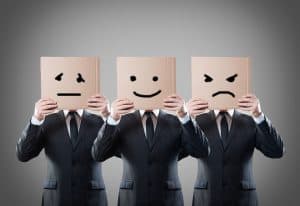
Emotion AI is about understanding the behaviors that let you measure and influence the emotions in an interaction.
Robots or chatbots that can sense or respond to stress in callers’ voices may go a long way to delivering customer or employee satisfaction. However, they have a long way to go. That’s the word from a recent report by Stephen Gossett, who explores the potential of emotion AI, or AI that “that detects and analyzes human emotional signals.
Gossett’s company’s technology maps verbal information — such as tonality, vocal emphasis, and speech rhythm — in call-center conversations in order to better match up representatives.”
See also: Credible Chatbots Outperform Humans
Emotion AI Challenges
Gossett explores the challenges seen across key categories of emotion AI:
Text emotion AI, NLP and sentiment analysis: Sentiment analysis “is a little bit controversial because there are questions about accuracy and usability — whether numbers actually correspond to real-world sentiment,”
says Seth Grimes, founder of Alta Plana and creator of the Emotion AI Conference. “To give an indicator of the complexity, I use an image in my presentations of Kobe Bryant smiling on the basketball court. And I ask, ‘How does this make you feel?’ Well, Kobe Bryant died a year ago in a tragic crash. So [just] because Kobe Bryant is smiling, that doesn’t make you happy. Maybe it’s sad, but sadness about someone who has died is actually a positive sentiment. There’s a lot of complexity and subjectivity.”
Audio and voice emotion AI: The key here is capturing and processing “honest signals,” says Skyler Place, who leads behavioral science at Cogito. These are “everything in the conversation besides the words — energy in the voice, pauses, intonation, the whole variety of signals that help us understand the intentions, goals, and emotions that people have in conversations — a very, very rich signal of information.” The next leap forward will come from the ability to “combine the understanding of NLP with the honest signals — that’s going to give us a novel way to understand and improve the emotion in conversations as we go forward. We have about 200 different signals that we utilize to recognize these behaviors. And then we link the behaviors to the outcomes that are valuable for call-center calls. That’s how we think about emotion; less about pure recognition, more about understanding the behaviors that allow you to not just
measure, but influence, the emotions in an interaction.”
Video and multimodal emotion AI: “What’s made this possible is the investment in camera technology over the past 20 years — cameras that have high-quality sensors with low noise,” says Daniel McDuff, principal researcher at Microsoft AI. “Using a camera even with a simple signal-processing algorithm, if you analyze skin pixels, you can typically pull out a pulse signal and also detect respiration for a person who’s stationary. The camera is sensitive enough to pick up the initial signal, but that often gets overwhelmed by different variations that are not related to physiological changes. Deep learning helps because it can do a very good job at these complex mappings.”