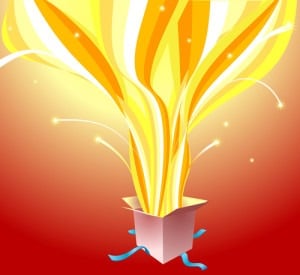
Even top data scientists admit they don’t know where the AI journey we are on as a society will end up. But we shouldn’t discard the benefits along the way.
There has been no shortage of discussion — and consternation — about the “black box” of artificial intelligence, in which decisions and output are delivered without any transparency or understanding of why the system made the decisions it made. Even the most highly knowledgeable data scientists admit they don’t quite understand how AI may arrive at its decisions. However, not everybody feels this is a bad thing.
Cathy O’Neil — mathematician, data scientist, and author of “Weapons of Math Destruction: How Big Data Increases Inequality and Threatens Democracy” — has been warning about the dangers of opaque AI decisions on businesses, individual and society in general, stating that such decisions may be loaded with biases, or just plain wrong. And nobody is double-checking results, she adds.
See also: Big data needs AI, and AI needs big data
Amid heightening concern about the lack of transparency in AI-driven decisions, Bank of America and Harvard University have partnered to create the Council on the Responsible Use of Artificial Intelligence, intended to together business, government and societal leaders to discuss the legal, moral, and policy implications of AI and machine learning, and propose more responsible AI platforms.
One of the goals of the council is to pave the way to opening up the AI black boxes that are increasingly driving decisions in today’s organizations, said Cathy Bessant, COO and CTO for BofA and an active sponsor of the program, in a recent Forbes interview with Peter High. “When the creators and sellers are dominating the discussion with their models and data sources around which they have built intellectual property, by definition, they are building a black box that as the user, we may or may not have transparent insight into,” she says. Opening up AI’s black box “is an important part of understanding the intended and unintended consequences of the models that we are using to drive learning. In financial services, we went through these decades ago in credit scoring.”
Still, we may want to think twice about tearing open the black box, some observers caution. David Weinberger, senior researcher at Harvard University’s Berkman Center for Internet and Society and co-author of “The Cluetrain Manifesto,” says opening up AI’s black box means making trade-offs we may not want to make. It’s human nature to seek logical explanations for every event, he says.
The promise of a safer autonomous transportation future?
Speaking at the recent Data Summit in Boston, he cites the example of a driverless car in a future scenario that veers off course and kills its occupant. “She was in an AV [autonomous vehicle], she should have been protected. It’s somebody’s fault. You want an explanation, but there is none. There are thousands of cars, they will be networked, they will be communicating. It’s a gigantic mess. Each has their own black box, these systems may be getting inputs from other black boxes, like weather systems. You simply may not be able to get an explanation.”
In many cases already — from healthcare to production — AI systems are producing results that can’t be explained, but are more accurate than human judgment, because they’re basing output on analytics run against massive amounts of data. In Weinberger’s traffic fatality example, opening up cars’ AI systems — making them more human explicable — may mean reducing their efficiency. “Let’s say we go from 40,000 to 5,000 fatalities per year. We could insist that all AVs be fully explicable, but that increases fatalities to 12,000 a year. “Are we willing to sacrifice 5,000 people a year for that?”
Such are the trade-offs that will be necessary as more businesses build their products and processes around AI. “We make AI stupider if we want to make it more explicable,” Weinberger continues, “which means more traffic deaths, perhaps less accuracy in diagnostics, etc. — all the benefits that we’re looking for.”
Rather than trying to make black boxes more transparent, Weinberger urges a greater emphasis on AI systems design at the time they are built or manufactured. This involves collaboratively working to set goals — for example, in the case of auto manufacturers, prioritizing safety over vehicle performance and so on. It’s not an easy task to set such priorities, he adds. “Coming up with such lists may be problematic. People may want to set different goals.”