess data quality, such as accuracy, consistency, completeness, and timeliness. AI can evaluate master data against these criteria and flag entries that don’t meet the standards, ensuring that only high-quality data enters the system.
Conclusion
AI’s integration into master data management is transforming how organizations across the globe manage and maintain accurate, up-to-date information. By automating key processes like data validation, cleansing, enrichment, and integration, AI not only improves the quality of data but also ensures consistency and accessibility across regions. This technology is essential for global businesses as it helps break down geographical and language barriers, enabling teams to collaborate more effectively, make informed decisions, and optimize operations.
The combination of AI and human expertise fosters a more efficient, reliable data environment, which is crucial for sustainable growth and competitive advantage in today’s fast-paced business landscape. Ultimately, AI’s role in master data management strengthens organizations’ ability to meet regulatory requirements, improve operational efficiency, and drive long-term success.