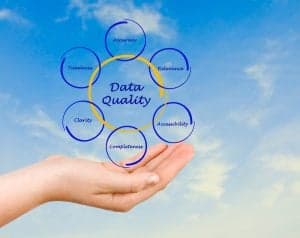
AI and ML applications need unified quality data from multiple silos and diverse formats that multiple workgroups can easily and securely access.
All artificial intelligence and machine learning initiatives, regardless of the resources organizations put behind them, have one important thing in common: they require well-managed, quality data.
That’s the word from David Baum, author of the recently released ebook Cloud Data Science for Dummies, sponsored by Snowflake. “ML models, and hence the decisions made from those models, are only as good as the data that supports them,” he writes. “The more data these models ingest and the more situations they encounter, the smarter and more accurate they become. And yet managing data remains one of the field’s most onerous tasks.”
To realize their full potential, data scientists should be working closely with their businesses, building the predictive models that put data to work. Yet, they spend almost two-thirds of their time “collecting, preparing, and visualizing data,” Baum states. A well-tuned ML algorithm needs unified quality data from multiple silos and diverse formats “to establish a single repository that multiple workgroups can easily and securely access.” Effective AI systems also should be able to access “near-unlimited data storage and compute power to scale data science apps from test to production.” Centralized data governance is also critical to the process, as it makes data science-driven insights available to anyone who needs it across the enterprise.
That’s why cloud-based data platforms offer a viable solution to manage and scale data environments that AI and ML initiatives require — they are well-known data hogs. Cloud services embed good data governance practices, and help “ensure fluidity among data science, analytics, and data engineering workloads,” Baum states. In addition, “a cloud data platform can also serve as the control center for sharing data among key business applications, such as connecting customer data in Salesforce with vendor data in Workday. A cloud data platform minimizes the amount of code between you and your data. Because some platforms support structured data, semi-structured data, and some forms of unstructured data, you can use a cloud data platform for your data lake and your data warehouse, bringing the two together.”
The following are measures AI and machine learning advocates can take to ensure they have quality data to build their data science capabilities:
Build a data foundation. “Take advantage of a cloud data platform that supports multiple types of data captured from various types of devices and applications,” Baum advises. “The platform should support popular data science programming languages, tools, and open-source environments to maximize options for your team.”
Identify the business problem. “If you want to predict an outcome, determine what will happen next, or make an educated guess about how a situation will evolve, you may need to build an ML model,” he states. “Rank potential projects based on expected business impact, data readiness, and level of executive sponsorship.”
Establish a skilled team. “You will need a data scientist or business analyst with the skills to build and train statistical models, a data engineer with experience building data pipelines and moving models into production, and a line-of-business leader or project manager to guide the effort,” Baum says. In addition, “before hiring new talent, see if you can train your existing team members to learn modern data science tools and adopt a predictive mindset.”
Build a culture of collaboration. “Standardizing on a modern cloud data platform enables everybody to
access the same data simultaneously, without having to copy or move the data,” Baum points out.
Measure, learn, and celebrate success. “Start small, identify metrics to demonstrate business results, and validate progress with executive sponsors and stakeholders. If you don’t obtain the results you were hoping for, step back, assess what went wrong, and try something else based on the lessons you learned. Apply successful outcomes to other departments and business problems.”
Scale the effort. “Look to the cloud and its boundless data storage and compute resources. You can start small and expand gradually to scale the effort on a pay-as-you-go basis. Rather than pursuing multiple proofs-of-concept in isolation, share best practices and encourage reusability. Strive to democratize analytics and extend ML capabilities to the entire organization.”