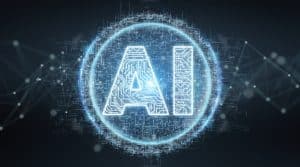
The Institute will bring together the brightest minds in the field to help address a wide spectrum of relevant AI use cases.
Deloitte has launched an institute to advance the adoption of artificial intelligence (AI) at a time when many organizations are struggling with such projects.
The Deloitte AI Institute will make best practices created by industry leaders, academics, and entrepreneurs more widely available as part of an effort to better identify use cases where AI is likely to have the most profound business impact, says Beena Ammanath, executive director of Deloitte AI Institute.
See also: Is Your AI Model Still Accurate After the Coronavirus Pandemic?
Participants will also be invited to discuss how best to navigate the complexities of AI ethics, adds Ammanath.
AI has become a major challenge to apply because of a host of issues ranging from the quality of the data available and the amount of infrastructure required to train AI models to determining how to continuously update AI models as business conditions change and more relevant data becomes available.
That latter requirement is driving the rise of machine learning operations, otherwise known as MLOps. IT teams once an AI model is built need to find a way to first actually embed an AI model into a specific application. Over time, that AI model will need to be retrained as more data becomes available or even replaced by a more accurate AI model.
The accuracy of AI models, of course, depends on the quality of the data employed to train them. Many AI projects never make it into production applications simply because the data employed to train them turned out to be unreliable. As a result, more organizations are making data quality, and associated management processes, the foundation upon which future AI projects are based.
The concern is the need to incorporate AI within the context of a business process has never been more pressing. In the wake of the economic downturn brought on by the COVID-19 pandemic, more organizations are at least exploring ways AI might enable them to either cut costs by streamlining existing business processes or potentially increasing revenue by adding services that previously would not have been feasible to develop and deploy.
“There’s still a lot of experimenting going on,” says Ammanath. “It’s not like there is a fixed playbook yet.”
Ultimately, the primary AI goal is to not replace humans as much as it is to augment them, adds Ammanath. It is not likely AI will be able to automate an end to end process without any guidance from a human any time soon, says Ammanath, However, she cautions they still need to be careful in terms of how they apply AI at scale because many things can go wrong.
As varying degrees of AI are incorporated into a wide variety of consumer applications, expectations concerning the level of AI capabilities that should be embedded within an application are on the rise. The challenge is finding a way to balance those expectations against the current state of the art, the size of the available budget, internal expertise, and the overall quality of the available data. In fact, when all those issues are considered it may very well explain why AI applications are not quite as pervasively deployed by now as everyone might have once initially anticipated.