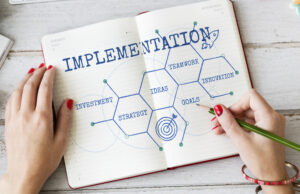
Businesses need an AI strategy that fosters a culture of innovation and collaboration, requiring multiple teams to break silos across data, processes, and workflows.
The buzz surrounding Generative AI (GenAI) was mirrored at every iteration of the AI trajectory. However, translating the hype into real-world impact is another story. A 2024 IBM report found that 40% of organizations experimenting with AI remain stuck in the sandbox, while IDC estimates that 20% of AI initiatives fail to deliver business outcomes. This is because the pace at which AI advancements have accelerated is yet to be matched by the pace at which enterprises can adapt and adopt the technology.
Despite this, investments in AI will continue to outpace IT investments by as much as five times over the next three years. As per a BCG survey, 54% of enterprise leaders expect AI to deliver cost savings in 2024, with half of them expecting cost savings of more than 10% through productivity gains.
For AI to deliver on these expectations, enterprises need to address the following opportunity gaps in their approach to AI:
Develop an AI vision: Enterprises often look at AI for what it can do rather than what they want AI to do for them. For instance, the initial euphoria surrounding GenAI was about how it could create code, text, images, or even music like humans could. It took time for executives to realize that it can speed up productivity and unlock efficiencies that open critical bandwidth for value-adding work. This lack of AI vision leads to misaligned investments, which is why 76% of enterprises barely break even with their investments in AI.
How: Addressing the lack of strategic alignment is key to successful AI transformation. Begin with a clear understanding of strategic priorities and business outcomes that can be accelerated with AI. A view of long-term business goals helps identify high-impact leverage points or use cases that maximize the business value delivered through AI.
Map business impact: Since advancements in AI come up faster than enterprises can strategize, most AI use cases remain point-focused. Enterprises get on the AI bandwagon without diving deep into a use case’s investments, impact, and business outcomes. For instance, it took a year for enterprises to realize GenAI can lead to significant time and cost compression if scaled across a business process end to end, compounding on the productivity gains it delivers when applied to a part of a workload.
How: A tailored prioritization framework can help enterprises evaluate an AI use case for desirability, viability, feasibility, predictability, and responsibility. Given the high upfront investments in technology, infrastructure, and skills, identifying the use case will help visualize the estimated return on value.
Foster internal coordination/collaboration: Without an enterprise-level AI strategy, different business units dedicate respective budgets to run pilots that cut horizontally across lines of business, leading to varying maturity levels and duplication of efforts. For instance, different teams invest in developing and deploying AI for knowledge assist systems that are fungible and find cross-functional applicability. As business units continue to operate in silos, the overarching AI initiatives hit the same hurdles, such as creating a data pipeline, training the underlying model, and enforcing governance.
How: ‘Platformizing’ horizontal AI capabilities that cater to the functional needs of business domains by developing AI accelerators, such as plug-and-play knowledge assist tools, can help foster collaboration and coordination in scaling AI pilots. These horizontal AI capabilities can work with a common set of retrieval augmented generation (RAG) components that power AI use cases, such as knowledge assist, digital asset management, and low-level virtual agents.
Access niche skill ecosystem: Operationalizing AI requires robust data engineering, model training, and post-deployment governance strategies to ensure accuracy and high performance. This needs guardrails to ensure AI ethics, explainability, and transparency. The requisite niche skills are hard to acquire or harder to retain.
How: Investing in Centers of Excellence (CoEs) with AI experts can help streamline model training, testing, and deployment. Enterprises can also form strategic partnerships with vendors across the AI stack – infrastructure, cloud hyperscalers, and data platforms – to get access to the right AI components and resources to orchestrate fit-for-purpose AI capabilities.
See also: Use Case Milestones on the Path from Data Foundations to GenAI Applications
Strategizing a Long-term AI Roadmap
To avoid the trough of disillusionment, enterprises need to define an AI vision that mirrors their long-term business strategy that answers the following questions:
1) The Why: Enterprises should pin top strategic priorities they want AI to co-drive. Breaking these down into a set of sub-goals will bring the required level of visibility into investments, stakeholder coordination, and impact areas. For instance, if an enterprise’s top goal is to maximize FTE utilization, then improving productivity is a sub-goal. AI use cases that lead to productivity gains either by assisting tasks or automating low-level decision-making are ideal, and a multi-level approach to implement such productivity improvements is the way forward.
2) The What: Enterprises should assess the current state of AI experiences for an objective baseline to help them decide ‘what’ incremental investments they need to make. For instance, for productivity gains, assessing the current SDLC processes can help identify bottlenecks and low-level tasks. Task-based analysis can help zero in on the variables that lead to loss of efficiency. Furthermore, with systems thinking, enterprises can understand the leverage points for maximum impact, which leads to potential AI use cases to optimize efficiencies.
3) The How: Before the identified AI use cases can take off, enterprises need to categorize them along a spectrum based on the complexity of AI-led decision-making. This spectrum spans from Assistance to Augmentation, Automation, and Autonomy. Establishing standardized AI accelerator stacks can be helpful in ensuring widespread applicability across various business processes. These stacks should encompass AI models, RAG architecture, data processing/governance, responsible AI governance, and infrastructure design. An AI CoE can oversee the development of horizontal AI accelerator stacks and establish reusable AI platform capabilities, paving the way for sustainable and scalable AI deployment.
Enterprise AI is an Evolving Journey
An AI strategy helps internal stakeholders work out a clear vision, formulate a roadmap and execution strategy, and create and adhere to a governance framework that aligns AI initiatives with business objectives. This approach also fosters a culture of innovation and collaboration, requiring multiple teams to break silos across data, processes, and workflows.
AI systems are self-learning and require a constant flow of data and in-production feedback to maintain acceptable levels of accuracy. This necessitates a cohesive data strategy encompassing a robust data ecosystem architecture, comprising data processing and governance capabilities.
This is a heavy lift for enterprises that do not have data and AI as core competencies. Partnering with a strategic player that brings the right synergies with vendor ecosystems, a rich understanding of data structures, and an established history of operationalizing ML models can help define an AI vision and get first-time-right AI that delivers on business outcomes.