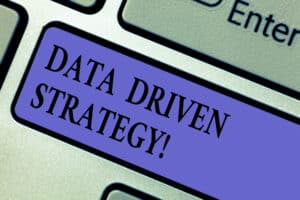
The more digital a company becomes, the more data it accumulates, providing a foundation for informed decision-making, strategic planning, and sustained success in the ever-evolving business landscape.
Though many companies are already investing in AI transformation (89% of large companies, in fact) many of them have failed to see a corresponding revenue lift. According to data from McKinsey, only 31% of companies executing AI initiatives globally have seen the expected revenue lift and only 25% of the expected cost savings from the effort.
So what’s the holdup? For many, it comes back to the quality of their data. AI tools are only as good as the data that fuels them, and so to see results from their investment, many companies must take a good, hard look at their data infrastructure layer.
There’s a lot of disparity among these global companies, with many of them at various stages of their digital transformation. From those still relying on traditional tools and processes to those fully immersed in the cloud, the diversity in digitalization levels can be overwhelming and makes recommendations difficult.
That disparity might account for the relatively low impact of digital transformation initiatives to date. But make no mistake, digital transformation can be wildly impactful, and many steps can be taken to elevate business operations for companies regardless of where they sit on the innovation curve. But a fruitful effort starts with a strong foundation.
1. Beginner: Data Centralization vs. Data Democratization
The average person often takes digitalization for granted, underestimating the transformative power of even simple digital tools. Consider the time saved by a basic CTRL + F search function, a tool so ingrained in most people’s digital lives that its absence would be conspicuous.
Many companies, especially those established before the internet era, had to make deliberate choices about embracing digitalization, and though these foundational decisions might seem as basic as a CTRL + F search, they’re no less impactful.
Sysco, which was founded in 1969, is one such company. Sysco operates in the food service industry, which has traditionally lagged behind other industries when it comes to embracing technology. Recently, it’s begun shifting from a data-centralization model to a data-democratization model.
Data centralization allows data accessibility but is usually managed by a dedicated team. This means organizations can be more certain about their data quality but at the expense of their agility and flexibility. It also creates bottlenecks as more employees make requests to access or analyze data.
Data democratization, on the other hand, empowers users to access, analyze, and share data without relying on a central authority. More people can run more queries and innovate in more areas, but this does mean that companies risk exposing themselves to problems regarding data quality and security. Too much data-democratization creates chaos and it can enable the development of too many internal resources that it’s hard to find the truth.
In Sysco’s case, they sought to imitate the customer experience—where all data is available on demand—and replicate it internally, developing an internal resource for all employee queries. Employees, much like customers, are accustomed to having data on demand in their personal lives, so bringing that to the workplace was a natural transition.
The key to avoiding the chaos of data democratization is to ensure there’s a base level of data literacy and clear governance in the organization. Even simple workflow permissions that manage user access and limit decision-making to users with specific credentials can ensure that accessibility does not put the organization at risk. With the right guardrails, organizations benefit from centralized data resources and mitigate the risk of data misuse. For organizations early in their digital transformation, data literacy, and governance should be a consideration as they explore data centralization vs. democratization.
2. Intermediate: Navigating Nice-to-Have vs. Need-to-Have
Digitalization is not a black-and-white transition. Many companies have tried their hand at transitioning some manual functions to digital without fully migrating their operations. At this intermediate stage, businesses can distinguish between “nice-to-have” and “need-to-have” digital elements.
For instance, moving inventory from an online marketplace to a DTC (direct-to-consumer) site is an exciting proposition when it comes to building longer-term relationships with customers that rely on more data. However, online marketplaces like Amazon command a vast majority of online shoppers. Owning customer data might be a longer-term goal and is nice to have, but prioritizing DTC while ignoring marketplaces might cause organizations to miss out on a lot of sales in the meantime.
In many cases, it makes sense to ramp on a marketplace while taking a “crawl, walk, run” approach to DTC. This may be time-consuming initially but will prove to be a worthwhile investment in the long run as you grow incremental sales while slowly gaining customer data.
As you consider navigating nice-to-haves vs. need-to-haves at this stage, evaluate your digital initiatives based on their contribution to efficiency, customer satisfaction, and overall business growth.
3. Advanced: Getting the Most from What You Have
For companies confident in their digital capabilities, there is always room for improvement. At the advanced stage, the focus shifts from gaining new sources of data to getting the most from existing data sources, often using AI.
Learning from existing data becomes a valuable investment for the most digitized organizations. Implementing advanced technologies, such as AI-powered customer service chatbots, enables businesses to understand user motivations, intentions, and reasoning. Predictive analytics can be employed to anticipate malfunctions, while automation of mundane processes further enhances operational efficiency.
For example, at Lyft and similar ride-hailing platforms, a consistent problem has been trying to understand customer motivations. Lyft knew people were riding with them but didn’t know why. So, they began using data from customer service conversations to unlock the answer to that question, helping them improve marketing, availability, and customer service.
The possibilities are vast, from machine learning algorithms that optimize supply chain logistics to data-driven marketing strategies that enhance customer targeting. The advanced stage is characterized by a proactive approach to leveraging data, turning it into a powerful tool for innovation and growth.
Defining the Starting Place
Employee education is crucial at every step of the digitalization journey. As companies advance in their digital capabilities, it is essential to ensure that employees possess the necessary skills to navigate and utilize the technological landscape.
While the process may seem daunting, digitalization is a worthwhile investment if it’s prioritized in the right areas at the right time. The more digital a company becomes, the more data it accumulates, providing a foundation for informed decision-making, strategic planning, and sustained success in the ever-evolving business landscape.
Embracing digitalization is not just a trend; it is a fundamental strategy for navigating the complex terrain of the modern business world.