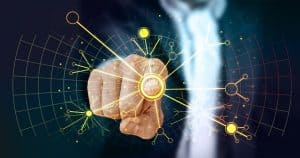
Google is trying to make it simpler for organizations to build and maintain AI applications at a time when there is often a disconnect between data scientists and the rest of the IT organization.
Google revealed it has embarked on a multi-prong effort to make it simpler to build and successfully deploy artificial intelligence (AI) applications faster.
A revamped set of tools for building conversational interfaces, rechristened Dialogflow CX, now makes it possible to construct omni-channel customer support engagement typical of a large contact center. Google has made available an Agent Assist module that can be employed now over both online chats and traditional voice calls and is making available in beta the ability to create unique voices using the Text-to-Speech application programming interface (API) exposed via Google Cloud.
See also: Is Your AI Model Still Accurate After the Coronavirus Pandemic?
Google is also moving to accelerate adoption of AI within specific vertical industry segments by making available Lending DocAI, an instance of its Document AI services for the mortgage industry that speeds up loan processing, and Procure-to-Pay DocAI, a service available in beta that automates procurement processes.
At the same time, Google is moving to make it simpler to build and deploy AI applications by adding a managed service for AI Platform Pipelines constructed using an instance of open source Kubeflow software for Kubernetes clusters that Google makes available on its cloud platform. AI Platform Pipelines promises to enable IT teams to automate machine learning operations (MLOps) in much the same way DevOps engineers rely on pipelines to create repeatable processes that accelerate the development and deployment of applications.
Google also committing to improving MLOps on its cloud by providing tools to evaluate the efficacy of AI models constructed data scientists along with tools to experiment with models and more easily track metadata attached to specific software artifacts.
Finally, Google is committing to opening a Feature Store repository by the end of the year to encourage reuse across AI projects.
Google is trying to make it simpler for organizations to build and maintain AI applications at a time when there is often a disconnect between data scientists and the rest of the IT organization, says Craig Wiley, director of product management for Google Cloud AI.
“In a lot of organizations data science teams are off to the side,” says Wiley.
It can take weeks for data scientists to first create an AI model before spending months working with IT to inject that model into an application. In the meantime, either the business assumptions on which the model was constructed have changed or more data sources that impact that model have become available. In either circumstance, the expected return on investment (ROI) that might be derived from those AI projects doesn’t materialize, note Wiley.
AI Platform Pipelines make it simpler for IT teams to operationalize and update AI models more frequently, promises Wiley. In fact, the best thing most organizations can do to maximize ROI for AI projects is to hire an MLOps engineer, adds Wiley.
It’s unclear to what degree reducing the friction level many organizations encounter when building and deploying AI applications will have on the initial selection of a cloud platform. However, in terms of ultimate success the time between when an AI model is first created and it becomes operational is nothing less than of the essence.