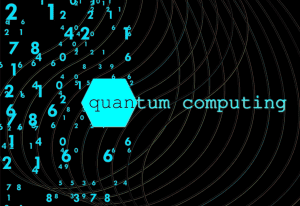
Quantum computing is being considered for application areas including supply chain logistics and challenges related to climate change. It is also expected to solve existing problems faster while reducing computing costs.
Quantum computing is moving out of the lab into the data center. A majority of high performance computing (HPC) data center operators in a recent survey. 76%, say they plan to use quantum computing by 2023, and 71% plan to move to on-premises quantum computing by 2026.
The survey of 110 data center executives, conducted by Atos and IQM last year, confirms that “it is becoming increasingly difficult for users to get the optimal performance out of high-performance computing while ensuring both security and resilience.” The expected benefits of the introduction of quantum computers include supply chain logistics (45%), challenges related to climate change (45%) and solving existing problems faster (38%) – while at the same time reducing computing costs (42%).
See also: Quantum Computing Acceleration of AI in Pharma on the Rise
Cloud is a key part of this HPC architecture, mixing standard elements with custom-developed infrastructure components — 46% of respondents cite hybrid and cloud deployments as especially important to deploying supercomputing power via cloud.
Supercomputing was once reserved for only the wealthiest or most well-funded operations. Think of the mighty Cray supercomputer employed a couple decades back to process large-scale scientific applications, or the massively parallel Linux-based systems employed in more recent times at national laboratories to conduct weather simulations or explore nuclear fusion. (Cray was acquired by Hewlett-Packard Enterprise in 2019.)
Download Now: Building Real-time Location Applications on Massive DatasetsSupercomputers are only getting more powerful — and will multiply in capacity and speed even more so with quantum computing as it becomes increasingly viable. At the same time, thanks to cloud, supercomputing power has become available to everyone who needs it, paying only for the time it is used. This has enormous implications for innovation as we know it.
See also: Quantum Computing Will Help Keep the Lights On
This means major disruptions in the way innovations are delivered. “The cloud has made the processing power of the world’s most powerful computers accessible to a wider range of companies than ever before,” Joris Poort writes in Harvard Business Review. “Instead of having to architect, engineer, and build a supercomputer, companies can now rent hours on the cloud, making it possible bring tremendous computational power to bear on R&D.”
Innovation-driven approaches include “evaluating new designs through cloud-based simulation instead of physical prototyping, simulating a product’s interaction with real-world scenarios when physically prototyping is impractical, and predicting the performance of a full range of potential designs.” Cloud-service-based supercomputing “also opens up the possibilities for new products and services, which would have previously been impossible or impractical.”
For example. experts at General Atomics (GA), working together with the San Diego Supercomputer Center (SDSC) and Drizti Inc., announced they recently developed a prototype for running fusion reactor simulations in the cloud. The idea may significantly simplify the modeling process for fusion reactor designs and help bring fusion energy closer. Use of the cloud-based supercomputing service promises to substantially reduce the challenges with fusion plasma simulations, which in the past required leading supercomputers.
“Exceptional hardware capabilities are not enough for scientists to make good use of cloud high-performance computing resources,” says Igor Sfiligoi, a HPC software developer at SDSC. “Scientists at on-premises facilities are typically working with a strictly prescribed and very optimized setup. This is very different from the wide-open and flexible cloud computing environment. That meant we still had to develop a convenient interface.”
When it comes to large simulations – “like the computational fluid dynamics to simulate a wind tunnel – processing the millions of data points needs the power of a distributed system and the software that schedules these workloads is designed for HPC systems,” according to Mary Branscombe, writing in ZDNet. “If you want to simulate 500 million data points and you want to do that 7,000 or 8,000 times to look at a variety of different conditions, that’s going to generate about half a petabyte of data; even if a cloud virtual machine (VM) could cope with that amount of data, the compute time would take millions of hours so you need to distribute it – and the tools to do that efficiently need something that looks like a supercomputer, even if it lives in a cloud data center.”
Cloud services such as Microsoft Azure offers Cray XC and CS supercomputers, Branscombe observes. “HPC users on Azure run computational fluid dynamics, weather forecasting, geoscience simulation, machine learning, financial risk analysis, modelling for silicon chip design (a popular enough workload that Azure has FX-series VMs with an architecture specifically for electronic design automation), medical research, genomics, biomedical simulations and physics simulations, as well as workloads like rendering.”
In another example of cloud-based supercomputing at work, Satcom provider Gogo, which provides next-generation in-flight connectivity solutions for commercial airliners, needed to mount its next-generation of satellite antennas and radomes on a wide variety of aircraft, without sacrificing the aircrafts’ aerodynamics, performance, or safety. Radomes—the weatherproof enclosures that protect the radio antennas and are effectively transparent to radio waves—are installed on the outside of the aircraft, which means their shape and placement can affect the aerodynamic loads on the structure of the plane. The team designing the enclosures had to perform “simulations to test designs that accounted for all the changing variables. With each one requiring 20 to 40 million data points to model the airplane, not to mention a tight schedule for the client, the team needed the computing power and resources of high-performance computers. With in-house computers, each simulation took between eight to 12 hours to complete, and without the infrastructure necessary to run simulations in parallel.