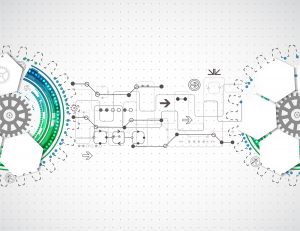
Companies that want deeper, richer insights from their data scientists must leverage their teams’ expertise with strategic tools designed to automate tasks that create bottlenecks.
Companies in the data-driven era are focused on two key goals – scalability and adaptability. One of the biggest questions now is how to achieve those two goals without overloading their already taxed data science teams. A recent survey from Ascend.io confirms that most data scientists are at or over capacity, which doesn’t bode well for companies looking to leverage the real power of data. Let’s take a look at what the results of the survey mean and how companies might shift their efforts to support data science teams and their own data initiatives.
Watch Video Now: Digital Complexity and How to Address ItBacklogs rule the game for data scientists
The vast majority of more than 400 survey respondents noted that their organizations intended to increase data pipelines. Even more significant, companies were adding new pipelines beyond what data science teams could manage. However, further questioning indicates that teams feel their infrastructure and tools were able to handle the scale.
If tools can handle the increased workload, that makes scale a people issue. The challenge in scale isn’t because of data volume; instead, output and developer productivity are the current concerns. Data products and tools are outpacing teams required to manage and deploy them.
Each aspect of a data science team identifies its own component as responsible for the backlog, i.e., data scientists feel like data science is the issue while data engineering identifies the engineering component as behind. To fix the lag in all areas, three main solutions came up.
See Also: Continuous Intelligence Insights
Replatform
The survey found that 30% of the respondents planned to retire slow and outdated legacy systems, switching over to tools better able to handle their pipelines. In reality, this could be a challenging project to take on because it could mean losing previous data collateral or taking on expensive and time-consuming training.
New products
53% of respondents indicated that purchasing new tools to add to existing solutions would be the way to go. Adding tools has several advantages:
- Less training
- Retaining legacy collateral
- Customization of products
Selecting products as the only solution can sometimes lead to shiny object syndrome. Instead of addressing the root issue, organizations put band-aids on the backlog and end up with more products than teams can handle.
Automation to aid data scientists
Another group (53%) points to automation as an expected solution. Although the survey doesn’t specify, at least a few most likely plan to automate along with either re-platforming or new products. This is the key.
Download Infographic Now: The 5 intelligence gaps curbing your climb to digital successWithout some sort of automation, teams could continue to chase the illusion of a perfect new tool, one that relieves pressure and facilitates their workflow. Without an element of automation, these tools will always fall short.
Why automation matters to data scientists
Survey respondents may be frustrated with backlogs, but this fact remains: data science team members cannot keep up with new pipelines and products. If companies want to leverage data and increase volume and scale, automation is the only thing that will make it possible.
Automation handles mundane tasks
For many data scientists, handling the mundane tasks of scrubbing and maintenance prevents working on high-level projects designed to produce greater insights. Companies can’t always hire more team members to handle the analysis, but automation could relieve that burden.
Automation ensures that data comes ready to analyze and that results from that analysis are of higher quality. Data scientists can focus on the task of visualization and interpretation, moving the needle towards true data-driven decision-making.
Automation allows scale
Automation helps accelerate outcomes. Period. It helps make teams more agile by handling a greater volume of data prep and requiring fewer interactions before data is ready. Teams are able to take on more projects and significantly reduce the time to ROI.
It also provides a foundation for working in agile. Even small data teams or single data scientists can create models and tweak the pipeline to account for different needs or changes in direction.
Automation helps teams weather disruption.
With the right automation, data science teams can work from anywhere. The proliferation of secure cloud-based initiatives removes the on-premises requirement, allowing teams to work in the office, at home, or a combination of both.
For disruptive events such as a pandemic, this frees businesses to make data-driven decisions despite any outward disturbances. As a result, companies don’t worry about achieving an unsustainable scale.
Considerations before moving to automate
Automation isn’t a magic bullet. Companies must understand that algorithms are only as good as the humans running them. Without some kind of human oversight, teams run the risk of losing track or control of insights, as well as the ability to explain results.
Automation cannot replace a good data science team. Instead, augmented intelligence – or human/machine partnership – helps ensure that data science teams have the freedom created by automating mundane tasks but continue to provide the expertise needed for trustworthy models.
Another issue highlighted by the survey itself is that experts still aren’t sure about no-code products, even if they facilitate the type of automation required for scale. Only 4% of respondents preferred a no-code interface, so companies should make concessions before adopting this type of software.
If data science teams have the option to use their preferred programming language in addition to no code choices, the willingness to use no-code products jumps to 73%. Data scientists may feel overwhelmed, but they still want some control over their environments. With a component like this, teams could have solutions for more complex business needs.
Reducing overwhelm is a critical piece of the puzzle
Companies that want deeper, richer insights from data must leverage their teams’ expertise with strategic tools designed to automate tasks that create bottlenecks. The results of Ascend.io’s survey suggest that no matter what role in data science, each position will require help from smarter, more efficient tools.
Engineering, analysis, and architecture can all use automation to troubleshoot and maintain the infrastructure and data that data scientists need for their models. And for that component, data scientists can rely on these automation tools to support their innovative efforts to draw up new models and build better visualizations – all in the name of business value.
Watch Video Now: Digital Complexity and How to Address It