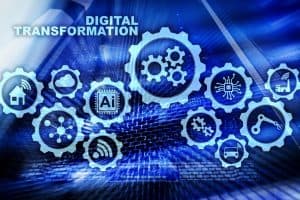
Successful digital transformation initiatives include powerful AI and machine learning techniques to process and analyze large volumes of data across the enterprise.
Digitalization has been a popular topic in business for years. No matter the industry, digital transformation is usually top of mind for executives striving to keep pace with massive amounts of data. The Office of the CFO, the traditional warehouse for numbers and figures, is faced with the unique opportunity to tap into tools such as Artificial Intelligence (AI) and machine learning, to expand efficiency in planning, budgeting, and forecasting. AI offers the opportunity to add more value to the entire organization by supporting the finance function.
According to Gartner, most finance teams still rely on robotic process automation (RPA), a process that can only automate simple, repeatable tasks but not more complex processes and workflows. AI-powered solutions, however, can. AI can take the Office of the CFO to the next level through smart data analysis and predictive analytics.
Essentially, AI is little more than a set of algorithms, statistics, and numbers that automate certain processes that do not require non-quantitative feedback. It is a solution that does not replace human beings but rather augments their judgments by providing real-time insights while eliminating the risk of human error. AI offers a faster, more efficient way to analyze data and identify certain patterns.
Traditionally, budgeting and forecasting were done by pulling data from multiple source systems into Excel. As the amount of digital records from every corner of the enterprise has exploded, the likelihood of making mistakes has increased astronomically. While the need for automation has grown, the biggest concerns finance executives have about AI adoption is getting executive buy-in along with its actual implementation, which many fear would translate into a heavy reliance on the IT department. Further, finance teams may require new skillsets for which they are not trained. There is no ‘getting around’ digitalization. Below are the top three hurdles in addressing digital transformation in finance today.
See also: Accelerating Digital Transformation: Low Code + Composable
Hurdle #1: Turning Data into Insights
Advancing digital transformation efforts paired with expanding the Office of the CFO’s reach across the entire organization both accelerates the opportunity and the complexity of handling massive amounts of data. Turning data into valuable insights is a challenge. It is significantly more difficult without the support of AI tools. In fact, most enterprises are still only in the embryonic stage of tapping into their data’s enormous potential by relying solely on RPA to drive efficiency. For finance teams attempting data consolidation, they are often faced with having to integrate disparate systems with the help of IT, analyze large datasets, and do it in a consistent and value-driven manner. Whether it is data from a CRM, ERP, HR, or other internal systems, everything needs to be unified, enriched with relevant external data, and analyzed intelligently. AI tools can ease this process considerably by helping us recognize patterns that natural human bias can miss. Relying on these patterns, finance teams can increase confidence in the data they provide to the CEO. It allows finance to turn data into valuable insights by creating patterns that could even predict future anomalies in your data. AI is about taking statistical patterns and turning them into even more reliable patterns for the future.
Key questions finance should be asking:
- Which external data sources add true value?
- How can we unify internal data silos?
- How can we combine cloud and on-premises data sources? Do we even need both?
- How can we ensure a consistent structure that yields high data quality?
- How can we handle access to sensitive data for various stakeholders?
Hurdle #2: Building Skillsets in Analytics and Adopting Solutions
Multiple data sources present a real opportunity for CFOs. It is not just about gathering data but about advanced analytics, which would require data science skills to be able to interpret and draw insights from the information. Clearly, these skillsets are still not common and require an investment that may not seem readily needed on the surface. The finance department cannot rely solely on the IT function or external Business Intelligence resources to support daily analytics requirements. The process would be too cumbersome and confusing. Instead, the goal is to develop a new Office of the CFO’s analytics resources in order for it to become the hub for company-wide analytics initiatives. Innovative FP&A tools offer technologies such as AI and machine learning to give finance new capabilities in a familiar application environment and decrease reliance on IT.
The trend is clearly toward even heavier use of AI in the future, which will be built into CRM and WMS systems. It will ease the process by automatically addressing supply and demand and fulfilling orders. As AI takes over more of the day-to-day processes, finance teams will be freed up to create more value based on the analytics it provides.
Hurdle #3: Expanding the Business through AI
As we have seen, successful digital transformation initiatives include powerful AI and machine learning techniques in order to process and analyze large volumes of data across the enterprise. As a result of identifying specific data patterns, insights, and predictions through these tools, the Office of the CFO will continue to expand its scope across multiple lines of business, identifying each particular requirement and ensuring that business functions such as HR and sales are able to act on new insights intuitively without in-depth analytics expertise. The CFO needs intimate knowledge of the business and flexible IT systems to adapt, refine, and embed AI results into essential business applications. Below are five key technologies to ease the digital finance journey.
Five Key Technologies for Digital Transformation in Finance
• Robotic Process Automation (RPA): As mentioned above, RPA allows finance teams to automate processes such as time-consuming data acquisition, reconciliation, and verification. Over time, it helps improve consistency and control while unshackling resources for more advanced tasks. But it is only the beginning.
• Predictive Analytics: While no one has a crystal ball, AI-driven predictive analytics is the modern-day answer to making future predictions based on current data. These predictions can help drive strategy and overall decision-making. Predictive analytics relies on multiple advanced analytics techniques such as data mining, statistics, and machine learning algorithms.
• In-Memory Database: When utilizing complex modeling and calculations scenarios such as budgeting, planning, and forecasting, in-memory database technology provides the necessary scalability and performance for fast, flexible data analysis. The data is stored for faster access and response times.
• GPU accelerated computing: Graphics Processing Unit (GPU), or graphics card, boosts the speed and performance of real-time data analysis, providing businesses with more agile decision-making capabilities.
• Data Hub: AI and machine learning thrive on data. Drawing data from different sources for analytics by consolidating it from multiple systems that are either whether in the cloud or on-premises is even more essential with these techniques. Advanced data integration and preparation, including mapping, cleansing while enriching datasets is a key technology for the digital enterprise.
Data is the new currency. CFOs with the capability to unleash its full potential will add measurable value to the entire enterprise. AI and machine learning are here to stay. Companies that take the step forward to create a strategy for working with this technology will significantly increase business value and performance.