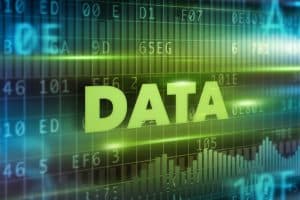
The volume of data is outpacing the ability to handle it. As such, more attention must be placed on automating data preparation.
Data is growing, and so is the time spent on data preparation to retrieve, process, and manage it. In the pursuit of real-time business intelligence, the enterprise’s reaction to big data is ambitious yet insufficient and inconsistent. The volume of data is outpacing their readiness to handle it.
Such inefficiencies are a concern largely when modules like preparation account for 44% of a professional’s time. In a survey of professionals, data scientists end up consuming 40% of their working hours in manual data preparation while only 11% is spared for their core tasks. Now imagine how much you are losing if the same preparation could be automated. Consequently, sluggish and error-prone processes are doing more damage than helping the data science ecosystem.
This increase in data traffic and the attached challenges has compelled organizations to think beyond and embrace contemporary data preparation solutions. As per Markets And Markets, we are in the middle of a data preparation market growth rate (CAGR) of 25.2%. If that is true, organizations should wake up to self-servicing data preparation methodologies.
Self-servicing tools automate the data preparation methods, thereby enabling the data scientists and the business users to execute the life cycle with ease.
That is, the process starting from exploration, accessibility, profiling to cleansing and transformation occurs in a predefined yet interactive pattern. Since the self-servicing tools hosted in cloud-native platforms automate the life cycle, the users (and other professionals) get to focus on core analytics. To put it simply, it empowers non-tech professionals such as business users to execute the preparation life cycle without the skills of coding or knowledge of the underlying technology layers.
How does it work? After collection and reconciliation, the self-servicing tool scans the data files through a workflow that is designed to perform all the steps iteratively. By the end of the workflow, the datasets populate into a final file that is further loaded into a data store or a warehouse for business analytics.
While selecting a self-servicing tool, check for the following attributes to ensure optimal value:
- Compliance with all data sets: Exploring and accessing should support all sources such as Excel, CSV, etc., to data lakes, warehouses, and SaaS platforms.
- ML engineered cleansing, profiling, and enrichment functions.
- By default, support for self-triggered discovery, profiling, standardization, suggestions, and visualization.
- Seamless function export to different file types such as Excel, SaaS native formats, analytics dashboard like Tableau, etc.
- Support for features like automated versioning, advanced designing for a variety of ETL processes.
Data preparation expertise a must
Despite the availability of self-servicing tools, enterprises struggle with their data preparation expertise and do not really avail the benefits for real-time as well offline applications.
This happens due to complex UI and the inability to populate qualitative data persistently. At the core, it is the conventional preparation methods such as database-by-database, row-by-row, and table-by-table. Not to miss, the complex joins to other tables through scripts and indexes. Here, the data mapping and validation logic is complicated and requires assuring referential integrity for every request.
To address this, micro-databases could be used to store and populate data for every business entity. Subsequently, it performs end-to-end data preparation (discover, collect, cleanse, transform and mask) for a specific business entity as and when required. Each of these business entities would store a single customer’s master data.
Among many attempts over the years, K2View’s Data Fabric is the most successful case study in using micro databases for automating data preparation. It captures the data from multiple source systems and stores them as a standalone digital entity in an exclusive micro DB. This micro DB is readily available for consumer apps.
The solution achieves end-to-end data preparation at the business entity level. Unlike conventional approaches, this data preparation hub defines a digital entity schema including all attributes for the specific business entity regardless of their source systems. It automatically locates the desired data sets specific to the business user in the system’s landscape and creates a connection to all those sources. The system performs automated synchronizing of datasets with the sources on a predefined schedule. Not to miss, it automatically implements filters, enrichments, and masking.
Besides optimal utilization of resources, such an approach leads to complete, correct, and qualitative data preparation.
Remember the end goal
Personalized, faster, and profitable consumer experience should be the ultimate goal of all business processes, including data analytics & preparation. Unless you strengthen your foundation, the impact at the front-end is bound to suffer and affect the utility of your products and services. That being said, there’s only one principle to master data issues: act upon them in advance.