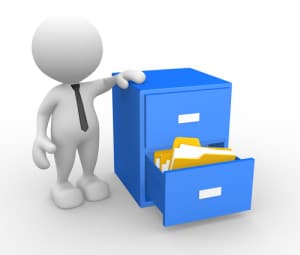
MemSQL says real-time analytics are prompting a new big data battleground over the future of the data warehouse and its role in IT infrastructure.
Going into 2018 there’s a major transition underway in terms of how IT organizations are approaching analytics. Instead of always employing a batch-oriented approach, there’s now a much bigger requirement to process analytics in real time to enable various types of digital business initiatives.
But even as that transition occurs the need for legacy analytics applications are not going to go away. If anything, the results generated by real-time analytics applications will be used to better inform legacy analytics applications. The issue that creates for IT organizations is a need to find a common database platform that can support both types of analytics applications.
See also: White House calls 5G a national security priority
Gary Orenstein, chief marketing officer for MemSQL, contends MemSQL is a unique position to address both those requirements because its database and data warehouse platform can process data both in-memory and using disk-based storage. That approach makes it simpler and cost-effective to compare historical data to real-time data as that news streams into the database, says Orenstein.
Orenstein says there is an increasing number of use cases where organizations can no longer wait to analyze data at the end of the day. They need to be able to process analytics several times a day to make better-informed decisions faster. But that daily analytics only have real business value when there is enough historical context to make the best decision possible. Because of that requirement, Orenstein says the number of organizations looking to unify real-time and traditional batch-oriented analytics will rise considerably in 2018.
“Organizations want to be able to store data as it streams into the database,” says Orenstein.
SQL is Still Data Warehouse King But….
The primary language IT organizations will employ to interrogate that data will remain SQL. But the amount of data that the underlying relational database is expected to be able to process now ranges into the realm of multiple petabytes. MemSQL addresses the challenges associated with storing that amount of data by making use of algorithms and a vector engine to compress data at a 10:1 ratio, regardless of whether that data is stored in SQL, JSON or a geospatial format.
Not too long relational databases were deemed to be not scalable enough to address such Big Data applications. There were major investments in Hadoop-based platforms that could process massive amounts of batch-oriented data, just not at the speed of a relational database. But in the last several years vendors such as MemSQL have created database engines that can now meet the scalability requirement of well over 80 percent of Big Data analytics applications. At the same time, databases such as MemSQL can now ingest millions of events per second. Those twin capabilities mean most IT organizations no longer necessarily need to support multiple data processing engines.
It’s too early say what database engines will win the next round of data warehousing wars. There’s no doubt that open source platforms based on Hadoop have carried much of the day in the past two years. But database administrators that have a lot of time and energy invested in relational database technologies still have much sway and, for many of them, the revenge of the relational database in the enterprise is an event that can’t come too soon.