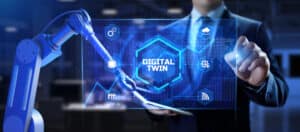
Digital twins are helping to address and provide transparency into matters keeping manufacturing leaders awake at night, such as forecasting abilities, material and labor constraints caused by rising costs, and talent gaps.
Digital twins have many applications, but nowhere is uptake as strong as in the industrial sector — where almost six out of ten executives either have either already implemented or planning a digital twin. In the process, they are reporting reduced downtime from production bottlenecks, accelerated production schedules, and improved inventory flow.
That’s the word from a recent analysis by McKinsey, whose survey of senior executives finds a majority, 86%, say they see digital twins as applicable to their organizations. At least 44% say they have already implemented a digital twin, while 15% are planning to deploy one.
Factory digital twins – real-time virtual representations of the factory – “provide manufacturers with the ability to support faster, smarter, and more cost-effective decision making,” the McKinsey authors observe. Digital twinning helps to understand “complex physical systems and production operations, optimize production scheduling, or simulate what-if scenarios to understand the impact of new product introductions.”
Digital twins in action are unearthing bottlenecks on production lines, the authors report. Digital twins are being integrated into manufacturing execution system platforms, internet of things devices, and inventory databases. This is helping to address and provide transparency into matters keeping manufacturing leaders awake at night, such as forecasting abilities, material and labor constraints caused by rising costs, and talent gaps.
Factory digital twins “can be integrated into real-time decision making, such as production scheduling – either with manual review and intervention or through full automation,” the authors state. They also serve in designing and constructing new facilities.
See also: Companies Double Down on Digital Twins, But Will Infrastructure Hold Them Back?
Digital twins can predict production bottlenecks where traditional modeling in spreadsheets falls short, they add. “Hard-to-predict stochastic processes, inventory buffers, material travel times, and changeovers can all be modeled with high fidelity using live data.”
The following are the ingredients that go into digital twins:
- Sourcing, storing, and processing data: “Data sits at the base of the tech stack, comprising production data sourced from programmable logic controllers and manufacturing execution systems to indicate the status of the line and most recent cycle times by asset. Inventory data shows raw-material availability, current work in progress, and finished goods, while demand data is ingested either directly from the customer or through the ERP.”
- Systematic data cleaning: This ensures modeling is “conducted in a repeatable and expected manner,” according to the McKinsey authors. “Data is cleaned, structured, and compiled, typically into intermediate data tables designed for consumption by the simulation tool.”
- Standard language: “Data service integration software enables data from disparate streams to be united into a common data pathway for processing and segmentation. This allows data to be manipulated and organized into a useful and categorical language for integration. Creating one common data model that integrates disparate data sources enables a step-change in operational insights.”
- Simulation software: “The most accurate way to simulate the factory floor is with discrete event simulation software or natively built code. This produces a virtual rendering of the factory to run thousands of simulated production sequences to identify bottlenecks and production constraints.”
- Optimization: “Layering optimizer software on top of a digital simulation enables the digital twin to run millions of hypothetical production sequences and isolate the optimal sequences that maximize productive time,” according to McKinley. “Although optimization approaches have been around for decades, recent advancements such as genetic algorithms, Baysien-based ‘optionization,’ active learning, and deep reinforcement learning are game changing in creating new ways to optimize the factory.”
- Artificial intelligence: “Incorporating machine learning algorithms allows for sequences to respond to both historic patterns and real-time variance to create a system of repeatable business rules that can step-change production output,” the authors state. Combining these machine learning and optimization approaches with a simulated replica of the plant and leveraging the latest in high-performance computing is allowing companies to drive a new level of performance in real time.”
To get started with a digital twin, the McKinsey team advises starting small with a proof of concept. Elements such as data feeds and architecture need to be developed.