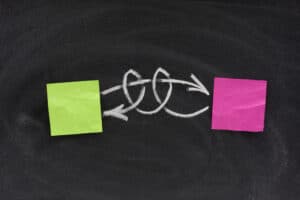
Integrating artificial intelligence (AI) into feedback-driven processes pushes the boundaries of what’s possible in automotive manufacturing.
One of the most significant advances for auto manufacturers is the chance to integrate artificial intelligence directly into the feedback loop. This integration is reshaping the industry, allowing manufacturers to improve quality more quickly. In one recent example, Audi deployed an artificial intelligence (AI) system to meticulously scrutinize 1.5 million spot welds on 300 vehicles during each shift at Audi’s Neckarsulm site. What are employees doing? Focusing on anomalies. The impact is efficiency and precision on a colossal scale, but that’s just the beginning.
Let’s explore the transformative nature of the feedback loop-artificial intelligence integration. It’s revolutionizing the way vehicles are built and assessed.
Understanding feedback loops in manufacturing
A feedback loop is a system that allows both humans and machines to learn from past input and make better decisions over time. For AI, the model uses output from the previous iteration as input for the next. Manufacturers can make incremental changes throughout the iteration process instead of waiting until the end to put products through quality control. The benefit is faster anomaly detection, allowing manufacturers to identify and address issues before they become huge problems.
Thanks to technology like IoT sensors, AI has access to an abundance of data within the complex auto manufacturing industry. As such, feedback-driven processes have become indispensable. Automakers can streamline the constant monitoring and analysis of various data sources, such as sensors, production equipment, and even employee input. Then, they can leverage this wealth of information to make immediate adjustments to the manufacturing process, ensuring that each vehicle meets the highest standards.
See also: You Can’t Spell Smart Manufacturing Without AI
The influence of AI
Although manufacturers have employed some measure of feedback loops for a while now, AI is actually a perfect companion to allow them to scale up—and quickly. AI can process millions, if not billions, of data points and quickly notice minute patterns humans might miss. This gives manufacturers an edge in the development and manufacturing cycle. Back to Audi, how long would it take for a human to process 1.5 million spot welds? Certainly more than the one shift mentioned in the report.
Another way companies are leveraging feedback loops is through digital twins. Back in 2019, Vietnamese car manufacturer VinFast began implementing these virtual environments in their development cycle. The combination of simulation and feedback from performance data from real products enables the manufacturer to shorten the development cycle and optimize products much earlier than what was possible before.
Digital twins, much like computer vision in the Audi example, are integral to a smart manufacturing process. These and other AI-driven tools enable a truly intelligent feedback loop thanks to:
- Real-Time Monitoring: Data from sensors placed on physical assets, processes, or environments provides a real-time view of the physical system’s status, enabling operators and engineers to monitor its performance closely.
- Data Analysis and Simulation: Automakers can apply advanced analytics, machine learning, and simulation techniques to understand data patterns, predict behavior, and identify anomalies or deviations from the expected norm.
- Comparison and Optimization: Algorithms can compare real-time data with predefined performance benchmarks or desired states. If there’s a discrepancy or issue, AI can suggest adjustments or optimizations to bring the physical system back into alignment with the desired outcome.
- Scenario Testing: Digital twins allow for the simulation of various scenarios and “what-if” analyses. By manipulating virtual representations of the physical system, operators can assess the potential impacts of different actions and decisions before implementing them in the real world. This speeds up iterations because there’s no need to waste time building a physical product for these experimentations and assessments.
- Remote Control and Automation: AI can be integrated into control systems thanks to computer vision, enabling remote operation and automation. When issues come up, automated processes can be triggered to make adjustments in the physical system with minimal to no human intervention.
- Predictive Maintenance: Digital twins can predict equipment failures or maintenance needs by analyzing historical data and real-time sensor information. This enables organizations to perform maintenance proactively, reducing downtime and improving overall system reliability.
- Continuous Improvement: As data continues to flow from the physical system to AI tools and back, organizations can use this feedback loop to implement ongoing improvements, refine processes, and optimize performance over time.
Companies can even leverage AI itself to decide if a human or machine is better suited to a particular job. Feedback loops here encourage greater efficiency and speed up decision-making, something critical in the auto industry where razor-thin margins already exist.
Some challenges still exist
Implementing AI feedback loops in the automotive industry can be highly beneficial, but it also comes with several challenges. Here are some of the key challenges automakers might encounter:
- Data Quality and Availability: AI systems rely heavily on high-quality data. Gathering and maintaining reliable data from various vehicle sensors and sources can be challenging. Data inconsistencies, inaccuracies, and incompleteness can affect the AI’s performance and decision-making.
- Integration Complexity: Integrating AI systems into existing automotive processes and systems can be intricate and time-consuming. Compatibility issues with legacy systems and hardware can arise, requiring extensive testing and adjustments.
- Training and Calibration: AI models used in feedback loops must be trained on large datasets and continuously calibrated to adapt to changing conditions. Maintaining the accuracy and reliability of AI models over time can be resource-intensive.
- Safety and Reliability: Implementing AI feedback loops requires rigorous testing and validation to ensure that AI decisions do not compromise vehicle safety. One recent study highlighted the potential for AI to “lie” or present outcomes based on humans’ perceived desires. Therefore, safety-critical applications must meet strict reliability standards, and companies need rigorous human supervision to prevent bias in feedback loops.
- Regulatory Compliance: Automotive manufacturers must navigate complex regulatory environments and ensure that AI systems comply with industry-specific safety and performance standards. Meeting these requirements while innovating with AI can be challenging.
- Cost and Resource Allocation: Implementing AI feedback loops demands significant technology, talent, and infrastructure investments. Allocating resources efficiently and managing costs is crucial for long-term sustainability.
Successfully implementing AI feedback loops will require a strategic approach, collaboration with technology partners, regulatory compliance, and a strong commitment to safety and data ethics.
The intelligent manufacturing system needs AI
As we move forward, the impact of AI on the automotive industry will only become more pronounced. Integrating artificial intelligence into feedback-driven processes represents a profound shift, pushing the boundaries of what’s possible in automotive manufacturing.
Additional Resources
Drive production excellence with intelligent manufacturing
The automotive industry is facing unprecedented challenges, with rapid change and intense pressure to meet the demands of climate change, regulations, and consumers. Download Now
Modernize automotive factories with Siemens Smart Manufacturing solutions
Discover how automakers can transform their business with Smart Manufacturing solutions using state-of-the-art hardware and software to modernize production lines. Watch Now
Virtual Manufacturing Development for the Automotive Industry
Companies in the automotive industry are pushing to develop the next generation of autonomous, electric, connected, and shared vehicles. View Now