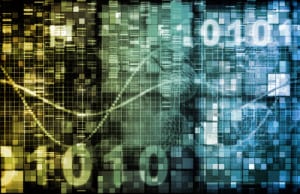
Streaming analytics is shining the light on better data understanding in today’s enterprise. Add in the cloud’s flexibility, and new applications arise.
Today’s generation of cognitive and analytical solutions packs a powerful punch, thanks to their ability to deliver insights as they are requested by decision makers or systems. Many of today’s game-changing solutions provide in-depth insights – ranging from understanding customer intentions, to health care measures, to systems performance – at the moment they are needed, which is often in real time. Cognitive and analytics systems can dive deep to draw and deliver inferences on behaviors and patterns.
But today’s cognitive and analytical engines systems don’t operate in a vacuum. They require data, and lots of it, to fuel their engines, with much of it fed on a real-time basis. That’s why behind every successful cognitive system or analytical engine is a streaming analytics engine – providing real-time data that is tapped and delivered from any and all selected sources.
The success of the various forms of cognitive computing – from artificial intelligence to machine learning to deep learning – rests on the ability to access data that provides a continuous flow of knowledge by which programs and algorithms can adapt and renew.
A new generation of streaming analytics solutions is making such capabilities possible, and with availability in the cloud, is accessible to any existing business environment. Such solutions are critical to cognitive and analytical computing for the following reasons:
- Streaming analytics is the enabler behind many powerful analytics and artificial intelligence applications and services.
- The value of streaming analytics is seen within very powerful and compelling business cases, such as leveraging the Internet of Things to transform business models from product producers to 24×7 service organizations; delivering quality healthcare decisions and predicting customer behavior.
- Cloud is making streaming analytics available to businesses – regardless of size and budget – on a widespread basis that is affordable and accessible.
What is Streaming Analytics?
Streaming analytics is the engine behind the engine – providing the continuous flow of data “fuel” that powers today’s generation of cognitive and analytical computing engines. Forrester Research defines streaming analytics as “software that provides analytical operators to orchestrate data flow, calculate analytics, and detect patterns on event data from multiple, disparate live data sources to allow developers to build applications that sense, think, and act in real time.” (Source: The Forrester Wave: Streaming Analytics, Q3 2017, Mike Gualtieri, September 7, 2017.)
While traditional analytics focuses on “data at rest,” streaming analytics focuses on data in motion. In the past, analytics and business intelligence solutions operated in batch mode, meaning data may be processed on a regular fixed schedule, often every 24 hours. Needless to say, much can happen or change within that time period. Streaming analytics processes “data in motion” and are triggered by selected events, such as customer transactions or system events.
Streaming analytics solutions identify what Forrester refers to as “perishable insights” — insights that enterprises must act on “within a given time frame or else the opportunity to change the course of business outcomes will pass.” Such capability also offers “continuous integration, orchestration of business processes, and embedded execution of code.”
In streaming analytics, there is a continuous query function that is running against data streaming in from a variety of sources, from applications to sensors. Streaming analytics also helps manage the cleansing and transformation of this data, which is especially critical for IoT-based data. The timing of data refreshes – whether in seconds or hours – can be adapted to the criticality of the requirement. Streaming analytics also supports other data management requirements, including aggregating to filtering, data joining and pattern matching.
Business Cases for Streaming Analytics
Streaming analytics is applicable anywhere there is a business problem that needs analysis. Prior to streaming analytics, events would take place across organizations – such as breakdowns in logistics – and would go unnoticed for hours, if not days and weeks, resulting in great costs to the business. Streaming analytics plays a key role in enabling enterprises and applications to react to events as they happen – or even before they happen.
Streaming analytics has a direct impact on business growth in the following ways:
- Business transformation: Streaming analytics paves the way to evolve into a digital, data-driven business. The ability to deliver data-driven insights, tied to key performance indicators, provides a foundation that the entire enterprise can rally around.
- Enhanced awareness and decision making: Streaming analytics provides decision-makers with insights from many data sources.
- Real-time/near-real-time responsiveness: Streaming analytics provides insights on events of material importance to the business as they occur.
- Greater operational efficiency: Streaming analytics enable managers and administrators to view and predict potential bottlenecks within their systems.
- Internet of Things management: As organizations increasingly rely on sensors, systems, and devices beyond the edges of the enterprise, streaming analytics helps capture and make sense of incoming data.
- Preventative and prescriptive insights: Streaming analytics help automatically take active measures to prevent potential issues, but also provide an intelligent review of counter-measures.
Streaming Analytics and the Cloud
Previously, a capability such as streaming analytics required a robust IT infrastructure, and implementing the technology may have been difficult to cost-justify for many organizations just beginning their analytics or cognitive computing journeys. Now, however, streaming analytics capabilities are available as cloud services – a game-changer for the technology.
The cloud provides to businesses seeking to leverage the power of streaming analytics:
- Streaming for all: Cloud-based services make streaming analytics available and affordable to businesses of all sizes and industries, providing economical and highly scalable capabilities.
- Streaming capacity: In today’s enterprise environment, there are countless points of data that may be beneficial to monitor – coming from internal enterprise systems, as well as networks or cloud environments. Many organizations do not have the capacity to build and support on-premises systems that can handle such data. However, it’s available through the cloud.
Case Study: Verizon Ensures Customer Understanding
Verizon, a leading telecommunications provider for almost 115 million people, was concerned about its ability to handle customer calls and turned to cognitive computing to enhance the customer experience at a large scale. Providing a range of services from wireless to broadband, mobile video, and Internet of Things networks, Verizon has a broad spectrum of customer support requirements, says Damodaram Bashyam, vice president of IT for Verizon. “We need to really understand the customer better every step of the way.”
The company built a real-time customer experience engine, based on IBM Streams, that analyzes speech in real time and integrates it with omnichannel insight to help customer service agents respond faster to customer needs. IBM Watson Explorer is a key component of this effort, providing the knowledge base to respond to issues. The goal was to help customers solve their problems the first time they call about the problem. Verizon wanted to raise first-call resolution rates by empowering its customer service teams with new insight.
“The data sources have been evolving over a period of time and we have collected a lot of structured and unstructured data through several sources of our customer interactions,” said Bashyam. “The customer can choose the channel through which they would like to interact with us, whether it’s digital, customer service center, or our stores. We have to make sure we follow the customer throughout the lifecycle.”
Verizon’s cognitive platform, supported by streaming analytics, enables the telecommunications giant to develop new applications in weeks and roll them out to millions of customers – helping to improve customer service and boost first-call resolution rates. “To provide the personalized experience, you need to understand the customer journey, customer intent, and gather the insights of the customer,” says Bashyam. “That means your system should be intelligent and continuously learning and getting smarter by understanding the customer interactions and correlating the interactions.”
Verizon’s combination of streaming analytics and cognitive processing “provides us these capabilities of artificial intelligence and machine learning to predict the customer intent by mining unstructured data,” he says. “To leverage all the data is going to be very critical as we continue this journey.”
Case Study: Medtronic’s Monitoring Boosts Patient Health
Medtronic, a diabetic health monitoring service, employs stream analytics to deliver insights through a cognitive mobile app that provides real-time actionable glucose insights and predictions to enable more informed decision-making by patients around oral medication, insulin management, diet, and exercise. To date, Medtronic has collected 125 million patient days of anonymous data from insulin pumps and glucose monitors, supported by IBM Streams. The anonymous data is then sent to IBM Watson Health for insights. The result of this effort is a mobile app called Sugar.IQ.
With Watson, a cognitive mobile personal assistant app that aims to provide real-time actionable glucose insights and predictions for individuals with diabetes helps to make daily diabetes management easier. “Before we started the development of Sugar.IQ with Watson, we correlated anonymized data from users’ insulin pumps and glucose monitors with their history of hypoglycemic episodes,” says Amit Singh, IT director of digital platforms at Medtronic. The model had an 85 to 89 percent accuracy rate for predicting hypoglycemic episodes, but Medtronic needed to refine the predictive model further.
“We needed a way to generalize and personalize the model further, and process data at near-real-time speed and scale,” Singh says. “Our insulin pumps send us data every 24 hours, and wearable continuous glucose monitors send data every five minutes, so we’re expecting to have millions of data points to analyze.”
Medtronic’s streaming analytics capability, delivered through IBM Streams, analyzes as it flows in from the devices, “using predictive models to assess each person’s current situation and the risk of their glucose levels falling outside safe thresholds,” Singh explains.
Case Study: Cerner’s Streams Keep the Lights On
Cerner, a provider of health information technology solutions, needed the ability to rapidly identify and address IT performance issues, and develop predictive models to warn of potential future problems. Providing uninterrupted uptime to healthcare applications is critical in healthcare environments.
To monitor and maintain peak performance at all times, Cerner employed stream analytics to rapidly identify and address IT performance issues, and develop predictive models to warn of potential future problems. By boosting IT performance, the company is delivering an improved user experience that helps clinicians gain instant insight into their patients, and make smarter treatment decisions.
Streaming analytics played a role in enabling the development of this predictive capability. One of the benefits has been an improved turnaround time on the code used in the company’s production environment, Adam Splitter, knowledge architect within the technology improvement department at Cerner, explains. “So what might have taken us 18-24 months in the past has all been eradicated now.” The solution also employs timing metrics “that tell us how the solutions perform, and what is going on, so we have about one and a half billion records that come back each and every day for that particular data set,” Splitter says. The company employs a data scientist to develop models for clinicians’ workflows, he says.
Summary and Recommendations
Every organization that seeks to provide higher levels of service and implements real-time decision making will benefit from streaming analytics capabilities. The following steps will help you on this journey.
Tie streaming analytics implementations directly to business needs. Streaming analytics aren’t applicable to every situation, as there are many events that will have little or no material impact on the business. It’s up to the business to determine what events and resulting insights have the most value to the business.
Promote involvement from across the enterprise. Just as data sources will come from across and beyond the enterprise, there needs to be assurance that data is not only available, but of the highest quality and accuracy as possible. This requires working closely with data providers, as well data consumers.
Start small, achieve demonstrable wins. Don’t overwhelm the analytics system with an enterprise worth of data. Select pilot projects and build from there.
Select a cloud service that will support scalable, cost-effective deployment. As the streaming analytics and cognitive solution domain grows, there will be a need to continuously expand capacity.