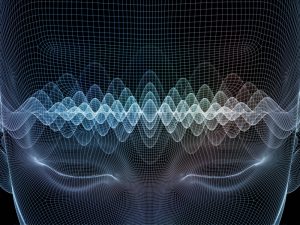
Machine learning and artificial intelligence are coming close to the peak inflated expectations stage in a hype cycle.
In a webinar, Gartner director analyst, Peter Krensky, outlined the current state of machine learning and artificial intelligence, the next five years, and some of the challenges likely to impact adoption, development, and deployment.
According to Krensky, ML and AI are coming close to the peak inflated expectations stage in a hype cycle. Augmented and virtual reality have already hit the ‘trough of disillusionment’, which follows the peak, and autonomous vehicles and drones were past the peak, but have yet to hit the bottom of the cycle.
That said, there is still a large amount of untapped industries for AI and ML. “Only 40 percent of top performers view artificial intelligence as a gamechanger,” said Krensky. “So, there’s a lot of greenspace within a lot of different types of organizations, even whole industries that are just dipping their toes into what’s possible with machine learning.”
One of the factors in favor of AI and ML’s continued development is the alignment between academic, scientific, and corporate core concepts. Over 90 percent of the industry write AI and ML algorithms and platforms in Python, many use the same open-source libraries (Apache, TensorFlow) and the same cloud infrastructure (AWS, Azure).
SEE ALSO: Gartner: 77% Organizations Aim To Deploy AI, Staff Skill Holds Adoption Back
This alignment leads to greater synergy across the industry, which could be valuable when recruiting talent in the future.
Across the industry, there is a steady migration to the cloud, while about 30 to 45 percent continue to run AI or ML on-premises. In many cases, Krensky believes organizations are spending too much building the AI or ML program, avoiding cheaper alternatives like pre-trained models and cloud infrastructure, when the results would be similar.
“We have to get used to machine learning infrastructure being in the cloud and an ever-evolving set of proprietary and open-source components as the new normal,” said Krensky. “Every year there’s a new open-source framework that we weren’t talking about two years ago and now we’re talking about all the time.”
Looking at how AI is implemented, Gartner has four models:
- Pass the baton – The AI will work on the tedious part of the task, and the human operator will complete the rest.
- Symbiosis – The AI is able to cover for a human’s weaknesses, for example by processing vast quantities of data in seconds.
- Augmented analytics – The AI is used as a trainer or guide for the human operator, providing analytics or insights from the data. An example of this could be a recent AI project that suggested missing words or sentences in ancient Greek engravings.
- Sanity check – The AI performs most of the functions, but has a human operator on standby to watch for mistakes. For example, a customer support bot that passes difficult cases onto a human.
In a survey by Gartner, most business leaders said skills of staff was the main challenge for AI and ML adoption, at 56 percent. Krensky mentioned in the webinar that most ML developers are young, have less than five years’ experience and have been at their job for less than two.
On top of that, Gartner sees the success of a project as having multiple staff members with different expertise. Data scientists, data engineers, ML specialists, and domain experts all have a distinct role to play in the development of an AI or ML project, but frequently organizations rely on data engineers with broad knowledge.
The second biggest challenge was understanding the benefits and uses of AI. In the webinar, Krensky recommended that organizations need to correctly manage the AI project and not invest in all areas, instead focusing on a few key zones that make financial sense.
Data scope and quality is the third biggest challenge and one that Krensky believes should be top of the list. Without proper data management, quality checks, and data governance an AI or ML project is much more likely to fail.