How Data Observability Enables the Modern Enterprise
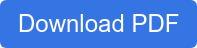
Introduction
As businesses embrace digital transformations and the increased use of sophisticated analytics in all aspects of their operations, their data-driven environments require data reliability and a data operations approach that meets the needs of the modern data stack.

As data pipelines become more complex (especially with the move to distributed cloud-based applications) and development teams grow to meet the increasingly complex needs of the modern data environment, organizations need to re-examine traditional processes that govern the flow of data from the source to consumption. In particular, they need processes to be automated and AI-based so that the business can respond to the increased scale of data and its increased complexity. More importantly, they need insights into what is happening to the data and context for how it operates as it moves through the entire data pipeline. And they need assurances that the data being used is reliable.
The issues go far beyond the needs of data engineers and data teams. Virtually all aspects of operations and everyone from business users to IT staff to DevOps to SREs need regulatory-compliant access to data that is current, high-quality, and highly available.
The bottom line is that most businesses face challenges with their data, and thus, they must transform their approaches to data management. Specifically, they need more insights into data issues throughout the data lifecycle. Such insights are available when a business adopts methods based on data observability.
Common data obstacles
All businesses face numerous data-related issues. One frequent problem is that good data can become bad. With all the touchpoints from data creation/ingestion to its ultimate use, there are many ways for data to be corrupted, deleted, or misused at different steps in its lifecycle.
Most enterprises use data solutions that are opaque, and the lack of transparency hides data issues and can impact data quality and application performance. The migration to the cloud has made it more critical that organizations have greater management and insights into data issues. Lacking such information and control can cause problems throughout the continuous lifecycle of data as it is used throughout an organization.
Additionally, many of the tools used to manage and monitor data only work on a specific part of the data lifecycle. For example, the current way organizations manage quality is manually by writing ETL scripts. The problem with this approach is that the method to not scale, and the approach prevents data engineers from working on higher-value projects. Additionally, tools that help ingest and prep data for use by applications are used by specific people and groups. These tools are self-contained, performing functions relevant to the particular group that handles data prep, and typically do not make any information available to others involved in later stages of the data lifecycle. The consequences are that if an error creeps in or a performance problem emerges at any stage, there is no way to take a higher-level view of the continuous processes and resulting problems. However, before looking for a solution, it is important to step back and look at the numerous data-related issues that businesses face today and why data observability is getting more attention.
See also: Multidimensional Data Observability
A major contributor to data problems today is the fact that data pipelines are more complex than before. For example, in the past, data was ingested from a database into a data warehouse using a basic ETL or CDC tool, sometimes transformed, cleaned, and tested for quality. From there, it was stored and transmitted onward to a simple application that ran a routine on the data to produce a report or use in a dashboard.
Now, data comes from many more sources besides databases, including via APIs, files, social media, logs, and more, and that makes the ingestion process more complicated. Add to that the fact that more of the data is dynamic. Rather than using unchanged data from a database (e.g., account information about a customer), a modern application might use events data (e.g., the customer has just made a query or initiated a purchase). These changes impact data transformation, storage, delivery, and consumption. And at every step of the way, there is a potential source of trouble. Also, in the past, no one tool provided insights into issues at every step of the data pipeline.
Other issues abound. For example, many businesses have vast amounts of data on legacy systems that they would like to use in new applications. The common problem here is that most companies have difficulty accessing data on legacy systems. The move to an API-centric model is giving many companies a way to provide access to such data.
Even if access is achievable, there is the problem of working with many siloed systems. That means there are multiple people involved with data management and multiple data management tools in use. Quite often, there is no uniformity in what the tools accomplish and what information is made available.
These and other issues can lead to multiple data reliability and data quality issues. There needs to be a way to understand if the data is complete and what the quality of that data is. Additionally, there also needs to be a way to understand how data changes over time. And when multiple systems are involved, data quality and reliability go down when data on those systems go out of sync.
A checklist to transform data operations
Numerous people, job types, and groups engage with data throughout an organization, and there are multiple touchpoints in the data journey as it is created, ingested, shared, transformed, processed, and analyzed. As a result, issues related to data operations impact the entire organization. So, decisions about data cannot be the sole domain of a single group or business unit. What’s critically needed is a high-level vision for data strategy that aligns business goals with technology.
That strategy should include the steps that must be taken to transform data operations from one that is loosely managed by individuals and departments to a cross-organization plan with a purpose. Some essential checklist items that all organizations should address to accomplish this include:
- Build a data-driven culture: Data issues must be front and center. Everyone involved in any aspect of a data pipeline must understand the business impact of data problems and the downstream problems any issue at any step in an end-to-end data pipeline might cause.
- Hire the right mix of data skills: Data goes through multiple stages from its source, including ingestion, transformation, storage, delivery, and consumption. Each stage requires special knowledge and skillsets.
- Realistic planning for digital transformation and the use of a modern data architecture: Data is useless if it is not accessible and available at the right moment when an application or other data source needs it. A modern cloud data architecture simplifies access and easily scales to accommodate growing data volumes, distributed applications, and the need for on-demand processing.
- Implement data governance to ensure compliance and security: Some businesses make the mistake of conflating data management with data governance. Data governance requires addressing the roles, responsibilities, and processes for ensuring accountability for and ownership of data assets.
- Automate data discovery and data anomaly detection: Automation obviously replaces manual processes, but it should also include the flexibility for data teams to set their own thresholds to monitor data according to business needs.
- Ensure data quality/data reliability: Institute data management best practices to standardize and improve data quality monitoring, so every team is speaking the same language.
- Implement self-service analytics: Encourage end-users and data analysts without a large depth of technical background to have confidence to perform queries, reports, and analysis using simplified BI tools and processes, and with easy to understand visualization tools for non-professional analysts to formulate the results.
The role of data observability
Data quality is not a given, and data problems do not happen in a vacuum. An organization may start with pristine data, but as it traverses the pipeline from ingestion to results, unwanted and unknown changes or problems can occur. Data may be corrupted or lost along an analysis pipeline. Or a transformation to put the data into useful format might be done incorrectly. Such small problems can have major consequences. An extreme example is NASA’s loss of its $125 million Mars orbiter. In that case, calculations for thrust firings to maneuver the spacecraft were wrong because one piece of ground software produced results in a United States customary unit, while a second system expected those results to be in SI units. That problem happened years ago but was unique in that such a small step and small issue ended up with such severe results. The issue persists today. Gartner recently noted that poor data quality costs organizations, on average, about $13 million per year.
Organizations must avoid such problems and have end-to-end real-time insight into their data. Enter data observability. Observability, in general, recently has become the darling of the IT world. In the past, most efforts centered on monitoring, which would tell those involved what was happening. In contrast, observability helps explain why things are happening.
In particular, data observability is an approach and a solution for data operations that enables monitoring, detection, prediction, prevention, and resolution of problems across infrastructure, data, and data pipelines in real-time. The more observable an enterprise application is, the easier it is to determine the root cause of any problems that affect it. As issues are identified and fixed, the application becomes more reliable and efficient. Additionally, data observability improves control over data pipelines, creates better SLAs, and provides insights to data teams that can be used to make better data-driven business decisions. Data observability solutions provide clear advantages over monitoring tools in several general ways, including:
- Providing data teams with more control over data pipelines.
- Allowing data teams to ensure high-quality data standards by automatically inspecting data transfers for accuracy, completeness, and consistency
- Enabling data engineers to automatically collect pipeline events, correlate them, identify anomalies or spikes, and use these findings to predict, measure, prevent, troubleshoot, and fix problems.
- Digital Transformation and Data Observability 5 Data observability can help a business manage the health of the data in its systems, eliminate data downtime, improve data pipelines, and address the skills issue since data teams are more efficient and effective by giving them visibility. In particular, what’s needed is modern data governance and data quality observability solution. Such a solution should ideally provide:
- Automated data discovery and smart tagging that simplifies tracking data lineages and problems
- Constant data reliability and quality checks, rather than single-moment-in-time testing of classic data quality tools
- Provide data pipeline cost analysis so data teams can optimize both the performance and cost of the data pipeline by eliminating underperforming and slow data pipelines and analyze the cost of data pipelines across different technologies
- Machine learning-powered predictive analyses and recommendations that ease the workload of data engineers, who are currently either bombarded with too many alerts
- A 360-degree view of an organization’s entire data infrastructure that can identify the main sources of truth, prioritize validating the data there and monitor the data pipelines originating there for potential problems.
In short, a data observability solution helps address the data problems of today’s complex data pipelines ensuring data quality and reliability are maintained. As such, a business can be assured that the results, the outcomes, the derived insight of any application that uses its data are valid and trusted.
A suitably selected solution should help data teams transition from reactive and passive modes of operations to more proactive methods. Such a solution should help predict, prevent, and resolve data quality and reliability issues before they impact performance and costs.
To learn more about data observability, visit Acceldata.io.