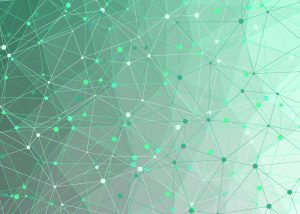
A Data Mesh approach removes the bottlenecks that come with the traditional data warehousing and data lake models, and in doing so, allows companies to drive faster insights.
The data warehousing model has owned the data management space for years. In this centralized model, data is typically passed from a number of operational systems to a single analytical system, with data engineers and analysts then trying to understand and derive value from that data. This model creates a bottleneck between the data producers and the analysts since we need a third function that is responsible for actually migrating the data from operational systems to the analytical system. This bottleneck results in an “air gap” between the data producers and the analysts, meaning that the analysts cannot be as “close” to the data and its original context, and this bottleneck becomes a key scalability constraint.
The data warehouse was traditionally designed to enable and support an organization with business intelligence through analytics, reports, and dashboards. This approach is predicated on consolidating data assets to create a “single source of truth.”
One of the reasons that the Data Lake concept was born was to overcome some of the challenges associated with Data Warehouses. In this approach, data is again brought from multiple sources to a single location for the purposes of analysis, but in this approach, the data is not modeled to form a single source of truth when it is bought together. Instead, the data is effectively modeled when it is exploited by the analysts, so now each analyst can determine their own single source of truth. This is a very flexible model for the analyst but suffers from a lack of governance around the data and thus the decision-making. However, the bigger concern is that it has not removed the need for a central team for getting the data to the Data Lake in the first place.
To some degree, the Data Warehouse and Data Lake are at opposite ends of the spectrum when it comes to defining a single source of truth but are very similar when it comes to the centralization of ownership of the process of getting data from the operational systems to the analytic systems. This centralization of ownership slows down the organization’s ability to respond to changing business needs.
See also: Data Lakes, Time-Series Data, and Industrial Analytics
Defining Data Mesh
Zhamak Dehghani, a principal technology consultant at ThoughtWorks, introduced Data Mesh as “a sociotechnical approach to share, access and manage analytical data in complex and large-scale environments – within or across organizations.” Rather than having a centralized monolithic pipeline for all of your data, Data Mesh considers each group of human experts that manages a particular set of datasets as a “domain,” who are responsible for producing “data products” that are then consumed by anyone in the organization in a self-serve manner. There are four key principles of Data Mesh: domain ownership, data as a product, self-service data infrastructure, and federated computational governance.
Understanding the business and data at a domain level is critical. Through domain ownership as part of Data Mesh, domain teams are entrusted to manage the operational data, the data migration/transformation, and the analytical data. With this approach, domain teams who understand both running the business and optimizing the business through data can design the data types and file formats needed by data consumers to support decision making while also responding and pivoting faster. As domains in large-scale organizations conduct planning exercises around new products or features, they can also plan for how to represent data to take account of those changes. So even though the Data Mesh approach assigns additional responsibilities to the domains, it also empowers them with autonomy to represent their data the way they see fit.
A key part of domain ownership lies in producing high-quality data products, which means the pipelines that create high-quality analytical data—including the extraction from operational systems, the cleaning, and conforming—must be owned and managed within the domain. Domain ownership doesn’t necessarily mean data pipelines are going away, but they are becoming modularized, with each module being owned and managed by a domain. This means that the self-service data platform needs to make it easier for non-technical data teams to support the creation and management of necessary pipelines for data products.
See also: Center for Automated Integration
Treating data as a product
When we consider treating data as a product, we are mandating that data must be usable, and there are baseline data usability characteristics that every data product must exhibit. It must be discoverable, understandable, addressable, secure, trustworthy, natively accessible, and valuable on its own. Data as a product creates a new world view where data can be trusted, built, and served with deep empathy for data consumers.
This combination of decentralized data ownership and treating data as a product as part of a Data Mesh approach removes the bottlenecks that come with the traditional data warehousing and data lake models, and in doing so, allows companies to drive faster insights. Is your organization ready?