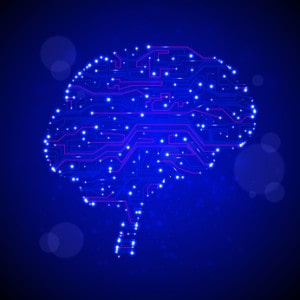
Machine learning involves intelligent systems that automatically learn, predict and act by using Big Data analysis. Here, RT Insights contributor Dr. Paulo Marques explains why machine learning is best to perform real-time data analysis of any kind, across all channels of your business.
I’m currently the CTO of Feedzai, Inc., a data science company that uses real-time, machine-based learning to analyze Big Data to make commerce safe. But several years ago, I worked as a Technical Consultant with the European Space Agency (ESA), whose mission is to “shape the development of Europe’s space capability and ensure that investment in space continues to deliver benefits to the citizens of Europe and the world.” I was excited to apply my skills to explore the next frontier.
After a number of years, I started looking at ways I could apply my expertise in other areas. It didn’t take long as another frontier in desperate need of tackling is not in outer space. In fact, it’s right here on Earth. There is a whole new world—no, scratch that, a whole new universe—of data waiting to be explored. And if you don’t tackle it in real-time, then you might as well not pursue it at all. Here’s why.
In the last two years, 90 percent of today’s existing data was created. The Internet of Things (IoT)—aka, more connected devices—will attract five billion smartphone users in the next four years. Nearly 80 percent of personal consumption expenditures (PCEs) will be conducted in electronic form by the year 2017. The amount of data for each person on Earth, currently estimated at 1TB of data per person, is doubling each year.
Data Explosion in Payments Industry
One industry seeing this data explosion is payments. Data is coming from everywhere; there are more payment channels, more mobile shoppers and new retail business models that output data every second. The growth can be very rapid and it’s often hard for the institution—whether it’s a bank, credit card issuer or retailer—to keep up with the sheer volume of data in order to pull out important insights.
As the data mass grows, it’s important to ensure that 1) the business can scale, 2) the customer experience is preserved, and 3) fraud is prevented. How can companies glean insights in real-time in order to keep on track? Humans can create algorithms to analyze data and manage risk but it’s become impossible to keep up with the sheer volume and rapid creation of data.
What Machine Learning Is
Enter machine learning. It’s the best bet in performing analysis of any kind in real-time, across all channels of the business. Machine learning is intelligent systems that automatically learn, predict and act using data. Many companies use legacy software solutions but, with our changing world of real-time data, there isn’t room for single-channel solutions as data easily crosses channels.
In the case of the financial industry, fraud happens quickly. In fact, it is happening as you read this, on all channels. Until recently, the industry used old, rules-only systems which can’t act or react quickly enough against machine-based attacks and smart fraudsters. And in-house scoring doesn’t work as a stand-alone solution because the growing volume, variety, velocity and veracity of usable data is unmanageable for most companies. In summary, data patterns change quickly. Human-generated rules cannot evolve fast enough.
In the image above, notice how fraud is long-tailed. The sum of corner cases is more damaging than major cases. For human rules, the one-size-fits-all rule generates many manual review cases. In other cases, true fraud does not trigger any rules at all. With machine models, probabilistic models fit the nuances of each case.
Why Machine Learning?
Data is created and used in real-time. Machine learning models allow you to mark cases that teach the system to improve. For instance, in the world of payments, past purchase data makes the system learn. Only with machine learning can you find hidden patterns in data. And machine learning models scale unlike any other solution. So, what do you need for all this to happen?
Machine Learning Needs Infrastructure
You must have the appropriate amount of data storage and memory, with the computer processors and bandwidth to handle this. It’s likely you will need to move beyond a standard Java virtual machine (JVM) as it’s almost impossible to have ultra-low latencies (i.e., in the range of five to 10 milliseconds) which customers demand, at least in the financial industry. Look for enterprise applications and workloads that require any combination of large memory, high transaction rates, low latency, consistent response times and high-sustained throughput.
Machine Learning Needs Historical Data
While this may be obvious, you need data sets of historical information since it’s from these that you learn and adapt. These are segmented for specific operations such as supervised learning, over/under sampling, clustering and classification. Leverage historical, third-party and proprietary data, and select model features that infer the expected behavior. Benchmark the results against a baseline and compare performance against the current baseline to understand performance improvements. Lastly, deploy new data configurations from sandboxing to production environments.
Machine Learning Needs Skilled Personnel
You need a solid team of data scientists, engineers and analysts to execute on the job. They’ll need to know how to use enterprise software packages such as Apache Cassandra and Azul as well as understand machine learning enough to be able to use data for real-time transactions and operations.
In all of this, remember to keep it human. Disciplines such as search engine optimization (SEO) have competitors abiding by rules but financial disciplines have hostile adversaries purposely breaking the rules. So, be sure to 1) focus, 2) ensure you have the right tools to perform in real-time, 3) improve the customer experience by scaling as needed, and 4) rapidly iterate.