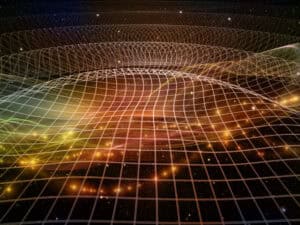
Digital twins and generative AI each have demonstrated their value across a wide range of use cases. Together, they serve to boost each other’s standing.
Since the dawn of digital twins a few years ago, it became obvious that artificial intelligence running underneath could assist in identifying, predicting, and offering workarounds for potential problem areas in the entities being digitally replicated, be they facilities, production systems. It wouldn’t have made sense to set up an installation without AI.
Now, there’s a new dimension to this relationship: Generative AI, which can serve as a capable assistant to those working with digital twins. That’s the word from a new McKinsey report, which sees this latest flavor of AI as a potential enabler of digital twins.
“Many organizations are separately implementing digital twins and generative AI — two technologies with distinct value propositions and tremendous promise — to support a wide range of use cases, the McKinsey co-authors, led by Alex Cosmas and Guilherme Cruz, observe. “Combining these technologies could produce synergies that reduce costs, accelerate deployment, and provide substantially more value than either could deliver on its own.”
See also: Digital Twins and the Next Generation Simulation Engine
Synergies abound with digital twins and GenAI
Digital twins and generative AI serve to boost each other’s standing. Generative AI is a tool that may ease the intricacies of digital-twin deployment, structuring inputs and synthesize outputs of digital twins, the authors state, Digital twins could serve to refine and validate generative AI output, as well as provide a robust test-and-learn environment for generative AI.
Separately, “each of these technologies has demonstrated its value across a wide range of industries and use cases,” Cosmas, Cruz, and the team point out. To enable AI projects, at least 75% of large enterprises are actively investing in digital twins to scale their AI solutions.
They explain what the convergence of generative AI and digital twins would look like. First, generative AI speeds up designing and building digital twins. “Building a digital twin, especially for highly specialized applications — such as multimachine production scheduling or vehicle routing — can be time-consuming and resource-intensive. The effort often entails designing and developing new digital-twin models, a process that can take six months or longer and incur substantial labor, computing, and server costs.”
By leveraging a software development platform such as GitHub, the large language models underpinning generative AI “can create code for the digital twin, accelerating the development process.”
This ability to generate such output “leads to an exciting prospect, the McKinsey authors point out: “LLMs could possibly be used to create a generalized digital-twin solution—a foundational, universal model—that facilitates design and serves as a starting point for developers across digital-twin projects and even industries.” Graph-based LLMs lend themselves to digital twin design, they add.
In turn, digital twins can deliver real-time data as a feedback loop to improve generative AI output. “With stores of robust, contextualized data, digital twins provide a secure environment in which gen AI can learn and broaden the scope of prompts and output,” the McKinsey team states. “Through what-if simulations run by digital twins, users can fine-tune gen AI, enabling it to conduct predictive modeling, as opposed to the primarily backward-looking view that most LLMs provide.”