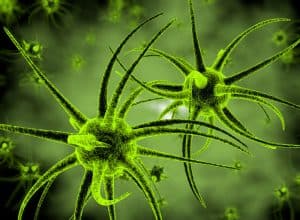
Scientists around the world are turning to sophisticated analytics to predict the spread of the coronavirus and other contagious diseases.
Businesses routinely use continuous intelligence (CI) and artificial intelligence (AI) to model dynamic systems, make predictions, automate decisions, and take actions in timeframes to achieve success. Just as businesses are turning to CI and AI to make predictions and take informed actions, world health organizations and government health agencies seek to use the same technologies to understand better and model the spread of the coronavirus and other contagious diseases.
See also: Predictive Analytics Algorithm Detects Sepsis In Pediatric Patients
With the current outbreak, a variety of efforts are using analytics to study social media streams and world health organization reports and databases. Some are using machine learning to scour medical publications, healthcare websites, and numerous other sources looking for information related to the symptoms of the disease, reported infections, infection rates, and other factors.
The results are mixed. Predicting the rate of growth and where the disease will appear next are incredibly complex challenges. Past efforts have fallen short. However, researchers are aware of the shortcomings that limited success in the past. Hopefully, such awareness will lead to better models and more accurate predictions in the future.
Building on the Past
In 2008, Google Flu Trends tried to estimate influenza activity for more than 25 countries by aggregating Google Search queries about flu symptoms. Unfortunately, it often over-estimated flu activity. Google shut down the service, but the technology behind the service is currently being used by some global health organizations.
The shortcomings are not limited to predictions with contagious diseases. Other efforts to predict outcomes in scientific fields have had similarly mix results.
Take the bomb cyclone, a special type of rare winter storm that explodes (hence the name) into major storms in a very short time period. For years, forecasting models failed to predict them. The consequences were often brutal. The storms produced massive snowfalls, hurricane force-winds (in some cases), and people had virtually no warning beforehand.
People were left stranded, without food or lights. They did not have the time to stock up on batteries, water, or other supplies in advance. Cities, towns, and utilities did not have staff on hand or resources in place to clear streets, remove downed trees, or restore electricity.
One problem with these storms was a lack of data. The storms rapidly developed while in the Atlantic. Basic data (temperatures, wind speeds, and pressures) was sparse compared to data collected over land. Meteorologists addressed the problem by collecting vast amounts of data at sea.
Once seeing the development with better data, they noted that the National Meteorological Center forecasting models used at the time did not predict the pressure drop and intensification of these storms. The models were then updated to incorporate the newly discovered physics.
Applicable Lessons for Contagious Disease Predictions and Business
Any predictive model needs data –lots and data that is relevant. And models must match real-world conditions.
Past efforts to model the spread of contagious diseases may have made false assumptions about the data they relied on? Does the fact that many people in one geographic region search for the name of an emerging contagious disease mean the disease is present and growing? Perhaps, perhaps not. The danger is relying on coincidences and not linking cause to effect.
Did past and current efforts have all the data they needed? One issue with forecasting the spread of a disease is that models might not have accurate data. The issue is especially relevant at the onset of new diseases. It is quite easy to blur flu-like symptoms in patients. Doctors may not know the symptoms of a disease at its onset, or they may make inaccurate diagnoses.
Are the models based on the right science? At the early stage of investigating a newly found disease, even basic information, like how a disease spreads, is unknown. Is it airborne? Does it spread via exposure to blood or other bodily fluids? What’s the incubation period? Such mechanisms need to be nailed down before predictions can be made.
Are traditional models that predict the spread of a disease valid? One lesson that many companies learn when using AI and machine learning is that data changes rapidly, and models must constantly be retrained. Predicting the spread of a rapidly propagating contagious disease must take these issues into account.
All these factors apply when businesses use CI and AI to make predictions. Successful efforts in business need the same things as successful efforts to model the spread of contagious diseases. Specifically, businesses need large volumes of meaningful data. They need to understand the processes they are modeling. They need to train, test, and continuously retrain the models using the most up to date, complete, and accurate data.